Foundation models are fundamental to the influence of ai on the economy and society. Transparency is crucial for accountability, competence and understanding, particularly when it comes to the data used in these models. Governments are enacting regulations such as the EU ai Law and the US ai Foundation Transparency Model Law to improve transparency. The Foundation Model Transparency Index (FMTI) introduced in 2023 assesses transparency among 10 major developers (e.g. OpenAI, Google, Meta) using 100 indicators. The initial FMTI v1.0 revealed significant opacity, with an average score of 37 out of 100, but also observed variability in disclosures.
The Core Model Transparency Index (FMTI), introduced in October 2023, conceptualizes transparency through a hierarchical taxonomy aligned with the core model supply chain. This taxonomy includes three top-level domains: upstream resources, the model itself, and its downstream use, and encompasses 23 subdomains and 100 binary transparency indicators. FMTI v1.0 revealed widespread opacity among 10 companies evaluated, with maximum scores reaching only 54 out of 100. Developers of open models performed better than closed ones. The index aims to track changes over time, encouraging transparency through public and stakeholder pressure, as demonstrated by historical indices such as the HDI and the 2018 Digital Rights Ranking Index.
Researchers from Stanford University, MIT, and Princeton University presented the follow-up study (of FMTI v1.0) of FMTI v1.1 to evaluate the evolution of transparency in the base models over six months, maintaining the 100 original transparency indicators. Developers were asked to report the information themselves, improving completeness, clarity, and scalability. Fourteen developers participated, revealing new information for 16.6 indicators on average.
FMTI v1.1 involves four steps: indicator selection, developer selection, information collection, and scoring. The 100 FMTI v1.0 indicators span three domains: upstream resources, the model itself, and downstream usage. Fourteen developers, including eight from version 1.0, submitted transparency reports for their flagship models. Information collection has moved from public searches to direct submissions from developers, ensuring completeness and clarity. Scoring involved two researchers independently evaluating each developer's disclosures, followed by an iterative refutation process. This approach improved transparency by allowing developers to provide additional information and reducing researcher effort.
The researchers summarize the implementation of FMTI v1.1 as follows:
- Developer Request: Leaders of 19 companies that develop foundation models were contacted, requesting transparency reporting.
- Developer Reports: Fourteen developers designated their flagship core models and submitted transparency reports addressing each of the 100 transparency indicators for their models.
- Initial Scoring: Developer reports were reviewed to ensure consistent scoring standards across all developers for each indicator.
- Developer Response: The scored reports were returned to the developers, who then questioned specific scores and potentially provided additional information. The finalized transparency reports, validated by the developers, were made public.
For the evaluation, 14 developers submitted transparency reports on 100 indicators for their flagship models. Initial scores varied significantly: 11 of 14 developers scored below 65, indicating room for improvement. The mean and median scores were 57.93 and 57, respectively, with a standard deviation of 13.98. The highest-scoring developer scores points for 85 of the 100 indicators, while the lowest-scoring developer scores 33. Developers revealed important new information, improving transparency scores by an average of 14.2 points. Transparency was highest in downstream domains and lowest in upstream domains, and open developers generally outperformed closed developers. Transparency improved across all domains compared to the previous iteration.
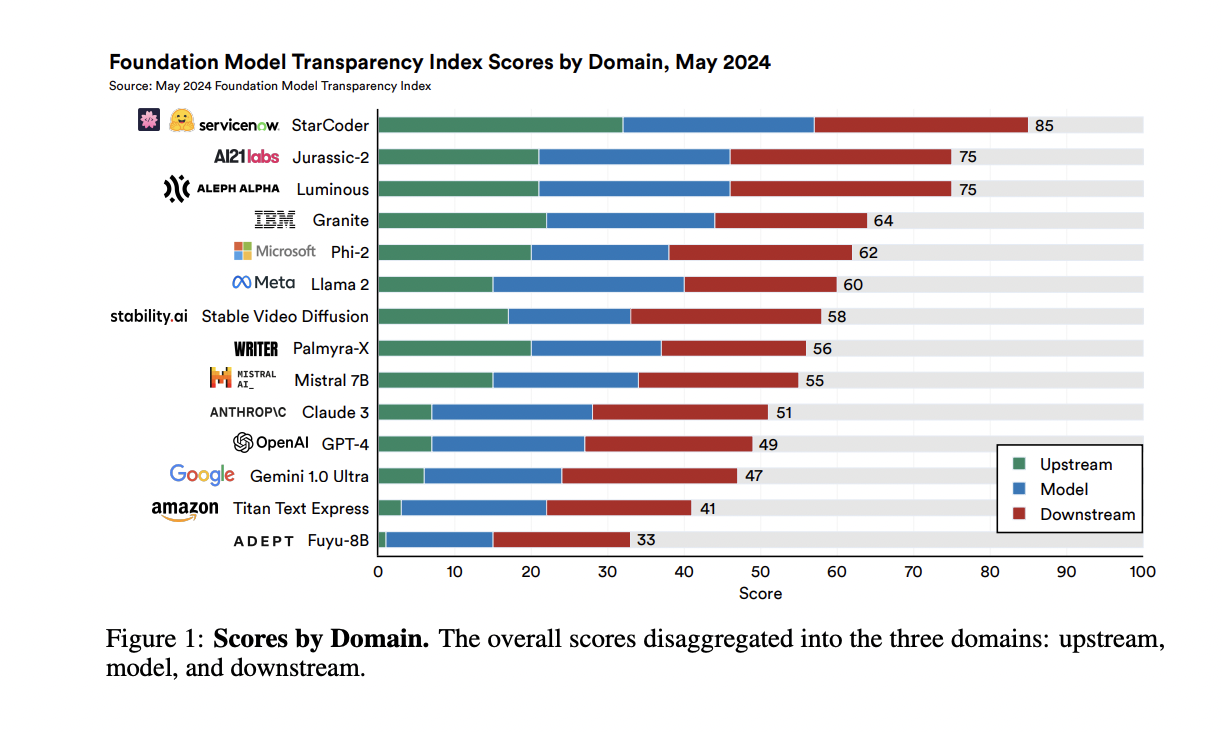
The social impact of foundation models is growing, attracting the attention of various stakeholders. The Foundation Model Transparency Index shows that transparency in this ecosystem needs to improve, although there have been positive changes since October 2023. By analyzing developer disclosures, the Index helps stakeholders make informed decisions. By establishing transparency reports for core models, the Index provides a valuable resource for developers, researchers, and journalists to improve collective understanding.
Review the Paper, GitHuband Project. All credit for this research goes to the researchers of this project. Also, don't forget to follow us on twitter.com/Marktechpost”>twitter. Join our Telegram channel, Discord channeland LinkedIn Grabove.
If you like our work, you will love our Newsletter..
Don't forget to join our 42k+ ML SubReddit
Asjad is an internal consultant at Marktechpost. He is pursuing B.tech in Mechanical Engineering at Indian Institute of technology, Kharagpur. Asjad is a machine learning and deep learning enthusiast who is always researching applications of machine learning in healthcare.
<script async src="//platform.twitter.com/widgets.js” charset=”utf-8″>