A new one investigation addresses a critical problem in large multimodal language models (MLLMs): the phenomenon of object hallucination. Object hallucination occurs when these models output descriptions of objects that are not present in the input data, leading to inaccuracies that undermine their reliability and effectiveness. For example, a model might incorrectly assert the presence of a “tie” in an image of a “wedding cake” or misidentify objects in a scene due to learned associations rather than actual observations. This problem is particularly pressing as MLLMs are increasingly deployed in applications requiring high accuracy, such as visual question answering and image captioning. The authors highlight that existing methods for mitigating hallucinations often come with significant drawbacks, including increased inference time, the need for extensive retraining, and potential degradation of overall model performance on general tasks.
To address this problem, this paper from Queen's University, the Vector Institute, Google Cloud ai Research, and Google DeepMind proposes a new method called Data-Augmented Contrastive Tuning (DACT). This approach builds on the foundations of existing MLLM frameworks but introduces a more efficient mechanism to reduce hallucination rates without compromising overall model capabilities. MLLMs trained using this framework are called Hallucination Attenuated Language and Vision Assistant (HALVA). Current methods for addressing object hallucination can be categorized into inference-based, pre-training, and fine-tuning techniques. Inference-based methods often slow down model response time, while pre-training techniques require large amounts of data and are not easily applicable to off-the-shelf models. While effective, fine-tuning methods can decrease model performance on other vision and language tasks. However, DACT employs a two-pronged strategy: it generates hallucinated responses using data augmentation and applies a contrastive tuning objective to reduce the likelihood of these hallucinations occurring during speech generation. This method allows for minimal retraining and maintains model performance across multiple tasks.
The proposed DACT method consists of two main components: generative data augmentation and contrastive fine-tuning. In the first step, the authors create hallucinated responses by selectively altering correct responses based on the input data. This involves replacing certain objects with others that match but are incorrect in the proper response, generating a set of contrastive pairs. For example, if the correct response describes a scene with a “fork,” the augmented response might include a “spoon” or a “knife” that does not appear in the input image. The second component, contrastive fine-tuning, focuses on minimizing the likelihood of generating these hallucinated tokens relative to correct tokens. This is achieved through a contrastive objective that encourages the model to favor accurate descriptions while maintaining a KL divergence constraint to ensure that the model does not deviate significantly from its original performance.
The results indicate that HALVA significantly reduces hallucination rates, while maintaining or even improving model performance on general tasks. For example, on the AMBER benchmark, HALVA variants demonstrate a marked decrease in hallucination rates compared to existing fine-tuning methods such as HA-DPO and EOS. Specifically, the HALVA-7B and HALVA-13B models show substantial reductions in object hallucination rates, improving both instance-level and sentence-level evaluations.
On visual question answering tasks, HALVA also outperforms the baseline model and other fine-tuning methods, achieving higher F1 scores and demonstrating its effectiveness in mitigating hallucinations while preserving overall accuracy. The authors also highlight that HALVA’s benefits extend beyond object hallucination, improving performance on other vision and language hallucinations, as evaluated by the HallusionBench benchmark.
In conclusion, the research presents a compelling solution to the problem of object hallucination in multimodal models by introducing data-augmented contrastive tuning. By effectively mitigating hallucination rates while preserving overall model performance, this method addresses a significant challenge in the implementation of multimodal models. The combination of generative data augmentation and contrastive tuning offers a promising avenue for improving the reliability of multimodal models, paving the way for their broader application in tasks requiring accurate visual understanding and language generation. The potential impact of the DACT method is significant and offers a promising future for the field of artificial intelligence and machine learning.
Take a look at the PaperAll credit for this research goes to the researchers of this project. Also, don't forget to follow us on twitter.com/Marktechpost”>twitter and join our Telegram Channel and LinkedIn GrAbove!. If you like our work, you will love our fact sheet..
Don't forget to join our Subreddit with over 48 billion users
Find upcoming ai webinars here
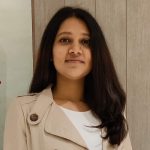
Shreya Maji is a Consulting Intern at MarktechPost. She pursued her Bachelors from the Indian Institute of technology (IIT) in Bhubaneswar. She is an ai enthusiast and enjoys keeping herself updated about the latest advancements. Shreya is particularly interested in real-life applications of cutting-edge technology, especially in the field of data science.
<script async src="//platform.twitter.com/widgets.js” charset=”utf-8″>