Introduction
In an era defined by technological innovation and rapid digitalization, e-commerce has become a cornerstone of modern business. With a global reach and immense potential for growth, online retail has reshaped consumer behaviors and expectations. In this digital marketplace, where countless products and services compete for attention, the ability to effectively connect with customers has never been more crucial. Welcome to the world of “E-commerce Reinvented: The Role of Large Language Models (LLMs).” This article delves into the transformative impact of Large Language Models on the e-commerce landscape. These sophisticated ai-driven systems, capable of understanding and generating human-like text, are reshaping the way businesses engage with their customers, tailor their marketing strategies, and optimize their operations.
From enhancing customer interactions and personalizing product recommendations to streamlining customer support and enabling advanced natural language processing in search. The article will also shed light on the benefits, challenges, and ethical considerations associated with deploying LLMs in the e-commerce ecosystem.
Learning Objectives
- Develop a foundational understanding of Large Language Models, their capabilities, and their role in transforming e-commerce marketing.
- Discover how Large Language Models are seamlessly integrated into e-commerce marketing strategies, driving personalized content creation and engagement.
- Explore the tangible benefits of using LLMs in e-commerce marketing, from enhancing customer engagement to improving conversion rates.
- Examine the potential challenges and ethical considerations when implementing LLMs in marketing, including privacy concerns and content quality.
- Gain insights into real-life examples of e-commerce businesses effectively utilizing LLMs for customized marketing copywriting, with practical takeaways for your own strategies.
- Look ahead to the future of LLMs in e-commerce marketing, considering evolving technologies and their potential impact on the industry.
This article was published as a part of the Data Science Blogathon.
<h2 class="wp-block-heading" id="h-generative-ai-overview”>Generative ai Overview
A Generative ai, often referred to as a Large Language Model (LLM), is akin to an avid learner in a vast library, absorbing knowledge from an extensive array of texts, books, articles, and websites. Just like that diligent individual, the LLM spends its time comprehending and deciphering the vast amount of information it encounters.
Through this immersive learning process, the LLM becomes exceptionally knowledgeable across various subjects, much like our dedicated reader, who becomes well-versed in a multitude of topics. It can answer questions, engage in logical conversations, and provide meaningful explanations based on the vast corpus of text it has absorbed.
The LLM’s modus operandi involves delving into an extensive collection of text, which serves as its virtual library, including books, websites, and articles. As it immerses itself in this sea of information, it deciphers the intricacies of word structures, and sentence compositions, discerns meanings, and understands how words and sentences interconnect. Once fully trained, the LLM emerges as an intelligent computer program that can generate responses, offer elucidations, and engage in dialogues informed by the input it receives. It possesses the remarkable ability to grasp context, thereby generating coherent and contextually relevant text.
Prominent Large Language Models (LLMs) in the Industry
In the rapidly evolving field of large language models (LLMs), several standout models have gained prominence. These models represent the cutting edge of natural language processing technology and are utilized across various industries for a multitude of applications. Comparing these prominent LLMs allows us to understand their unique features, strengths, and areas of specialization. By examining how these models differ, we can gain insights into their potential applications and the specific challenges they address. This comparison sheds light on the capabilities and limitations of each LLM, aiding businesses and researchers in selecting the most suitable model for their specific needs and objectives.
GPT-3 (Generative Pre-trained Transformer 3)
GPT-3, unveiled in 2020 and developed by OpenAI, has emerged as a true giant in the LLM landscape. Its distinguishing feature lies in its size and depth, with a staggering 175 billion parameters. This extensive training allows GPT-3 to produce text outputs that are remarkably close to human-like in quality. What makes it even more accessible to businesses is its partnership with Microsoft, which now licenses GPT-3, making it widely available. Among its standout applications is ChatGPT, a highly popular ai chatbot. Furthermore, GPT-3 offers a public API, enabling seamless integration into various applications and systems.
BERT (Bidirectional Encoder Representations from Transformers)
Introduced by Google in 2018, BERT represents a significant leap in Natural Language Processing (NLP). Unlike previous models that looked at words in isolation, BERT understands the context in which words appear by considering both left and right contexts. It achieves this by training on massive plain text datasets, including sources like Wikipedia. This bidirectional comprehension is invaluable for understanding user queries and delivering contextually relevant responses, making it indispensable in e-commerce search and recommendation systems.
