Mathematical reasoning in artificial intelligence represents a frontier that has long challenged researchers and developers. While effective for specific tasks, traditional computational methods often need to catch up when faced with the complexities and nuances of complex mathematical problems. This limitation has stimulated the search for more sophisticated solutions, leading to the exploration of large language models (LLMs) as potential vehicles for advanced mathematical reasoning. The development of these models marks a fundamental shift towards harnessing the vast capabilities of ai to decipher, interpret and solve mathematical challenges.
At the forefront of this innovation are Tsinghua University's DeepSeek-ai and Peking University's DeepSeekMath, an innovative language model designed specifically to navigate the complexities of mathematical reasoning. Unlike conventional models that rely on a limited scope of predefined algorithms and data sets, DeepSeekMath benefits from a rich and diverse training experience. The genesis of this model lies in the strategic compilation of a vast data set comprising over 120 billion tokens of mathematics-related content from the vast realms of the Internet. This approach broadens the model's exposure to a wide range of mathematical concepts and enriches its understanding, allowing it to address various mathematical problems with unprecedented precision.
What sets DeepSeekMath apart is its innovative training methodology, particularly using Group Relative Policy Optimization (GRPO). This variant of reinforcement learning represents an important advance, as it optimizes the problem-solving capabilities of the model while efficiently managing memory usage. The effectiveness of GRPO is evident in DeepSeekMath's ability to formulate step-by-step solutions to complex mathematical problems. This feat reflects human problem-solving processes and exceeds the capabilities of previous models.
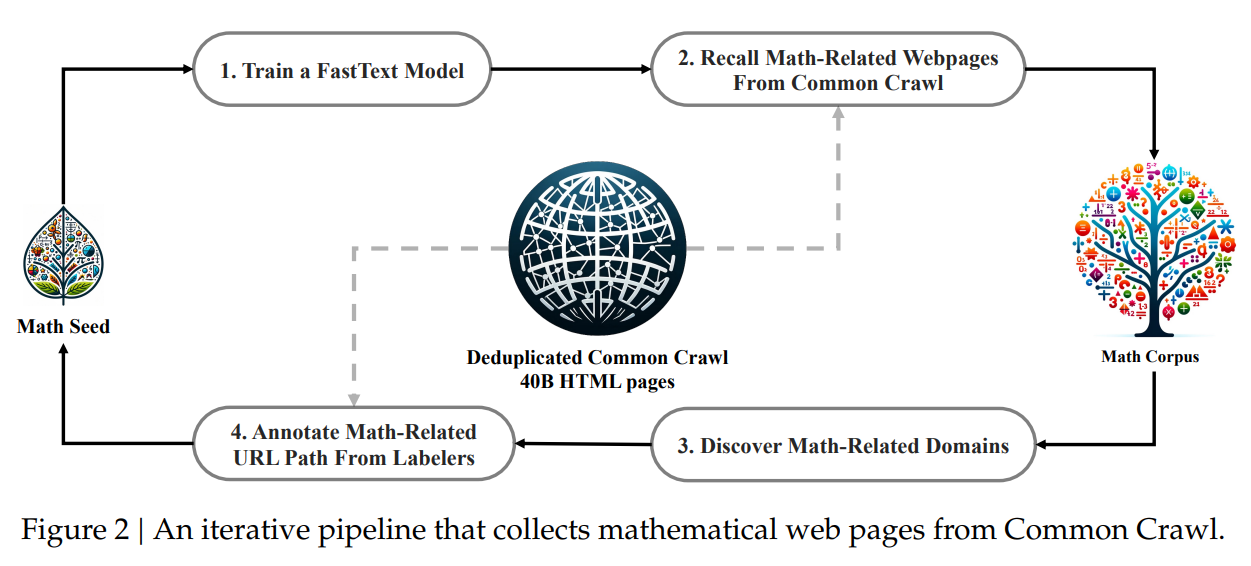
The performance and results of the DeepSeekMath model demonstrate superior mathematical reasoning on a variety of benchmarks and show significant improvements over existing open source models. Highlights include:
- Achieving a superior accuracy of 51.7% on the competitive MATH benchmark is a testament to your advanced reasoning capabilities.
