Three-dimensional (3D) modeling has become critical in various fields, such as architecture and engineering. 3D models are computer-generated objects or environments that can be manipulated, animated, and rendered from different perspectives to provide a realistic visual representation of the physical world. Creating 3D models can be time consuming and expensive, especially for complex objects. However, recent advances in computer vision and machine learning have made it possible to generate 3D models or scenes from a single input image.
3D scene generation involves the use of artificial intelligence algorithms to learn the underlying structure and geometric properties of an object or environment from a single image. The process typically consists of two stages: the first is to extract the shape and structure of the object, and the second is to generate the texture and appearance of the object.
In recent years, this technology has become a hot topic in the research community. The classical approach to 3D scene generation involves learning the functions or characteristics of a scene rendered in two dimensions. In contrast, novel approaches take advantage of differentiable rendering, which allows the computation of gradients or derivatives of rendered images with respect to the input geometric parameters.
However, all of these techniques, often developed to address this task for specific categories of objects, provide 3D scenes with limited variations, such as minorly changed terrain renderings.
A novel approach to 3D scene generation has been proposed to address this limitation.
His goal is to create natural scenes that possess unique characteristics resulting from the interdependence between their constituent geometry and appearance. The distinctive nature of these features makes it difficult for the model to learn the features of common figures.
In similar cases, the instance-based paradigm is employed, which involves manipulating a suitable instance model to build a richer target model. Therefore, the exemplary model must have similar characteristics to the target model for this technique to be effective.
However, having different exemplary scenes with specific characteristics makes it difficult to have ad hoc designs for each type of scene.
Therefore, the proposed approach uses a patch-based algorithm, which was used long before deep learning. The pipeline is presented in the following figure.
Specifically, a multi-scale patch-based generative framework is adopted, employing a Generative Patch Nearest-Neighbor (GPNN) module to maximize bidirectional visual summary between input and output.
This approach uses Plenoxels, a grid-based radiation field known for its impressive visual effects, to render the input scene. While its regular structure and simplicity benefit patch-based algorithms, certain essential designs must be implemented. Specifically, the exemplary pyramid is built through a coarse-to-fine Plenoxels training process on images of the input scene rather than simply downsampling a high-resolution pretrained model. In addition, the high-dimensional, boundless, and noisy features of the Plenoxels-based model at each level are transformed into well-defined, compact appearance and geometric features to improve robustness and efficiency in subsequent patch matching.
Furthermore, this study employs various representations for the synthesis process within the generative nearest neighbor module. Mixing and patching operate simultaneously on each level to progressively synthesize a scene based on intermediate values, eventually morphing into a coordinate-based equivalent.
Finally, the use of patch-based algorithms with voxels can lead to high computational demands. Therefore, an exact-to-approximate patch nearest neighbor field (NNF) modulus is used in the pyramid, which keeps the search space within a manageable range while making minimal compromises in optimizing the visual summary.
The results obtained by this model are reported below for some random images.
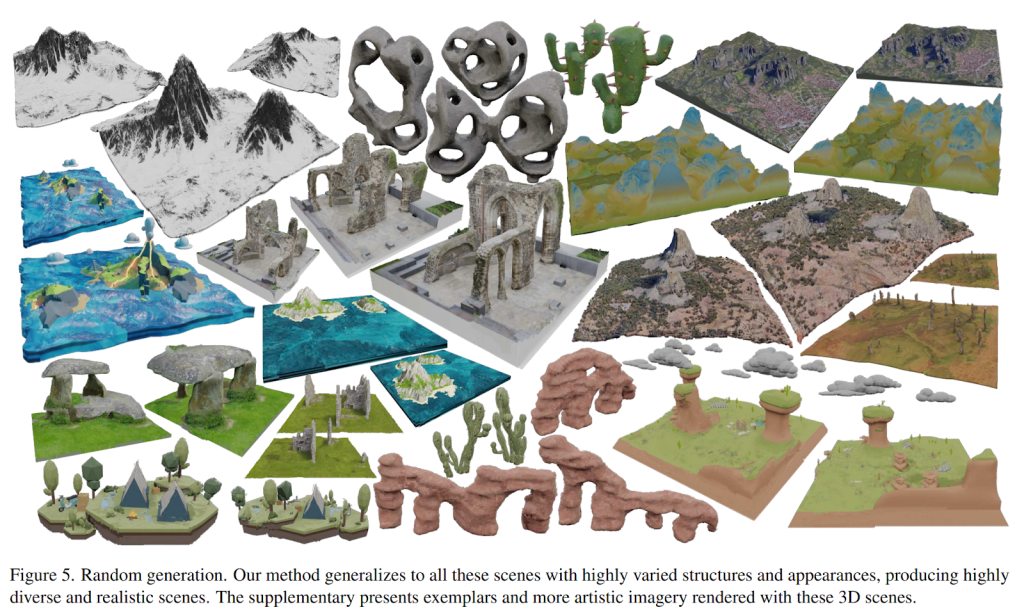
This was the brief for a new AI framework to enable imaging to high variation 3D scenes. If you are interested, you can learn more about this technique at the links below.
review the Paper and Project. Don’t forget to join our 21k+ ML SubReddit, discord channel, and electronic newsletter, where we share the latest AI research news, exciting AI projects, and more. If you have any questions about the article above or if we missed anything, feel free to email us at [email protected]
🚀 Check out 100 AI tools at AI Tools Club
Daniele Lorenzi received his M.Sc. in ICT for Internet and Multimedia Engineering in 2021 from the University of Padua, Italy. He is a Ph.D. candidate at the Institute of Information Technology (ITEC) at the Alpen-Adria-Universität (AAU) Klagenfurt. He currently works at the Christian Doppler ATHENA Laboratory and his research interests include adaptive video streaming, immersive media, machine learning and QoS / QoE evaluation.