LaMDA (Language Model for Dialogue Applications)
Google’s LaMDA, unveiled in 2022, stands out as a conversational LLM. What sets it apart is its focus on dialogue and conversation, a crucial aspect in e-commerce for engaging customers. LaMDA employs a decoder-only transformer language model and is pre-trained on an astonishingly vast corpus of text containing 1.56 trillion words. This corpus encompasses not only documents but also dialogues, making it ideal for powering conversational ai. Google utilizes LaMDA in its ai chatbot, Bard, and extends its capabilities through a Generative Language API, opening doors for third-party applications to enhance customer interactions.
PaLM (Pathways Language Model)
Another noteworthy creation from Google ai, PaLM, was introduced in 2022. It places significant emphasis on the quality of training data. PaLM is pre-trained on a diverse range of high-quality datasets, including filtered web pages, books, Wikipedia articles, news articles, source code from open-source repositories on GitHub, and even social media conversations. This meticulous curation of data ensures that PaLM understands and generates text that aligns with users’ expectations, making it a valuable asset for content generation and customer engagement in e-commerce.
<h4 class="wp-block-heading" id="h-llama-large-language-model-meta-ai“>LLaMA (Large Language Model Meta ai)
Facebook entered the LLM arena in 2023 with the introduction of LLaMA. Similar to other LLMs, LLaMA operates by taking a sequence of words as input and predicting the subsequent word, enabling it to generate coherent text. What sets LLaMA apart is its focus on linguistic diversity. It was trained on text from the 20 languages with the most speakers, with a particular emphasis on languages using Latin and Cyrillic alphabets. This linguistic diversity empowers businesses to reach a broader audience, facilitating multilingual marketing and customer engagement.
GPT-4 (Generative Pre-trained Transformer 4)
Building upon the success of GPT-3, OpenAI introduced GPT-4 as a multimodal LLM. What makes it particularly powerful is its ability to process both images and text as input. This multimodal capability opens up a myriad of possibilities in e-commerce, such as analyzing product images, summarizing customer reviews, or even generating images based on textual descriptions. GPT-4 also enables the creation of APIs, allowing businesses to develop innovative applications that combine the strengths of text and images. Additionally, it powers ChatGPT Plus, enhancing customer interactions with advanced conversational ai.
These top LLMs represent the cutting edge of ai technology and are revolutionizing e-commerce and marketing. They offer businesses the means to create personalized, engaging content, deliver exceptional customer experiences, and gain valuable insights from data analysis, shaping the future of online commerce.
The Significance of Large Language Models in E-commerce Marketing
The advent of artificial intelligence and machine learning models has ushered in significant advancements across various industries, and e-commerce is no exception. Large language models, such as GPT-3, have emerged as transformative tools, reshaping the way businesses engage with their customers, elevating personalization efforts, and bolstering overall operational efficiency.
Enhanced Customer Interaction
Large language models in e-commerce play a pivotal role in augmenting customer interactions. These models possess the ability to comprehend and generate human-like text, enabling businesses to deliver tailored assistance and support to their clientele. Through the utilization of chatbots or virtual assistants powered by large language models, e-commerce platforms can actively engage with customers in real time. They adeptly address queries, furnish product recommendations leveraging machine learning algorithms, and facilitate seamless transactions. This heightened level of responsiveness and interactivity greatly enriches the customer experience, fostering trust, and culminating in heightened customer satisfaction and loyalty.
Precision in Product Recommendations
Large language models excel in generating precise and pertinent product recommendations. By scrutinizing customer preferences, past purchase history, and browsing behavior, these models can proffer personalized product suggestions aligned with individual interests and needs. This degree of personalization not only aids customers in discovering products that resonate with them but also empowers businesses to drive increased sales and amplify customer engagement. The integration of large language models allows e-commerce platforms to harness the potential of data, delivering tailored recommendations that elevate the overall shopping experience.
Streamlined Customer Support
Efficient customer support is a linchpin of e-commerce, and large language models have significantly elevated the efficiency and efficacy of this critical function. With the assistance of language models, businesses can automate and streamline their customer support operations. ai-driven chatbots, underpinned by large language models, can proficiently address a wide array of customer inquiries, furnishing instant responses and escalating complex issues to human agents when necessary. This automation translates into reduced response times, the capacity to manage a higher volume of customer inquiries concurrently, and ultimately, elevated customer satisfaction. Moreover, continuous training of large language models on customer interactions empowers them to learn and evolve over time, leading to even greater precision and helpfulness in customer support.