- It outperformed models many times its size, illustrating that data quality and the efficiency of learning algorithms can surpass sheer computational power.
- The successful application of GRPO has been shown to markedly improve performance, setting a new standard for the integration of reinforcement learning in training language models for mathematical reasoning.
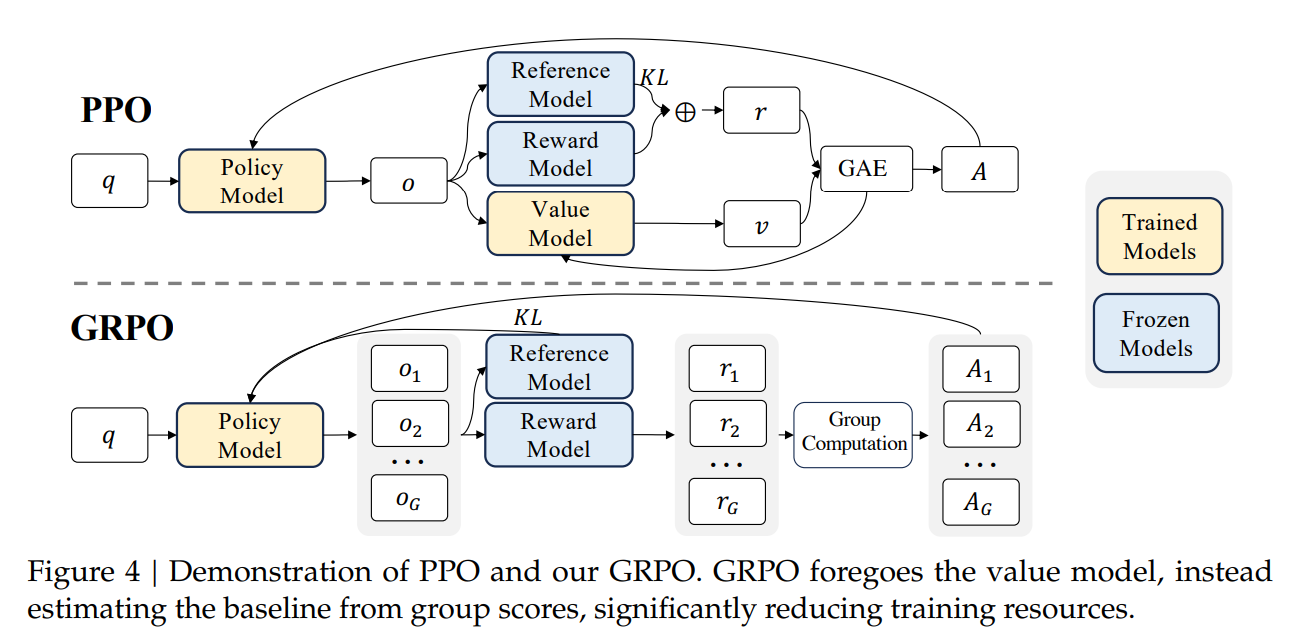
This research not only highlights the potential of ai to revolutionize mathematical reasoning, but also opens new avenues of exploration. The success of DeepSeekMath paves the way for further advances in ai-powered mathematics, offering promising prospects for educational tools, research assistance, and more. The convergence of ai and mathematics through initiatives like DeepSeekMath heralds a future in which the limits of what machines can understand and solve will continue to expand, closing the gaps between computational intelligence and the complex beauty of mathematics.
Review the Paper. All credit for this research goes to the researchers of this project. Also, don't forget to follow us on Twitter and Google news. Join our 36k+ ML SubReddit, 41k+ Facebook community, Discord channeland LinkedIn Grabove.
If you like our work, you will love our Newsletter..
Don't forget to join our Telegram channel
Hello, my name is Adnan Hassan. I'm a consulting intern at Marktechpost and soon to be a management trainee at American Express. I am currently pursuing a double degree from the Indian Institute of technology, Kharagpur. I am passionate about technology and I want to create new products that make a difference.
<!– ai CONTENT END 2 –>