Integrating advanced predictive models into autonomous driving systems has become crucial to improve safety and efficiency. Camera-based video prediction emerges as a fundamental component, offering valuable real-world data. ai-generated content is currently a leading area of study within the domains of computer vision and artificial intelligence. However, generating photorealistic and coherent videos poses significant challenges due to limited memory and computation time. Furthermore, video prediction from a front-facing camera is critical for advanced driver assistance systems in autonomous vehicles.
Existing approaches include diffusion-based architectures that have become popular for generating images and videos, with improved performance on tasks such as image generation, editing, and translation. Other methods such as generative adversarial networks (GANs), flow-based models, autoregressive models, and variational autoencoders (VAEs) have also been used for video generation and prediction. Probabilistic diffusion denoising models (DDPMs) outperform traditional generation models in effectiveness. However, generating long videos remains computationally demanding. Although autoregressive models such as Phenaki address this problem, they often face challenges with unrealistic scene transitions and inconsistencies in longer sequences.
A team of researchers from Columbia University in New York has proposed the DriveGenVLM framework to generate driving videos and used vision language models (VLMs) to understand them. The framework uses a video generation approach based on diffusion denoised probabilistic models (DDPM) to predict real-world video sequences. A pre-trained model called efficient learning in context on egocentric videos (EILEV) is used to evaluate the suitability of the generated videos for VLMs. EILEV also provides narrations for these generated videos, which can improve traffic scene understanding, aid navigation, and enhance planning capabilities in autonomous driving.
The DriveGenVLM framework is validated using the open dataset Waymo, which provides diverse real-world driving scenarios across multiple cities. The dataset is split into 108 videos for training and split equally between the three cameras, and 30 videos for testing (10 per camera). This framework uses the Frechet Video Distance (FVD) metric to evaluate the quality of the generated videos, where FVD measures the similarity between the distributions of the generated videos and the real ones. This metric is valuable for temporal consistency and visual quality assessment, making it an effective tool for evaluating video synthesis models on tasks such as video generation and future frame prediction.
The results of the DriveGenVLM framework on the Waymo open dataset for three cameras reveal that the hierarchy-2 adaptive upsampling method outperforms other sampling schemes by producing the lowest FVD scores. Prediction videos are generated for each camera using this upsampling method, where each example is conditioned on the first 40 frames, with both ground-truth frames and predicted frames. Furthermore, training the flexible diffusion model on the Waymo dataset shows its ability to generate consistent and photorealistic videos. However, it still faces challenges in accurately interpreting complex real-world driving scenarios such as navigating traffic and pedestrians.
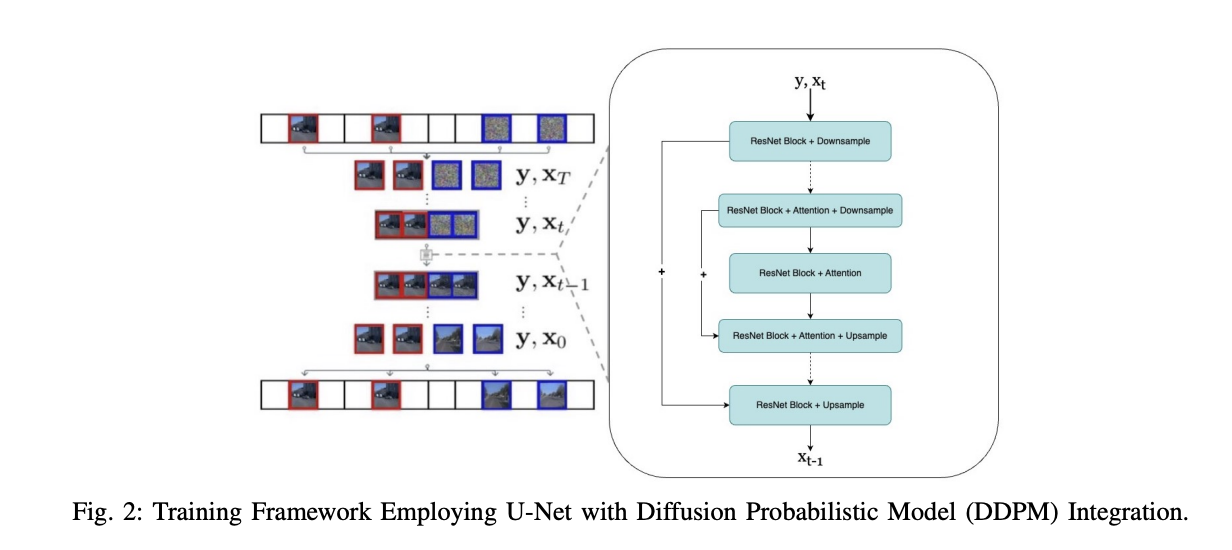
In conclusion, researchers from Columbia University have introduced the DriveGenVLM framework to generate driving videos. The DDPM trained on the Waymo dataset is proficient in generating consistent and realistic images from the front and side cameras. Furthermore, the pre-trained EILEV model is used to generate action narratives for the videos. The DriveGenVLM framework highlights the potential of integrating generative models and VLMs for autonomous driving tasks. In the future, the generated descriptions of driving scenarios can be used in large language models to provide driver assistance or support language model-based algorithms.
Take a look at the Paper. All credit for this research goes to the researchers of this project. Also, don't forget to follow us on twitter.com/Marktechpost”>twitter and LinkedInJoin our Telegram Channel.
If you like our work, you will love our fact sheet..
Don't forget to join our SubReddit of over 50,000 ml
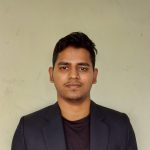
Sajjad Ansari is a final year student from IIT Kharagpur. As a technology enthusiast, he delves into practical applications of ai, focusing on understanding the impact of ai technologies and their real-world implications. He aims to articulate complex ai concepts in a clear and accessible manner.
<script async src="//platform.twitter.com/widgets.js” charset=”utf-8″>