Natural Language Processing (NLP) in Search
Large language models exhibit prowess in Natural Language Processing (NLP), enabling more advanced search functionalities within e-commerce platforms. Customers can employ conversational queries while shopping and receive highly accurate search results that align with their intent. For instance, a customer might pose a question like, “Which smartphones offer the best camera quality within a budget of $500?” The large language model can interpret such inquiries, discern the customer’s specific requirements, and present relevant product options. This elevated search capability simplifies the shopping experience, reduces the time spent searching for products, and ultimately augments overall customer satisfaction.
Content Generation and Marketing
E-commerce heavily relies on content generation for crafting product descriptions, marketing materials, and nurturing customer engagement. Large language models prove invaluable in generating high-quality content by comprehending and emulating a brand’s writing style, tone, and voice. These models can produce captivating product descriptions, compelling marketing copies, and even craft personalized emails based on customer interactions. The capacity to generate ai-driven content not only conserves time and resources for businesses but also ensures a consistent and coherent brand message across diverse channels. Large language models contribute significantly to enhancing marketing endeavors, translating into heightened customer engagement and improved conversion rates.
Data-Driven Decision Making
Large language models empower e-commerce businesses to harness the power of data for informed decision-making. By analyzing customer interactions, feedback, and purchase patterns, these models can identify emerging trends and insights. This data-driven approach guides marketing decisions, inventory management, and product development strategies, resulting in more effective and efficient operations.
Implementing and Scaling LLMs in E-Commerce
Integrating large language models into your marketing strategy begins with a comprehensive evaluation of your current approach. Identify areas where automation and personalization can yield benefits, ranging from content creation to customer engagement. Once potential areas for improvement are identified, the next step involves determining which tasks should be automated with LLMs. The implementation process may vary based on your specific requirements and the capabilities of the chosen LLMs. Collaborating with ai developers or vendors can facilitate a seamless transition and ensure the successful integration of these powerful tools into your e-commerce ecosystem.
Challenges and Solutions in LLM Implementation in E-commerce
The integration of Large Language Models (LLMs) into the e-commerce landscape undoubtedly offers a plethora of advantages, from enhancing customer interactions to delivering personalized marketing content. However, this transformative technology also brings along its set of challenges and ethical considerations that businesses must navigate to fully harness its potential.
Bias and Fairness
One of the foremost concerns in the realm of LLMs applied to e-commerce is the potential for biased or unfair responses. These language models acquire their knowledge from extensive datasets, which can inadvertently include societal biases. When an LLM generates content or recommendations, it may unknowingly favor certain demographic groups, perpetuate stereotypes, or exhibit subtle biases. Such biases can harm a brand’s reputation, alienate customers, and even lead to legal ramifications. To mitigate these challenges, it is imperative to actively monitor and evaluate the output of LLMs. Additionally, companies should implement strategies to reduce bias in the model’s recommendations and responses. This might involve fine-tuning the model on more diverse and inclusive datasets, implementing fairness algorithms, or conducting regular audits of generated content.
Ethical Usage
Ethical considerations are paramount when implementing LLMs in e-commerce. It’s essential to define clear guidelines and principles for the responsible use of this technology. This includes safeguarding customer data, respecting privacy rights, and adhering to ethical standards and regulations. Businesses must establish transparent policies for how LLMs are employed in marketing, customer interactions, and data handling. Moreover, ethical training for personnel involved in LLM implementation is crucial to ensure that they understand the potential ethical pitfalls and how to navigate them. This may involve the creation of ethical ai committees or boards within organizations to oversee and guide LLM usage.
Content Quality
While LLMs are powerful tools for generating content, they are not without their imperfections. There is a possibility that LLMs may produce content that is irrelevant, nonsensical, or even inappropriate. Such content can detract from the user experience, diminish brand credibility, and deter customers. Therefore, a proactive approach to managing the quality of LLM-generated content is essential. This includes implementing robust quality control measures, conducting regular content audits, and fine-tuning the model’s responses based on user feedback. Furthermore, human oversight remains critical in ensuring the accuracy and relevance of LLM-generated content.
