Movies are among the most artistic expressions of stories and feelings. For instance, in “The Pursuit of Happyness,” the protagonist goes through a range of emotions, experiencing lows such as a breakup and homelessness and highs like achieving a coveted job. These intense feelings engage the audience, who can relate to the character’s journey. To comprehend such narratives in the artificial intelligence (AI) domain, it becomes crucial for machines to monitor the development of characters’ emotions and mental states throughout the story. This objective is pursued by utilizing annotations from MovieGraphs and training models to observe scenes, analyze dialogue, and make predictions regarding characters’ emotional and mental states.
The subject of emotions has been extensively explored throughout history; from Cicero’s four-way classification in Ancient Rome to contemporary brain research, the concept of emotions has consistently captivated humanity’s interest. Psychologists have contributed to this field by introducing structures such as Plutchik’s wheel or Ekman’s proposition of universal facial expressions, offering diverse theoretical frameworks. Affective emotions are additionally categorized into mental states encompassing affective, behavioral, and cognitive aspects and bodily states.
In a recent study, a project known as Emotic introduced 26 distinct clusters of emotion labels when processing visual content. This project suggested a multi-label framework, allowing for the possibility that an image might convey various emotions simultaneously, such as peace and engagement. As an alternative to the conventional categorical approach, the study also incorporated three continuous dimensions: valence, arousal, and dominance.
The analysis must encompass various contextual modalities to predict an extensive array of emotions accurately. Prominent pathways in multimodal emotion recognition include Emotion Recognition in Conversations (ERC), which involves categorizing emotions for each instance of dialogue exchange. Another approach is predicting a singular valence-activity score for short segments of movie clips.
Operating at the level of a movie scene entails working with a collection of shots that collectively tell a sub-story within a specific location, involving a defined cast and occurring over a brief time frame of 30 to 60 seconds. These scenes offer significantly more duration than individual dialogues or movie clips. The objective is to forecast the emotions and mental states of every character in the scene, including the accumulation of labels at the scene level. Given the extended time window, this estimation naturally leads to a multi-label classification approach, as characters may convey multiple emotions simultaneously (such as curiosity and confusion) or undergo transitions due to interactions with others (for instance, shifting from worry to calm).
Furthermore, while emotions can be broadly categorized as part of mental states, this study distinguishes between expressed emotions, which are visibly evident in a character’s demeanor (e.g., surprise, sadness, anger), and latent mental states, which are discernible only through interactions or dialogues (e.g., politeness, determination, confidence, helpfulness). The authors argue that effectively classifying within an extensive emotional label space necessitates considering the multimodal context. As a solution, they propose EmoTx, a model that concurrently incorporates video frames, dialog utterances, and character appearances.
An overview of this approach is presented in the figure below.
EmoTx utilizes a Transformer-based approach to identify emotions on a per-character and movie scene basis. The process begins with an initial video pre-processing and feature extraction pipeline, which extracts relevant representations from the data. These features include video data, character faces, and text features. In this context, suitable embeddings are introduced to the tokens for differentiation based on modalities, character enumeration, and temporal context. Additionally, tokens functioning as classifiers for individual emotions are generated and linked to the scene or particular characters. Once embedded, these tokens are combined using linear layers and fed to a Transformer encoder, enabling information integration across different modalities. The classification component of the method draws inspiration from previous studies on multi-label classification employing Transformers.
An example of EmoTx’s behavior published by the authors and related to a “Forrest Gump” scene is reported in the following figure.
