Early attempts at 3D generation focused on single-view reconstruction using category-specific models. Recent advances use pre-trained image and video generators, in particular diffusion models, to enable open-domain generation. Fine-tuning of multi-view datasets improved results, but challenges persisted in generating complex compositions and interactions. Efforts to improve compositionality in image generation models faced difficulties in transferring techniques to 3D generation. Some methods extended distillation approaches to compositional 3D generation, optimizing individual objects and spatial relationships while adhering to physical constraints.
The synthesis of human-object interactions has advanced with methods such as InterFusion, which generates interactions based on textual cues. However, limitations in controlling the identities of humans and objects remain. Many approaches struggle to preserve the identity and structure of the human mesh during interaction generation. These challenges highlight the need for more effective techniques that allow for greater user control and practical integration into virtual environment production processes. This paper builds on previous efforts to address these limitations and improve the generation of human-object interactions in 3D environments.
Researchers from the University of Oxford and Carnegie Mellon University introduced a zero-shot method for synthesizing 3D human-object interactions using textual descriptions. The approach leverages text-to-image diffusion models to address challenges arising from diverse object geometries and limited datasets. It optimizes human mesh articulation by sampling gradients by distilling scores from these models. The method employs a dual implicit-explicit representation, combining neural radiance fields with skeleton-driven mesh articulation to preserve character identity. This innovative approach avoids extensive data collection, enabling the generation of realistic HOIs for a wide range of objects and interactions, advancing the field of 3D interaction synthesis.
DreamHOI employs a dual implicit-explicit representation, combining neural radiance fields (NeRF) with skeleton-driven mesh articulation. This approach optimizes human-skinned mesh articulation while preserving character identity. The method uses score distillation sampling to obtain gradients from pre-trained text-to-image diffusion models, which guides the optimization process. The optimization alternates between implicit and explicit ways, refining mesh articulation parameters to align with textual descriptions. Representing the skinned mesh together with the object mesh allows for direct optimization of explicit pose parameters, improving efficiency due to the reduced number of parameters.
Extensive experimentation validates DreamHOI’s efficacy. Ablation studies evaluate the impact of various components, including regularizers and rendering techniques. Qualitative and quantitative evaluations demonstrate the model’s performance against baselines. Various benchmark tests show the method’s versatility in generating high-quality interactions in different scenarios. The implementation of a guideline blending technique further improves the consistency of the optimization. This comprehensive methodology and rigorous testing establish DreamHOI as a robust approach for generating realistic and contextually appropriate human-object interactions in 3D environments.

DreamHOI excels at generating 3D human-object interactions from textual cues, outperforming baselines with higher CLIP similarity scores. Its implicit-explicit dual representation combines NeRF and skeleton-driven mesh articulation, allowing for flexible pose optimization while preserving character identity. The two-stage optimization process, including 5000 NeRF refinement steps, contributes to high-quality results. Regularizers play a crucial role in maintaining proper model size and alignment. A regressor facilitates transitions between NeRF and skinned mesh representations. DreamHOI overcomes the limitations of methods such as DreamFusion in maintaining mesh structure and identity. This approach holds promise for applications in film and game production, simplifying the creation of realistic virtual environments with interacting humans.

In conclusion, DreamHOI presents a new approach to generating realistic human-object interactions in 3D using textual cues. The method employs a dual implicit-explicit representation, combining NeRF with explicit pose parameters from skinned meshes. This approach, coupled with score distillation sampling, effectively optimizes pose parameters. Experimental results demonstrate DreamHOI’s superior performance compared to baseline methods, and ablation studies confirm the importance of each component. The paper addresses the challenges of direct pose parameter optimization and highlights DreamHOI’s potential to simplify the creation of virtual environments. This advancement opens up new possibilities for applications in the entertainment industry and beyond.
Take a look at the Paper and Project pageAll credit for this research goes to the researchers of this project. Also, don't forget to follow us on twitter.com/Marktechpost”>twitter and join our Telegram Channel and LinkedIn GrAbove!. If you like our work, you will love our fact sheet..
Don't forget to join our SubReddit of over 50,000 ml
FREE ai WEBINAR: 'SAM 2 for Video: How to Optimize Your Data' (Wednesday, September 25, 4:00 am – 4:45 am EST)
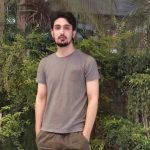
Shoaib Nazir is a Consulting Intern at MarktechPost and has completed his dual M.tech degree from Indian Institute of technology (IIT) Kharagpur. Being passionate about data science, he is particularly interested in the various applications of artificial intelligence in various domains. Shoaib is driven by the desire to explore the latest technological advancements and their practical implications in everyday life. His enthusiasm for innovation and solving real-world problems fuels his continuous learning and contribution to the field of ai.
<script async src="//platform.twitter.com/widgets.js” charset=”utf-8″>