Implementing and Scaling LLMs in E-commerce
Implementing and scaling Large Language Models (LLMs) in the context of e-commerce involves the process of integrating these advanced ai models into an online retail business’s operations and expanding their use to enhance various aspects of the business. Here’s an explanation of this point:
Implementing LLMs in E-Commerce
- Integration Planning: The first step in implementing LLMs is to carefully plan how and where these models can be integrated into the e-commerce ecosystem. This requires identifying specific areas and tasks where LLMs can bring value, such as customer support, content generation, personalized marketing, and product recommendations.
- Selecting LLMs: Choosing the right LLM is crucial. E-commerce businesses need to evaluate different models available in the market, considering factors like the model’s capabilities, compatibility with their systems, and the extent to which it aligns with their business objectives.
- Customization: Once a suitable LLM is selected, it may require customization to align with the unique needs and branding of the e-commerce platform. This involves training the model on specific datasets related to the business and configuring it to understand and generate content that resonates with the target audience.
- Testing and Validation: Before deploying LLMs at scale, thorough testing and validation are essential. Businesses should conduct rigorous testing to ensure that the ai-driven systems work as intended, provide accurate responses, and enhance user experiences.
Scaling LLMs in E-Commerce
- Infrastructure Scaling: As the usage of LLMs grows within an e-commerce platform, the infrastructure must scale accordingly to handle increased computational demands. This may involve cloud-based solutions or dedicated hardware resources to support the ai models.
- Data Management: Scaling LLMs also means managing larger volumes of data. E-commerce businesses need effective data management strategies to collect, store, and process the data required for LLMs to function optimally. This includes customer data, product information, and user interactions.
- Continuous Learning: To keep LLMs effective and up-to-date, businesses should implement mechanisms for continuous learning. This involves regularly updating the model with new data, customer feedback, and market trends to ensure that it remains relevant and capable of providing accurate recommendations and responses.
- Monitoring and Optimization: Ongoing monitoring of LLMs is crucial to identifying any issues or deviations from desired performance. Regular optimization is necessary to fine-tune the models, improve response accuracy, and address any emerging challenges.
- User Training: Both customers and employees may need training on how to interact with ai-powered systems effectively. Providing resources and guidance on using LLM-driven features can improve user satisfaction and adoption.
- Scalable Business Processes: E-commerce businesses should adapt their processes to accommodate the capabilities of LLMs. This includes streamlining workflows to leverage ai automation effectively and integrating ai-driven insights into decision-making processes.
In summary, implementing and scaling LLMs in e-commerce involves careful planning, selection, customization, testing, and continuous optimization of these advanced ai models. It also requires adapting infrastructure, data management, and business processes to harness the full potential of LLMs in improving customer experiences and driving business growth.
<h2 class="wp-block-heading" id="h-example-of-ai-integration-in-e-commerce-with-real-life-use-cases”>Example of ai Integration in E-commerce with Real-Life Use Cases
ai integration in e-commerce has transformed the way businesses operate, enhancing customer experiences, streamlining processes, and boosting sales. Here are some real-life use cases that illustrate how ai is integrated into e-commerce:
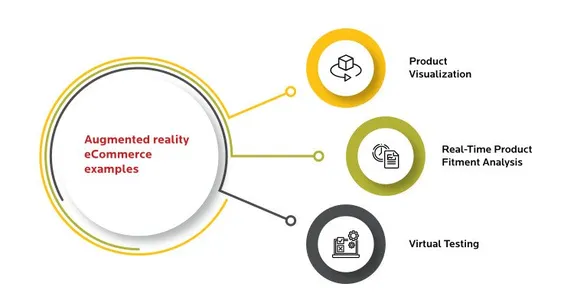
Chatbots for Customer Support
Use Case: Many e-commerce websites have integrated ai-powered chatbots into their customer support systems. For example, an online clothing store might employ a chatbot to assist customers with inquiries about product availability, sizing, or returns.
How it Works: Chatbots use natural language processing (NLP) to understand customer queries and provide relevant responses. They can handle routine inquiries, offer product recommendations, and even assist with the checkout process.
Benefits: ai chatbots provide 24/7 support, reduce response times, and free up human agents to focus on more complex issues. This results in improved customer satisfaction and operational efficiency.
