artificial intelligence (ai) increasingly relies on vast and diverse data sets to train models. However, a major issue has emerged regarding the transparency and legal compliance of these data sets. Researchers and developers often use data at scale without fully understanding its origins, proper attribution, or licensing terms. As ai continues to expand, these gaps in data transparency and licensing pose significant ethical and legal risks, making it crucial to audit and track data sets used in model development.
The central problem is the frequent use of unlicensed or improperly documented data in training ai models. Many datasets, especially those used to tune ai models, come from sources that do not provide clear licensing information. This results in high rates of misattribution or failure to comply with data usage conditions. The risks associated with such practices are serious, including exposure to legal action, as models trained with unlicensed data could violate copyright laws. Furthermore, these issues raise ethical concerns regarding the use of the data, particularly when it contains personal or sensitive information.
While some platforms attempt to organize and provide licenses for datasets, many need to do so accurately. Platforms such as GitHub and Hugging Face, which host popular ai datasets, often contain incorrect or incomplete licensing information. Studies have shown that over 70% of licenses on these platforms are unspecified and nearly 50% contain errors. This leaves developers in need of clarification on their legal obligations when using such datasets, which is particularly worrying given the increasing scrutiny of data use in ai. The widespread lack of transparency not only complicates the development of ai models, but also risks producing models that are legally vulnerable.
Researchers from institutions such as MIT, Google, and other leading institutions have introduced the Data Provenance Explorer (DPExplorer) to address these concerns. This innovative tool was designed to help ai practitioners audit and trace the provenance of datasets used for training. DPExplorer allows users to view the origins, licenses, and terms of use of over 1,800 popular text datasets. By offering a detailed view of the source, creator, and license of each dataset, the tool enables developers to make informed decisions and avoid legal risks. This effort was a comprehensive collaborative initiative between legal experts and ai researchers, ensuring that the tool addresses both the technical and legal aspects of using the datasets.
DPExplorer employs an extensive process to collect and verify metadata from widely used ai datasets. Researchers meticulously audit each dataset, recording details such as license terms, dataset source, and modifications made by previous users. The tool extends existing metadata repositories like Hugging Face by offering a more comprehensive taxonomy of dataset characteristics, including language composition, task type, and text length. Users can filter datasets by commercial or noncommercial licenses and review how datasets have been repackaged and reused in different contexts. The system also automatically generates data provenance cards, summarizing metadata for easy reference and helping users identify datasets suited to their specific needs without stepping outside legal boundaries.
