If you’re new to the terminology, you might be wondering what cinemagraphs are, but I can assure you that you’ve probably come across them already. Cinemagraphs are visually captivating illustrations where specific elements repeat continuous motion while the rest of the scene remains motionless. They are not images, but we cannot categorize them as videos. They provide a unique way to display dynamic scenes while capturing a particular moment.
Over time, cinemagraphs have gained popularity as short videos and animated GIFs on social media platforms and photo-sharing websites. They are also commonly found in online newspapers, business websites, and virtual meetings. However, creating a cinemagraph is a very challenging task as it involves capturing videos or images with a camera and using semi-automatic techniques to generate continuously looping videos. This process often requires significant user input, including capturing appropriate images, stabilizing video frames, selecting animated and static regions, and specifying directions of motion.
In the study proposed in this article, a new research problem, namely the synthesis of text-based cinemagraphs, is explored to significantly reduce the reliance on data capture and laborious manual efforts. The method presented in this work captures movement effects such as “falling water” and “flowing river” (illustrated in the introductory figure), which are difficult to express through still photography and existing text-to-image techniques. Crucially, this approach broadens the range of styles and compositions that can be achieved in cinemagraphs, allowing content creators to specify various artistic styles and describe imaginative visual elements. The method shown in this research has the ability to generate realistic cinemagraphs and scenes that are creative or otherworldly.
Current methods face significant challenges in addressing this new task. One approach is to use a text-to-image model to generate an artistic image and then animate it. However, existing animation methods that operate on individual images have difficulty generating meaningful movements for artistic inputs, mainly because they are trained on real video data sets. Building a large-scale dataset of looping artistic videos is impractical due to the complexity of producing individual cinemagraphs and the various artistic styles involved.
Alternatively, text-based video models can be used to generate videos directly. However, these methods often introduce noticeable temporary flicker artifacts in static regions and fail to produce the desired semi-periodic motions.
An algorithm called Text2Cinemagraph based on twin image synthesis is proposed to bridge the gap between artistic images and animation models designed for real videos. The overview of this technique is presented in the following image.
The method generates two images from a text message provided by the user, one artistic and one realistic, sharing the same semantic layout. The artistic image represents the desired look and feel of the end result, while the realistic image serves as input that is more easily processed by current motion prediction models. Once the motion of the realistic image is predicted, this information can be transferred to its artistic counterpart, allowing for the synthesis of the final cinemagraph.
Although the realistic image is not displayed as the final result, it plays a crucial role as an intermediary layer that resembles the semantic design of the artistic image while being compatible with existing models. To improve motion prediction, additional information from text cues and semantic segmentation of the realistic image is taken advantage of.
The results are reported below.
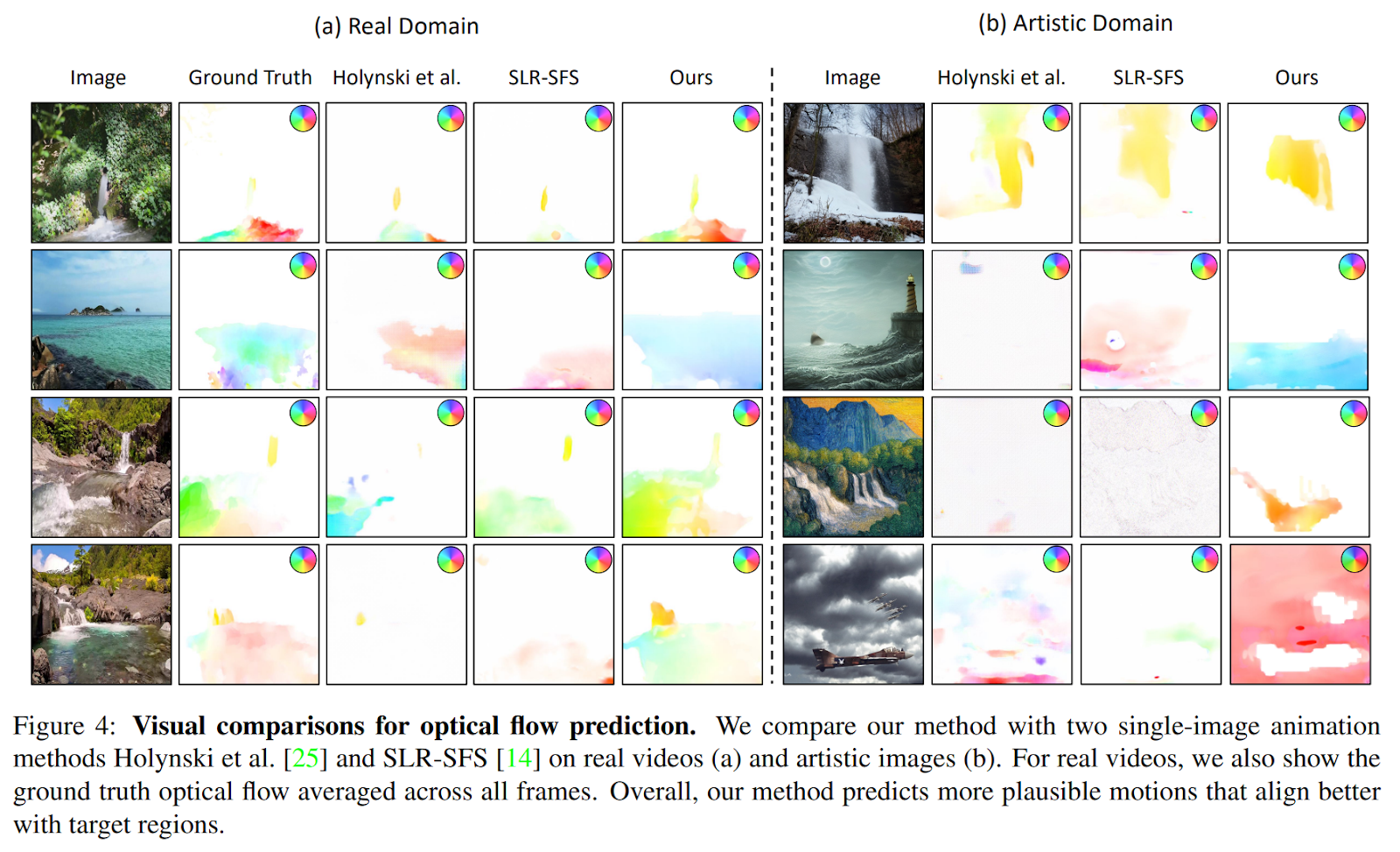
This was the brief for Text2Cinemagraph, a novel AI technique for automating the generation of realistic cinemagraphs. If you are interested and would like to learn more about this job, you can find out more by clicking on the links below.
review the Paper, Github and Project. All credit for this research goes to the researchers of this project. Also, don’t forget to join our 26k+ ML SubReddit, discord channeland electronic newsletterwhere we share the latest AI research news, exciting AI projects, and more.
🚀 Check out over 800 AI tools at AI Tools Club
Daniele Lorenzi received his M.Sc. in ICT for Internet and Multimedia Engineering in 2021 from the University of Padua, Italy. He is a Ph.D. candidate at the Institute of Information Technology (ITEC) at the Alpen-Adria-Universität (AAU) Klagenfurt. He currently works at the Christian Doppler ATHENA Laboratory and his research interests include adaptive video streaming, immersive media, machine learning and QoS / QoE evaluation.