Personalised Product Recommendations
Use Case: E-commerce giants like Amazon leverage ai algorithms to deliver personalized product recommendations. When a user browses for items, ai analyzes their browsing and purchase history to suggest relevant products.
How it Works: ai algorithms use collaborative filtering, user behavior analysis, and machine learning to understand individual preferences and make product recommendations in real time.
Benefits: Personalise recommendations increase the chances of customers discovering products they are interested in, leading to higher conversion rates and sales.
Dynamic Pricing Optimization
Use Case: Airlines and online marketplaces like eBay use ai-driven dynamic pricing models. These algorithms adjust prices based on factors such as demand, competitor pricing, and historical sales data.
How it Works: ai analyzes market conditions and competitor pricing in real time to optimize product prices. For instance, during high demand, prices may increase, while they may drop during low demand periods.
Benefits: Dynamic pricing maximizes revenue and helps businesses remain competitive in a constantly changing market.
Visual Search
Use Case: E-commerce platforms like Pinterest and ASOS employ visual search powered by ai. Users can upload images or snapshots of products they like, and ai identifies similar items in the catalog.
How it Works: Computer vision ai algorithms analyze images and match them to visually similar products in the database.
Benefits: Visual search simplifies product discovery, reduces search time, and enhances the user experience, especially for fashion and home decor e-commerce.
Fraud Detection and Prevention
Use Case: E-commerce businesses use ai to detect and prevent fraudulent transactions. When a user makes a payment, ai assesses the transaction for suspicious behavior and flags potential fraud.
How it Works: ai algorithms analyze transaction data, user behavior, and historical patterns to identify anomalies that may indicate fraud.
Benefits: ai-driven fraud detection reduces financial losses, protects customer data, and ensures secure online transactions.
Inventory Management and Demand Forecasting
Use Case: Retailers use ai to optimize inventory management and forecast demand. ai algorithms analyze historical sales data, seasonal trends, and external factors to determine optimal inventory levels.
How it Works: ai models apply machine learning and data analysis techniques to predict future demand, reducing overstocking or understocking issues.
Benefits: Improved inventory management reduces costs, minimizes product waste, and ensures products are available when customers want them.
These real-life examples demonstrate the diverse ways in which ai is integrated into e-commerce, enhancing customer experiences, optimizing operations, and driving business growth. ai’s adaptability and ability to process vast amounts of data continue to revolutionize the e-commerce landscape.
Crafting Personalized Marketing Copy: A Thorough, Step-by-Step Handbook
Incorporating Large Language Models (LLMs) for personalized marketing copy in e-commerce necessitates a structured approach. This step-by-step guide outlines the fundamental implementation process.
Library Importation
Initiate the project by importing essential Python libraries tailored for your LLM API integration. Common choices include libraries for API interfacing, text data manipulation, and utility functions. For instance:
import openai # Import the library for GPT-3 or the relevant LLM API
import pandas as pd # For data manipulation if needed
API Authentication
Authentication is pivotal for accessing the LLM’s API. Acquire an API key or credentials from your LLM provider (e.g., OpenAI) to establish a secure connection. This API key binds your project to the LLM service, enabling seamless communication. Here’s an example:
api_key = "your_api_key_here"
openai.api_key = api_key
Data Preparation
Effective marketing copy relies on well-prepared data. Prepare the input data that will serve as the foundation for generating tailored marketing content. This data may encompass product details, customer profiles, or other pertinent information. For instance:
product_description = "Introducing our latest smartphone, designed for tech enthusiasts."
customer_name = "John Doe"
Marketing Copy Generation
Leverage the power of LLMs to create personalized marketing copy based on your input data. Construct prompts or messages that initiate the content generation process. Fine-tune parameters such as length and quantity as needed. Example code snippet:
prompt = f"Create a marketing message for {customer_name} about our new smartphone: '{product_description}'"
response = openai.Completion.create(
engine="text-davinci-002",
prompt=prompt,
max_tokens=50, # Adjust the desired length of the generated copy
n=1 # Number of responses to generate
)
Result Extraction and Display
Extract the generated marketing copy from the API response and prepare it for integration into your e-commerce platform. This content will serve as personalized marketing material. An example snippet:
generated_copy = response.choices(0).text.strip()
print(f"Generated Marketing Copy: {generated_copy}")
Integration and Iteration
Seamlessly incorporate the personalized marketing copy into your e-commerce ecosystem. Monitor the performance of the generated content and iterate as necessary for ongoing enhancement. Consider retraining the LLM with fresh data or prompts to further refine future marketing content.