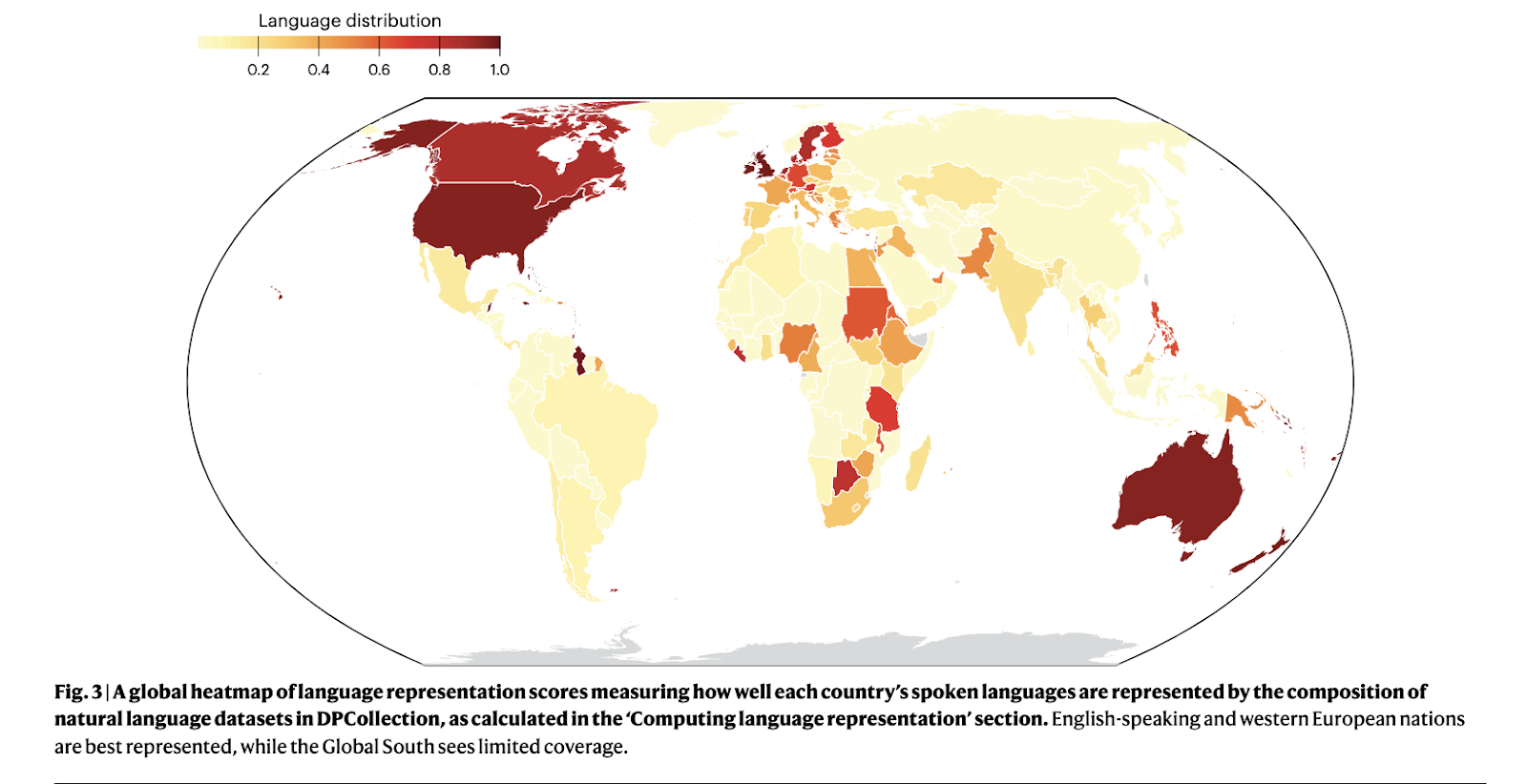
In terms of performance, DPExplorer has already yielded significant results. The tool successfully reduced the number of unspecified licenses from 72% to 30%, marking a substantial improvement in dataset transparency. Of the audited datasets, 66% of permissions on platforms like Hugging Face were misclassified, with many flagged as more permissive than the original author’s license. Additionally, over 1,800 text datasets were crawled to check the accuracy of licenses, allowing for a better understanding of the legal conditions under which ai models can be developed. The findings reveal a critical divide between datasets licensed for commercial use and those restricted to non-commercial purposes, with the latter being more diverse and creative in their content.
The researchers observed that datasets used for commercial purposes often need a greater diversity of tasks and topics than non-commercial datasets. For example, non-commercial datasets feature more creative and open-ended tasks, such as creative writing and problem solving. In contrast, commercial datasets often focus more on short text generation and classification tasks. Furthermore, 45% of non-commercial datasets were generated synthetically using models such as OpenAI’s GPT, while commercial datasets were primarily derived from human-generated content. This stark difference in dataset types and their use indicates the need for more careful consideration of licensing when selecting training data for ai models.
In conclusion, the research highlights a significant gap in the licensing and attribution of ai datasets. The introduction of DPExplorer addresses this challenge by providing developers with a robust tool to audit and track dataset licenses. This ensures that ai models are trained on properly licensed data, reducing legal risks and promoting ethical practices in the field. As ai evolves, tools like DPExplorer will ensure that data is used responsibly and transparently.
Take a look at the Paper. All credit for this research goes to the researchers of this project. Also, don't forget to follow us on twitter.com/Marktechpost”>twitter and LinkedInJoin our Telegram Channel. If you like our work, you will love our fact sheet..
Don't forget to join our SubReddit of over 50,000 ml
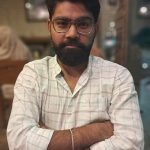
Nikhil is a Consultant Intern at Marktechpost. He is pursuing an integrated dual degree in Materials from Indian Institute of technology, Kharagpur. Nikhil is an ai and Machine Learning enthusiast who is always researching applications in fields like Biomaterials and Biomedical Science. With a strong background in Materials Science, he is exploring new advancements and creating opportunities to contribute.
<script async src="//platform.twitter.com/widgets.js” charset=”utf-8″>