Self-supervised learning (SSL) has expanded the reach of speech technologies to many languages by minimizing the need for labeled data. However, current models only support 100-150 of the world’s 7,000+ languages. This limitation is largely due to the scarcity of transcribed speech, as only half of these languages have formal writing systems, and even fewer have the resources to generate the extensive annotated data needed for training. While SSL models can operate on unlabeled data, they typically cover a narrow range of languages. Projects like MMS have expanded coverage to over 1,000 languages, but need help with data noise and a lack of diverse recording conditions.
Researchers from Carnegie Mellon University, Shanghai Jiaotong University, and Toyota Institute of technology in Chicago have developed XEUS, a universal multilingual speech encoder. XEUS is trained on over one million hours of data from 4,057 languages, significantly increasing the language coverage of SSL models. This includes a new corpus of 7,413 hours from 4,057 languages, which will be published soon. XEUS incorporates a novel dereverberation objective for increased robustness. It outperforms state-of-the-art models on multiple benchmarks, including ML-SUPERB. To support future research, the researchers will publish XEUS, its code, training configurations, checkpoints, and training logs.
SSL has advanced speech processing by allowing neural networks to learn from large amounts of unlabeled data, which can then be fine-tuned for a variety of tasks. Multilingual SSL models can leverage cross-language transfer learning, but can only scale to cover a few languages. XEUS, however, can scale to 4057 languages, outperforming models such as Meta’s MMS. XEUS includes a novel dereverberation target during training to handle noisy and diverse speech. Unlike state-of-the-art models that often use closed datasets and lack transparency, XEUS is completely open, with publicly available data, training code, and extensive documentation, facilitating further research into large-scale multilingual SSL.
XEUS is pre-trained on a vast dataset of 1.081 million hours across 4,057 languages, compiled from 37 public speech datasets and additional sources such as the Global Recordings Network, WikiTongues, and Jesus Dramas. Unique data types improve its robustness, such as accented speech and code-switching. XEUS incorporates new targets, including dereverberation and noise reduction, during training. The model architecture is based on HuBERT but includes enhancements such as E-Branchformer layers and a simplified loss function. Training on 64 NVIDIA A100 GPUs uses advanced augmentation techniques and covers significantly more data than previous models.
The XEUS model is evaluated on several downstream tasks to assess its acoustic and multilingual representation capabilities. It excels at multilingual speech tasks, outperforming state-of-the-art models such as XLS-R, MMS, and w2v-BERT on benchmarks like ML-SUPERB and FLEURS, especially in low-resource language environments. Furthermore, XEUS demonstrates strong performance in task universality by matching or outperforming leading models on English-only tasks like emotion recognition and speaker diarization. In acoustic representation, XEUS outperforms models like WavLM and w2v-BERT in generating high-quality speech, evidenced through metrics like MOS and WER.
XEUS is a robust SSL speech coder trained on over 1 million hours of data spanning 4057 languages, demonstrating superior performance on a wide range of multilingual and low-resource tasks. XEUS’ dereverberation task improves its robustness, and despite limited data for many languages, it still provides valuable results. XEUS promotes multilingual research by offering open access to its data and models. However, ethical considerations are crucial, especially in handling voice data from indigenous communities and preventing misuses such as generating audio deepfakes. Integrating XEUS with accessible platforms aims to democratize voice model development.
Review the Paper, Data set, and ModelAll credit for this research goes to the researchers of this project. Also, don't forget to follow us on twitter.com/Marktechpost”>twitter.
Join our Telegram Channel and LinkedIn GrAbove!.
If you like our work, you will love our Newsletter..
Don't forget to join our Subreddit with over 46 billion users
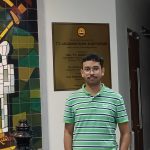
Sana Hassan, a Consulting Intern at Marktechpost and a dual degree student at IIT Madras, is passionate about applying technology and ai to address real-world challenges. With a keen interest in solving practical problems, she brings a fresh perspective to the intersection of ai and real-life solutions.
<script async src="//platform.twitter.com/widgets.js” charset=”utf-8″>