Output
Generated Marketing Copy:
"Introducing our latest smartphone, designed for tech enthusiasts. Experience innovation like never before with our cutting-edge technology. Get yours today and stay ahead of the curve. #TechEvolution #Smartphone"
This is an example of the marketing copy generated by the Large Language Model (LLM) based on the input data and prompt. The output can vary based on the specific LLM you’re using, the input data, and the prompt provided.
This comprehensive guide lays the foundation for harnessing LLMs to elevate your e-commerce marketing strategy, delivering tailored and engaging content to your audience. Adapt these steps to your specific requirements and the LLM of your choice for optimal results.
<h2 class="wp-block-heading" id="h-future-considerations-in-e-commerce-with-generative-ai“>Future Considerations in E-commerce with Generative ai
As e-commerce continues to evolve, it’s crucial for businesses to consider the future landscape of generative ai and its potential impact on their operations. Here are key points to consider:
<h4 class="wp-block-heading" id="h-the-evolving-landscape-of-generative-ai“>The Evolving Landscape of Generative ai
Advancements in ai: The field of generative ai is rapidly advancing. New models, techniques, and applications are emerging regularly. To stay competitive, businesses should dedicate resources to keeping abreast of these developments. This might involve participating in ai research communities, attending conferences, or collaborating with ai specialists.
Research and Development: Consider establishing an internal research and development team or partnering with ai research organizations. Investing in ai innovation can help you explore how emerging ai models can benefit your e-commerce business. This includes identifying opportunities for automation, personalization, and efficiency improvements.
Integration Opportunities: Generative ai is not a one-size-fits-all solution. It can be integrated into various aspects of your e-commerce ecosystem, from customer support and content creation to supply chain management and pricing optimization. Continuously assess how generative ai can provide value beyond its current applications. Explore novel use cases that align with your business goals.
Unlocking E-Commerce Success: Real-World Case Studies of LLM Implementation
- Amazon: They use natural language processing (NLP) models to improve their product recommendations. When you see “Customers who bought this also bought…” or “Frequently bought together,” those suggestions are generated by LLMs analyzing the vast amount of customer data.
- Netflix: While primarily a streaming service, Netflix has a significant e-commerce component in the form of merchandise sales and subscription upgrades. They have used LLMs to enhance their personalized content recommendations, which has contributed to increased user engagement and revenue.
- Shopify: Shopify, a leading e-commerce platform, has incorporated LLMs into their chatbot and customer support systems. These chatbots can understand and respond to customer queries more naturally, leading to improved customer satisfaction and increased sales for businesses using their platform.
- Sephora: The cosmetics giant Sephora implemented LLMs in their mobile app. Customers can use natural language to describe the type of product they’re looking for, and the app provides personalized product recommendations and tips. This has not only increased user engagement but also boosted sales and customer loyalty.
- Etsy: Etsy, a platform for unique and handmade goods, uses LLMs to improve search functionality. Their algorithms understand user queries in natural language and provide relevant search results. This has led to a significant improvement in the user experience, increased sales for sellers, and higher customer retention rates.
- Walmart: Walmart has been using LLMs to optimize its supply chain and inventory management. These models help predict demand more accurately, ensuring that products are in stock when customers want them. This has led to increased sales and reduced carrying costs for the company.
These real-world examples illustrate how e-commerce businesses of varying sizes and niches have successfully implemented LLMs to enhance their operations, improve customer experiences, and drive revenue growth.
Potential Impact on E-Commerce
Enhanced Personalisation
Generative ai is poised to play a pivotal role in personalising customer experiences. Future ai models may possess the ability to predict user preferences and behaviours with unparalleled accuracy. This will lead to hyper-personalised product recommendations, tailored content, and marketing strategies that resonate deeply with individual customers.
Customer Engagement
Expect generative ai to further elevate customer engagement. ai-driven chatbots, virtual shopping assistants, and interactive experiences may become even more sophisticated. These technologies could simulate in-store shopping experiences, guide customers through complex purchasing decisions, and offer real-time support.
