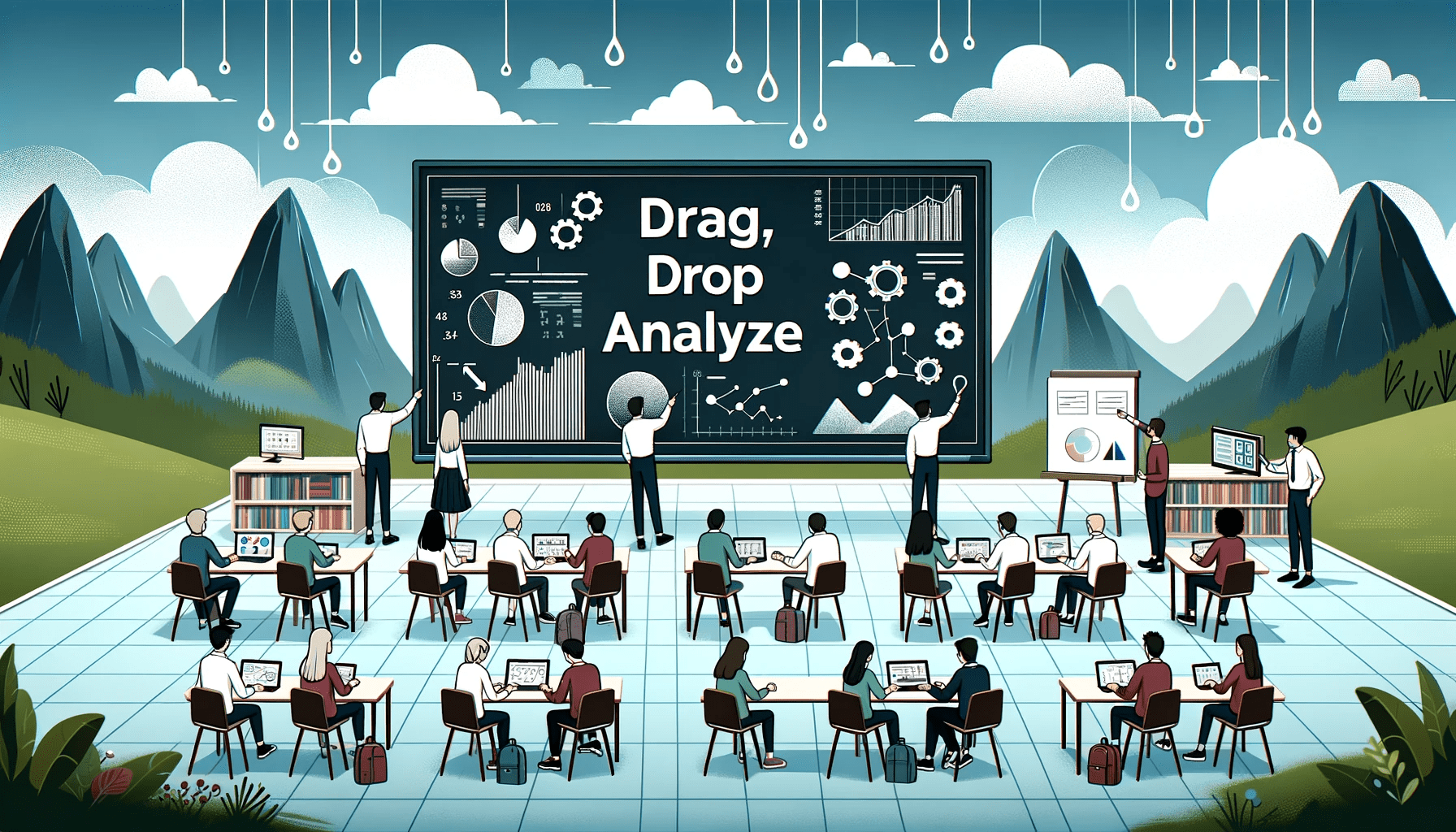
Image generated with DALLE 3
One of the challenges data professionals face is having to code everything from scratch for each new use case. This can be a slow and inefficient process. No-code or low-code solutions help data scientists create reusable solutions that can be applied to a wide range of use cases. This can save time and effort and improve the quality of data science projects.
You can do almost everything in data science without writing a single line of code. “No-code or low-code solutions are the future of data science,” he said. Ingo Mierswasenior vice president of product development at Altair and founder of fast miner, a data science platform. As an established inventor in the field of no-code data science, his experience and contributions have influenced the adoption and implementation of these capabilities in the industry. “These capabilities,” Mierswa said during our phone interview, “make it possible for people without much programming experience to build and deploy data science models. This can help democratize data science and make it more accessible to everyone.”
“When I found myself being a computer scientist, there was no no-code or low-code platform that recreated very similar solutions for each new use case. It was an inefficient process, which seemed like a huge waste of time,” shares Miesrwa. . Sticking with the basics, he articulated, “If you solve a problem a second time and you’re still coding, it means you didn’t solve it correctly the first time. You should have created a solution that can be reused to solve the problem.” same or similar problems over and over again. “People, he says, “often don’t realize how similar their problems are and, as a result, end up coding the same thing repeatedly.” The question they should ask themselves is, ‘Why do I keep coding?’ Maybe they shouldn’t do it to save time and effort.”
