Enzymes are essential molecular catalysts that facilitate biochemical processes vital to life. They play crucial roles in metabolism, industry and biotechnology. Despite their importance, there are important gaps in our knowledge about these catalysts. Of the approximately 190 million protein sequences cataloged in databases such as UniProt, less than 0.3% are expert-curated and less than 20% have experimental validation. Furthermore, 40% to 50% of known enzymatic reactions remain unlinked to specific enzymes, often called “orphan” reactions. These knowledge gaps hinder progress in synthetic biology and biotechnological innovation. Traditional computational tools, including EC classification and sequence similarity methods, frequently fall short, particularly when dealing with low sequence homology enzymes or reactions that do not align with established classifications. To overcome these limitations, new strategies that combine structural and functional knowledge are needed.
EnzymeCAGE: a new approach
A team of researchers from Shanghai Jiaotong University, Hong Kong University of Science and technology, Hainan University, Sun Yat-sen University, McGill University, Mila-Quebec ai Institute and MIT developed a new basic open source model for enzyme recovery and function. prediction called enzyme cage. This model is trained on a data set of approximately one million enzyme-reaction pairs and employs the Contrastive Language and Image Pretraining (CLIP) framework to annotate unseen enzymes and orphan reactions. EnzymeCAGE, an acronym for CAtalytic-aware GEometric Enhanced Enzyme Recovery Model, integrates structural learning with evolutionary insights to address the limitations of conventional methods. The model effectively links unannotated proteins to catalytic reactions and identifies enzymes for novel reactions. EnzymeCAGE is a robust tool for enzymology and synthetic biology that takes advantage of enzyme structures and reaction mechanisms. Its geometry-aware and reaction-guided modules enable precise insights into enzymatic catalysis, making it applicable to a wide range of species and metabolic contexts.
Technical features and benefits
EnzymeCAGE incorporates several advanced features to effectively model interactions between enzymes and reactions. At its core is the geometry-enhanced pocket attention module, which uses structural information such as residue distances and dihedral angles to identify catalytic sites. This improves both the accuracy and interpretability of your predictions. Additionally, the model employs a center-aware reaction interaction module that emphasizes reaction centers through weighted attention, capturing the dynamics of substrate-product transformations. EnzymeCAGE combines pocket-level local coding using Graph Neural Networks (GNN) with global enzyme-level features from the ESM2 protein language model. This holistic approach provides a comprehensive representation of catalytic potential. Furthermore, the model's compatibility with both experimental and predicted enzyme structures expands its applicability to tasks such as enzyme recovery, orphan reaction elimination, and pathway engineering.
Performance and knowledge
EnzymeCAGE has undergone rigorous testing demonstrating superior performance compared to existing methods. On the Loyal-1968 test set, which included unseen enzymes, the model achieved a 44% improvement in function prediction and a 73% increase in enzyme recovery accuracy relative to traditional approaches. It recorded a Top 1 success rate of 33.7% and a Top 10 success rate of over 63%, outperforming benchmarks such as BLASTp and Selenzyme. In orphan reaction elimination tasks, EnzymeCAGE consistently identified enzymes suitable for orphan reactions, achieving higher enrichment factors and ranking metrics across diverse test sets. Practical case studies further highlight its capabilities, including the precise reconstruction of the glutarate biosynthesis pathway, where it outperformed traditional methods in enzyme classification and selection. These results underscore the utility of EnzymeCAGE in addressing major challenges in enzyme function prediction and catalysis research.
Conclusion
EnzymeCAGE represents an important step forward in addressing long-standing challenges in enzyme research, particularly in function prediction and reaction annotation. By integrating geometric, structural, and functional knowledge, it provides accurate predictions for unseen enzyme functions, annotations for orphan reactions, and support for pathway engineering. The adaptability of the model and its tuning capabilities enhance its usefulness for specific enzyme families and industrial applications. EnzymeCAGE lays a solid foundation for future advances in biocatalysis, synthetic biology and metabolic engineering, offering new avenues to deepen our understanding of enzymatic processes and their potential for innovation.
Verify he Paper and GitHub page. All credit for this research goes to the researchers of this project. Also, don't forget to follow us on <a target="_blank" href="https://twitter.com/Marktechpost”>twitter and join our Telegram channel and LinkedIn Grabove. Don't forget to join our SubReddit over 60,000 ml.
Trending: LG ai Research launches EXAONE 3.5 – three frontier-level bilingual open-source ai models that deliver unmatched instruction following and broad context understanding for global leadership in generative ai excellence….
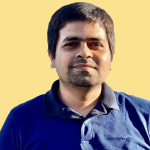
Asif Razzaq is the CEO of Marktechpost Media Inc.. As a visionary entrepreneur and engineer, Asif is committed to harnessing the potential of artificial intelligence for social good. Their most recent endeavor is the launch of an ai media platform, Marktechpost, which stands out for its in-depth coverage of machine learning and deep learning news that is technically sound and easily understandable to a wide audience. The platform has more than 2 million monthly visits, which illustrates its popularity among the public.
<script async src="//platform.twitter.com/widgets.js” charset=”utf-8″>