Machine Learning ML offers significant potential to accelerate the solution of partial differential equations (PDEs), a critical area in computational physics. The goal is to generate accurate PDE solutions faster than traditional numerical methods. While machine learning shows promise, concerns about reproducibility in machine learning-based science are increasing. Issues such as data leaks, weak baselines, and insufficient validation undermine performance claims in many fields, including medical ML. Despite these challenges, interest continues in using ML to improve or replace conventional PDE solvers, with potential benefits for optimization, inverse problems, and reduction of computational time in various applications.
Researchers at Princeton University reviewed the literature on machine learning and machine learning to solve fluid-related PDEs and found overly optimistic claims. Their analysis revealed that 79% of studies compared ML models with weak baselines, leading to exaggerated performance results. Furthermore, widespread reporting biases, including outcome and publication biases, further distorted the results by underreporting negative outcomes. Although ML-based PDE solvers such as physics-based neural networks (PINN) have shown potential, they often fail in speed, accuracy, and stability. The study concludes that the current scientific literature does not provide a reliable assessment of the success of ML in resolving PDEs.
Machine learning-based PDE solvers often compare their performance to standard numerical methods, but many comparisons suffer from weak baselines, leading to exaggerated claims. Two major obstacles include comparing methods with different levels of precision and using less efficient numerical methods as baselines. In a review of 82 articles on ML for PDE resolution, 79% compared weak baselines. Furthermore, reporting biases were prevalent: positive results were often highlighted while negative results were not reported or hidden. These biases contribute to an overly optimistic view of the effectiveness of ML-based PDE solvers.
The analysis employs a systematic review methodology to investigate how frequently the ML literature on PDE resolution compares its performance to weak baselines. The study focuses specifically on papers that use ML to derive approximate solutions for various fluid-related PDEs, including the Navier-Stokes and Burgers equations. Inclusion criteria emphasize the need for quantitative comparisons of speed or computational costs, while excluding a variety of non-fluid-related PDEs, qualitative comparisons without supporting evidence, and articles lacking relevant baselines. The search process involved compiling a comprehensive list of authors in the field and using Google Scholar to identify relevant publications from 2016, including 82 articles that met the defined criteria.
The study establishes essential conditions to ensure fair comparisons, such as comparing ML solvers with efficient numerical methods with equal accuracy or execution time. Recommendations are provided to improve the reliability of comparisons, including cautious interpretation of results from specialized machine learning algorithms versus general-purpose numerical libraries and justification of hardware choices used in evaluations. The review highlights in detail the need to evaluate baselines in ML applications for PDEs, pointing out the predominance of neural networks in the selected articles. Ultimately, the systematic review seeks to illuminate existing deficiencies in the current literature while encouraging future studies to adopt more rigorous comparative methodologies.
Weak baselines in machine learning for PDE resolution are often due to a lack of experience in the ML community, limited numerical analysis benchmarking, and insufficient awareness of the importance of strong baselines. . To mitigate reproducibility issues, it is recommended that ML studies compare results to standard numerical methods and other ML solvers. Researchers must also justify their choice of baselines and follow established rules to make fair comparisons. Additionally, addressing biases in reporting and fostering a culture of transparency and accountability will improve the reliability of ML research in PDE applications.
look at the Paper. All credit for this research goes to the researchers of this project. Also, don't forget to follow us on twitter.com/Marktechpost”>twitter and join our Telegram channel and LinkedIn Grabove. If you like our work, you will love our information sheet..
Don't forget to join our SubReddit over 50,000ml
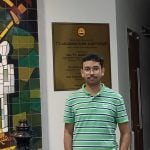
Sana Hassan, a consulting intern at Marktechpost and a dual degree student at IIT Madras, is passionate about applying technology and artificial intelligence to address real-world challenges. With a strong interest in solving practical problems, he brings a new perspective to the intersection of ai and real-life solutions.
<script async src="//platform.twitter.com/widgets.js” charset=”utf-8″>