Understanding protein sequences and their functions has always been a challenging aspect of protein research. Proteins, often described as the building blocks of life, are made up of long, complex sequences that determine their functions in biological systems. Despite advances in computational biology, making sense of these sequences in a meaningful way remains a difficult task. Traditional methods for analyzing proteins are time-consuming and expensive. Even with recent technological advances, researchers struggle to map the great diversity of protein structures and their functional variations found in nature. This gap between available data and practical knowledge remains a major obstacle to developing new therapies, bioengineering solutions and addressing broader challenges in health and environmental sciences. The need for a comprehensive tool to analyze proteins at an unprecedented scale has never been more urgent.
EvolutionaryScale has launched ESM Cambriana new language model trained on protein sequences at a scale that captures the diversity of life on Earth. ESM Cambrian represents a major step forward in bioinformatics, using machine learning techniques to better understand protein structures and functions. The model has been trained on millions of protein sequences, covering an immense range of biodiversity, to uncover the underlying patterns and relationships in proteins. Just as large language models have transformed our understanding of human language, ESM Cambrian focuses on protein sequences that are fundamental to biological processes. It aims to be a versatile model capable of predicting structure, function and facilitating new discoveries between different species and families of proteins.
Technical details
ESM Cambrian's technical foundation is as impressive as its objectives. EvolutionaryScale has released different versions of the model, including the ESM C 300M and ESM C 600M, with weights openly available to the research community. These models strike a balance between scale and practicality, allowing scientists to make powerful predictions without the infrastructure challenges that come with very large models. The largest variant, ESM C 6B, is available on EvolutionaryScale Forge for academic research and on AWS Sagemaker for commercial use, with plans to launch on NVIDIA BioNemo soon. These platforms facilitate access to this tool for users in both academic and industrial environments.
The model, based on the transformative architecture, uses self-attention mechanisms to identify complex relationships within protein sequences, making it well suited for tasks such as predicting protein folding or discovering new functions. One of the main benefits of ESM Cambrian is its ability to generalize knowledge about different proteins, which could accelerate the discovery of new drugs and synthetic biology applications.
ESM Cambrian was trained in two stages to achieve its high performance. In Stage 1, for the first million training steps, the model used a context length of 512, and metagenomic data accounted for 64% of the training data set. In Stage 2, the model underwent an additional 500,000 training steps, during which the context duration was increased to 2048 and the proportion of metagenomic data was reduced to 37.5%. This staged approach allowed the model to efficiently learn from a diverse set of protein sequences, improving its ability to generalize across different proteins.
First results and insights
Early tests of ESM Cambrian have shown promising results. The model's ability to predict the structure and function of protein sequences is comparable to traditional experimental methods and offers significant savings in both time and cost. The evaluations were carried out using the methodology of Rao et al. measure unsupervised learning of protein tertiary structure via contact maps. Logistic regression was used to identify contacts and the precision of top L contacts (P@L) was assessed for proteins of length L, with a sequence separation of 6 or more residues. The average P@L was calculated on a set of temporarily held protein structures (with a deadline of May 1, 2023) for scaling laws and on the CASP15 benchmark for performance evaluation. Initial insights suggest that ESM Cambrian performs well in generalizing to understudied protein families, helping researchers uncover hidden relationships in sequences that would otherwise be difficult to analyze. Its predictive accuracy also opens new possibilities in enzyme engineering, where understanding the subtle nuances of protein activity is crucial.
The availability of ESM Cambrian on platforms such as AWS Sagemaker and NVIDIA BioNemo will make it easier for business users to integrate machine learning tools into their existing workflows. EvolutionaryScale's decision to launch open weights for ESM C 300M and ESM C 600M reflects a commitment to open science, fostering collaboration to better understand the foundations of life on Earth.
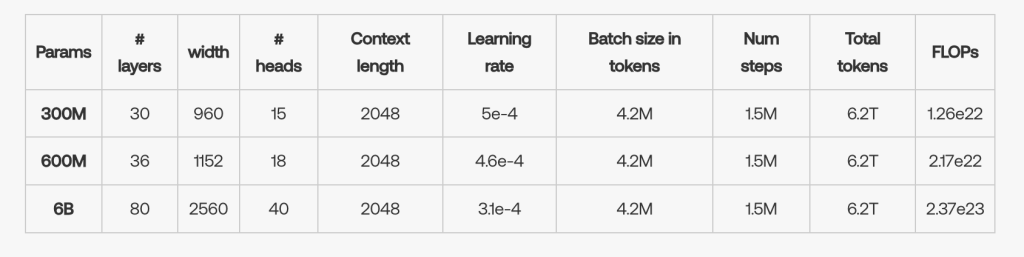
Conclusion
The release of ESM Cambrian by EvolutionaryScale marks an important milestone in computational biology and protein science. By providing a model that can analyze protein sequences at a scale that captures the diversity of Earth's biodiversity, EvolutionaryScale has demonstrated the potential of applying ai in biological research and has opened numerous opportunities to accelerate discovery and innovation. ESM Cambrian will play a key role in protein engineering, drug discovery and gaining a deeper understanding of biological systems. As the scientific community begins to explore applications of this model, it is clear that the future of protein research is evolving, with tools like ESM Cambrian leading the way.
Verify he <a target="_blank" href="https://www.evolutionaryscale.ai/blog/esm-cambrian” target=”_blank” rel=”noreferrer noopener”>Details and GitHub page. All credit for this research goes to the researchers of this project. Also, don't forget to follow us on <a target="_blank" href="https://twitter.com/Marktechpost”>twitter and join our Telegram channel and LinkedIn Grabove. If you like our work, you will love our information sheet.. Don't forget to join our SubReddit over 60,000 ml.
(<a target="_blank" href="https://landing.deepset.ai/webinar-fast-track-your-llm-apps-deepset-haystack?utm_campaign=2412%20-%20webinar%20-%20Studio%20-%20Transform%20Your%20LLM%20Projects%20with%20deepset%20%26%20Haystack&utm_source=marktechpost&utm_medium=desktop-banner-ad” target=”_blank” rel=”noreferrer noopener”>Must attend webinar): 'Transform proofs of concept into production-ready ai applications and agents' (Promoted)
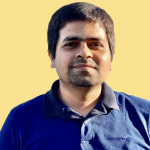
Asif Razzaq is the CEO of Marktechpost Media Inc.. As a visionary entrepreneur and engineer, Asif is committed to harnessing the potential of artificial intelligence for social good. Their most recent endeavor is the launch of an ai media platform, Marktechpost, which stands out for its in-depth coverage of machine learning and deep learning news that is technically sound and easily understandable to a wide audience. The platform has more than 2 million monthly visits, which illustrates its popularity among the public.
<script async src="//platform.twitter.com/widgets.js” charset=”utf-8″>