No-code or low-code data science solutions can be very rewarding. “The first and most important advantage is that they can lead to better forms of collaboration,” underlines Miesrwa. “Everyone can understand workflows or visual models if it is explained to them, however, not everyone is a computer scientist or programmer, and not everyone can understand code.” So to collaborate effectively, you need to understand what assets the team is collectively producing. “Data science is, at the end of the day, a team sport. You need people who understand business problems, regardless of whether they know how to code or not, since coding may not be their daily activity.”
Then there are other people who have access to the data, who are saturated with computational thinking, who think, “Okay, look, if I want to build, for example, some machine learning model, I need to transform my data in a specific way.” That’s a great skill and they need to collaborate as well, but again, for skills like that, we know that ETL products have been available for a long time. “Yes, in rare cases, in special, very personalized situations, you still need to code. Even in those situations, that’s the one percent exception,” Miesrwa said. “It shouldn’t be the norm, but the real magic happens when you bring together all the different skills, data, people and experience.”
“You’ll never see that with a code-only approach. You’ll never get stakeholder buy-in. That often leads to what I call dead projects. We should treat data science as a solution to problems. We shouldn’t treat it as a scientific approach, where it doesn’t matter whether we actually create a solution or not. Miesrwa reasoned. “It’s important. We’re solving multibillion-dollar business problems here. We should actually work to find a solution that works, get buy-in, implement it, and really improve our situation here. Without saying, ‘Yeah, I know what that is.'” If it fails, I don’t care.’ So collaboration is a huge benefit,” she stated.
Acceleration is another, explains Miesrwa. When you perform repetitive tasks by coding, you are not working as quickly as possible. If I create, for example, a RapidMiner workflow that consists of five or ten operators, that often equates to thousands of lines of code. Copying and pasting code can slow you down, but low-code platforms can help you build custom solutions faster.
Accountability, often easily overlooked, is the most important benefit. When building a code-based solution, it can be difficult to keep track of who made changes and why. “This can lead to problems when someone else needs to take over the project or when there is a bug in the code. On the other hand, low-code platforms are self-documenting. This means that the visual workflows you create are also accompanied by documentation that explains what the workflow does. “This makes it easier to understand and maintain the code, and also helps ensure accountability,” Miesrwa said. “People understand it.” They accept this, but they can also take ownership those results. Collectively, as a team.”
The torrent of advances in ai is transforming the data science landscape, and companies that want to stay ahead are open, using open source and open standards, and not hiding anything that is very important in the data science market. data.
Companies that have remained open have been in a winning position because the market moves quickly and requires constant iteration. “This is true for the overall data science market for the last 10 to 20 years,” Miesrwa reflected, “the fast-paced nature of the market requires constant iteration, so it is extremely unwise to shut down the ecosystem. This is part of “This is why some companies that have traditionally been closed have opened up and even taken a vendor-neutral approach to supporting more programming languages and integrations.”
While the code-optional approach allows researchers to perform complex data analysis tasks without writing a single line of code, there are situations where coding may be necessary. In such cases, most low-code platforms integrate with programming languages, machine learning librariesand deep learning environments. They also offer users the possibility to explore the market for third-party solutions, Miesrwa specified. “RapidMiner even provides an operator framework that allows users to create their own visual workflows. This operational framework makes it easy to extend and reuse workflows, providing a flexible and customizable approach to data analysis.”
Altair, a leader in computer science and artificial intelligence, conducted a survey that revealed the widespread adoption of data and artificial intelligence strategies in organizations around the world.
The research, which involved more than 2,000 professionals from various industries and 10 different countries, revealed a significant failure rate (ranging between 36% and 56%) for artificial intelligence and data analysis projects when there is friction between different departments within an organization.
The study identified three main sources of friction that hinder the success of data and ai projects: organizational, technological and financial.
- Organizational friction arises from challenges in finding qualified people to fill data science roles and a lack of ai knowledge among the workforce.
- Technological friction arises from limitations in data processing speed and issues with data quality.
- Financial friction is caused by limitations in funding, leaders’ focus on upfront costs, and perceptions of high implementation costs.
James R. Scapa, founder and CEO of Altair, in theai-and-data-projects” rel=”noopener” target=”_blank”> Press release emphasized the importance of organizations leveraging their data as a strategic asset to gain competitive advantage.
Friction paralyzes mission-critical projects. To overcome these challenges and achieve what Altair calls “frictionless ai,” companies must adopt self-service data analytics tools. These tools,” highlights Scapa, “enable non-technical users to navigate complex technological systems easily and cost-effectively, eliminating the friction that hinders progress.”
He also acknowledged that there are obstacles in the form of people, technology and investment, which prevent organizations from effectively leveraging data-driven insights. And by closing skills gaps, organizations can help build strong insights across cross-functional teams to overcome friction.
Saqib Khan is a technology writer and analyst with a passion for data science, automation, and cloud computing.