FineWeb2 Significantly advances multilingual pre-training datasets, covering over 1000 languages with high-quality data. The dataset uses approximately 8 terabytes of compressed text data and contains almost 3 trillion words, obtained from 96 CommonCrawl snapshots between 2013 and 2024. Processed using the datatrove library, FineWeb2 demonstrates superior performance compared to established datasets such as CC-100, mC4, CulturaX, and HPLT in nine different languages. Ablation and evaluation settings are present in this github repository.
Huggingface community researchers introduced FineWeb-C, a community-driven collaborative project that extends FineWeb2 to create high-quality educational content annotations in hundreds of languages. The project allows community members to rate the educational value of web content and identify problematic elements through the Argilla platform. Languages that reach 1000 annotations qualify for inclusion in the data set. This annotation process has a dual purpose: to identify high-quality educational content and to improve the development of LLMs in all languages.
318 members of the Hugging Face community have submitted 32,863 entries, contributing to the development of high-quality LLMs in underrepresented languages. FineWeb-Edu is a dataset built from the original FineWeb dataset and employs an educational quality classifier trained on LLama3-70B-Instruct annotations to identify and retain most educational content. This approach has proven successful, outperforming FineWeb on popular benchmarks while reducing the volume of data needed to train effective LLMs. The project aims to extend the capabilities of FineWeb-Edu to all world languages by collecting community annotations to train language-specific educational quality classifiers.
The project prioritizes human-generated annotations over LLM-based ones, particularly for low-resource languages where LLM performance cannot be reliably validated. This community-driven approach parallels Wikipedia's collaborative model, which emphasizes open access and the democratization of ai technology. The contributors join a broader movement to break down language barriers in ai development, as commercial companies often focus on profitable languages. The open nature of the dataset allows anyone to create ai systems tailored to the specific needs of the community, while making it easy to learn about effective approaches in different languages.
FineWeb-Edu uses multiple annotations per page for some languages, allowing flexible calculation of annotator agreement. Quality control measures include plans to increase annotation overlap in heavily annotated languages. The data contains a boolean column 'problematic_content_label_present' to identify pages with problematic content indicators, which often result from incorrect language detection. Users can filter content based on individual problematic labels or annotator agreement via the 'problematic_content_label_agreement' column. The dataset operates under the ODC-By v1.0 license and the CommonCrawl Terms of Use.
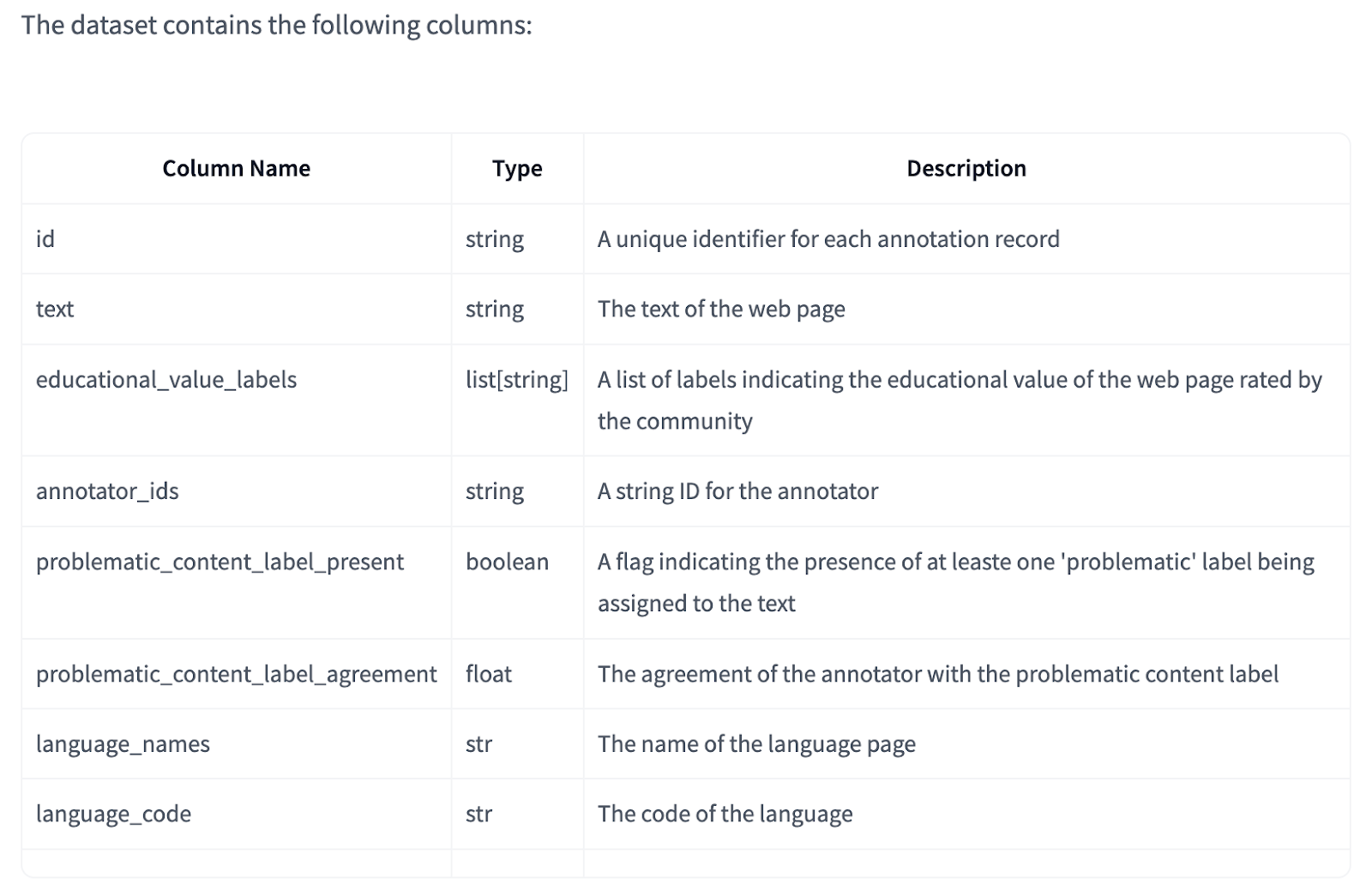
In conclusion, FineWeb2's community-driven extension, FineWeb-C, has collected 32,863 annotations from 318 contributors, focusing on educational content tagging. The project demonstrates superior performance compared to existing datasets with less training data through FineWeb-Edu's specialized educational content classifier. Unlike commercial approaches, this open source initiative prioritizes human annotations over LLM-based ones, particularly for low-resource languages. The dataset features robust quality control measures, including multiple layers of annotations and filtering of problematic content, while operating under the ODC-By v1.0 license.
Verify he details. All credit for this research goes to the researchers of this project. Also, don't forget to follow us on <a target="_blank" href="https://twitter.com/Marktechpost”>twitter and join our Telegram channel and LinkedIn Grabove. Don't forget to join our SubReddit over 60,000 ml.
Trending: LG ai Research launches EXAONE 3.5 – three frontier-level bilingual open-source ai models that deliver unmatched instruction following and broad context understanding for global leadership in generative ai excellence….
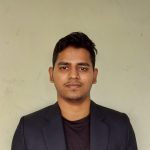
Sajjad Ansari is a final year student of IIT Kharagpur. As a technology enthusiast, he delves into the practical applications of ai with a focus on understanding the impact of ai technologies and their real-world implications. Its goal is to articulate complex ai concepts in a clear and accessible way.
<script async src="//platform.twitter.com/widgets.js” charset=”utf-8″>