Dynamic Pricing
Explore the potential for generative ai to revolutionize pricing strategies. Future ai systems may optimize pricing dynamically based on real-time market conditions, demand fluctuations, competitor pricing, and even individual customer data. This can help maximize revenue and profitability.
Content Generation
While ai-generated content is already valuable for product descriptions, it may extend to content marketing, email campaigns, and social media management. Future ai models could write compelling blog posts, craft persuasive ad copy, and engage with customers on social platforms in a more human-like manner.
Supply Chain Optimization
Generative ai can contribute to enhanced supply chain management. This includes advanced demand forecasting, inventory optimization, logistics efficiency, and order fulfillment automation. These improvements can lead to cost savings and better customer service.
Visual Search
Explore the integration of ai models for visual search capabilities. In the future, customers may search for products by submitting images or photos, and ai algorithms will accurately identify and recommend matching products.
Customer Insights
Harness the power of generative ai to gain deeper insights into customer behaviors and preferences. Advanced analytics and ai-driven insights can inform marketing strategies, product development, and inventory decisions.
<h4 class="wp-block-heading" id="h-ethical-ai-practices”>Ethical ai Practices
As ai technologies evolve, ethical considerations remain paramount. Implement and uphold ethical guidelines for ai use. Ensure responsible handling of customer data and transparency in ai-driven decision-making processes.
Competition and Market Trends
Monitor how your competitors adopt generative ai in their e-commerce strategies. Keep a close watch on industry trends influenced by ai-driven experiences. Stay agile and responsive to shifts in consumer preferences shaped by ai technologies.
Conclusion
In conclusion, the integration of Large Language Models (LLMs) in e-commerce marketing has ushered in a new era of personalized customer experiences and efficient marketing strategies. These advanced ai-driven tools have empowered businesses to engage with their customers more effectively, offering tailored recommendations, enhancing customer support, and streamlining content generation. As a result, customer satisfaction and loyalty have seen significant boosts.
However, this transformative journey is not without its challenges. The responsible and ethical use of LLMs is paramount, with considerations like potential biases and data privacy at the forefront. Additionally, maintaining the quality and relevance of content generated by these models requires ongoing monitoring and adjustments. As we look ahead, the evolving landscape of generative ai promises to have even more profound impacts on e-commerce, and businesses that embrace these innovations will position themselves best to thrive in this ever-changing digital landscape.
Key Takeaways
- Revolutionise large language models (LLMs) like GPT-3 and GPT-4 which has e-commerce marketing by enabling personal customer interactions, precise product recommendations, and efficient customer support.
- LLMs empower e-commerce platforms to generate high-quality marketing content, improving customer engagement and conversion rates while saving time and resources.
- Challenges such as potential biases and ethical considerations require vigilance in LLM implementation, emphasising the responsible use of ai in e-commerce.
- Implementing and scaling LLMs in e-commerce demands careful planning and consideration of customer data privacy and ethical use.
- Real-life examples showcase how LLMs enhance e-commerce marketing, offering valuable insights into their practical applications.
- As generative ai continues to evolve, its impact on e-commerce will grow, presenting exciting opportunities for businesses that adapt to this transformative technology.
Frequently Asked Questions
A. ai systems like Large Language Models (LLMs) have undergone training on vast amounts of text data, allowing them to comprehend and produce human-like text. In e-commerce marketing, LLMs provide several advantages, including elevating customer interaction through chatbots, enhancing product recommendations grounded in customer preferences, automating customer support effectively, employing advanced natural language processing in search, and crafting marketing materials content. They empower businesses to personalise marketing endeavours, boost customer engagement, and optimise multiple facets of their operations.
A. Certainly! LLMs power chatbots and virtual assistants that engage with customers in real-time, answer queries, and provide personalised product recommendations. For instance, an LLM can suggest products from customer’s past purchases and browsing behaviour, leading to more relevant product suggestions. This level of personalisation enhances the customer experience and increases satisfaction, ultimately driving sales.
A. Implementing LLMs in e-commerce comes with challenges such as potential biases in responses and ethical concerns like data privacy and misuse. LLMs learn from vast datasets, which may contain societal biases, so careful monitoring and attention must ensure fairness and avoid discriminatory outcomes. Additionally, businesses must handle customer data responsibly and ensure the ethical use of ai technology.
The media shown in this article is not owned by Analytics Vidhya and is used at the Author’s discretion.