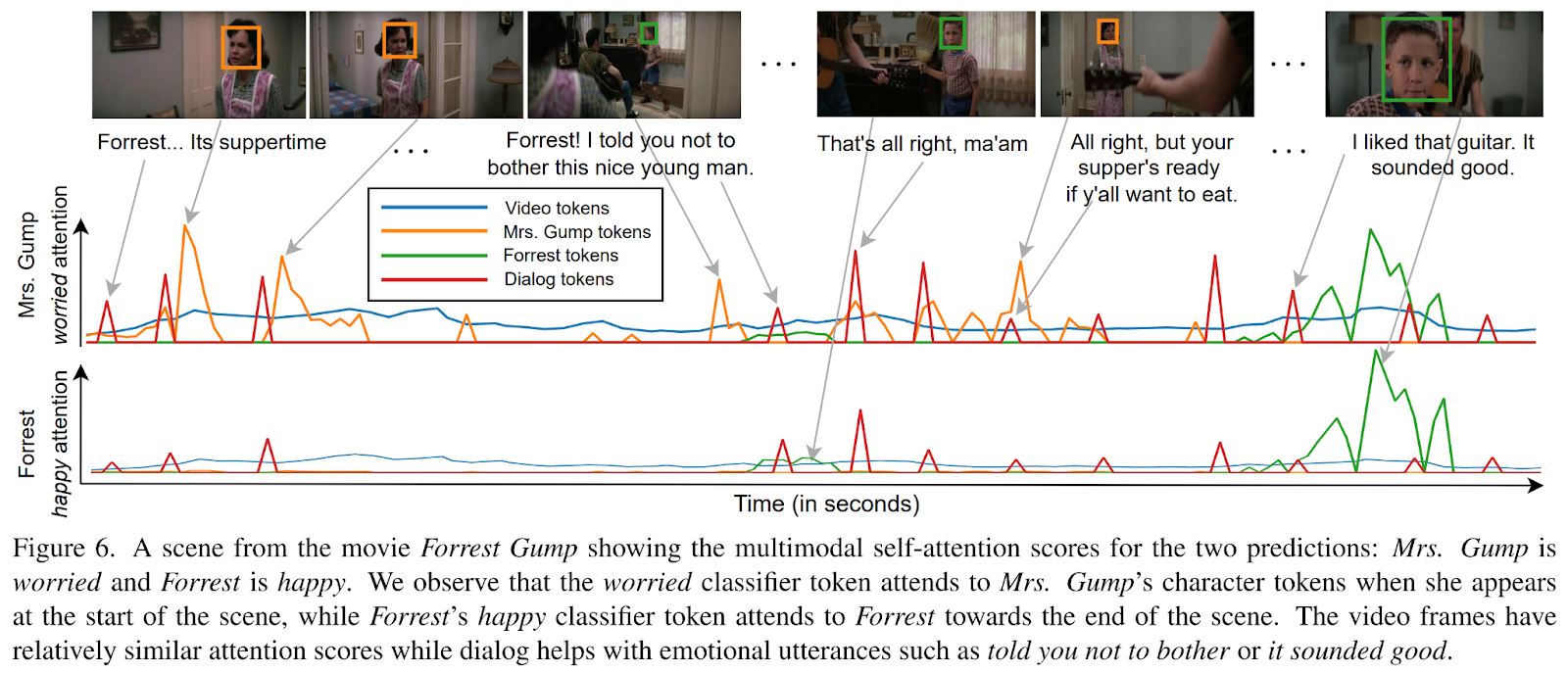
This was the summary of EmoTx, a novel AI Transformer-based architecture EmoTx that predicts the emotions of subjects appearing in a video clip from suitable multimodal data. If you are interested and want to learn more about it, please feel free to refer to the links cited below.
Check out the Paper and Project. All Credit For This Research Goes To the Researchers on This Project. Also, don’t forget to join our 29k+ ML SubReddit, 40k+ Facebook Community, Discord Channel, and Email Newsletter, where we share the latest AI research news, cool AI projects, and more.
If you like our work, please follow us on Twitter
Daniele Lorenzi received his M.Sc. in ICT for Internet and Multimedia Engineering in 2021 from the University of Padua, Italy. He is a Ph.D. candidate at the Institute of Information Technology (ITEC) at the Alpen-Adria-Universität (AAU) Klagenfurt. He is currently working in the Christian Doppler Laboratory ATHENA and his research interests include adaptive video streaming, immersive media, machine learning, and QoS/QoE evaluation.