Quality of Service (QoS) is a very important metric used to evaluate the performance of network services in mobile edge environments where mobile devices frequently request services from edge servers. It includes dimensions such as bandwidth, latency, jitter, and data packet loss rate. However, most current QoS datasets, such as the WS-Dream dataset, mainly focus on static QoS metrics and ignore factors such as geographic location and temporal data. These dynamic attributes, which capture the location of the mobile device at the time of service requests and the sequence of those requests, are not currently being fully utilized. These factors are essential to accurately predict network performance, as QoS often varies with changes in location and time.
Current methods for QoS prediction use collaborative filtering, which relies on user historical data to predict missing QoS values based on similarities. These approaches often need help with data sparsity, which limits their ability to generate accurate predictions. During this, essential temporal and spatial variations are ignored. Deep learning-based methods have also been introduced, using models such as neighborhood-based learning and user-service graphs or to improve prediction accuracy. These methods still need to be revised to adapt to the changing conditions and diverse user behaviors characteristic of mobile edge environments. Existing data sets such as WS-Dream, which focuses on static QoS metrics, fail to capture time-specific and location-based fluctuations in in-service performance. To address this, the CHESTNUT dataset was developed, which offers a customized solution for mobile edge environments by incorporating attributes such as user mobility, server resource load, and real-time geographic data.
A group of researchers from Shanghai University has proposed CHESTNUTwhich improves QoS prediction by incorporating key factors such as edge server load, user mobility and service diversity, critical elements for accurately modeling complex interactions in mobile edge environments. To build CHESTNUT, the researchers used two real-world data sets from Shanghai: the Johnson Taxi GPS data set to simulate user mobility and the Shanghai Telecom data set to represent the locations of edge servers. After preprocessing, these data sets provided a realistic view of user and edge server behavior. CHESTNUT also includes network-specific metrics such as response time and network jitter, which are affected by the distance, speed, and server bandwidth usage between the user and the server. This dataset offers temporal and spatial details, enabling more accurate and context-sensitive QoS predictions and capturing real-world dynamics. It also introduces resource-based attributes, such as processing and queuing delays, which are influenced by user demand and server capabilities. This granular data enables detailed analysis of service outages, quality fluctuations, and network stability, providing a solid foundation for QoS prediction models that can respond to the changing demands of mobile edge computing applications. , providing a richer and more realistic basis for QoS prediction. , allowing researchers to create more accurate and responsive models suited to the ever-evolving demands of edge computing.
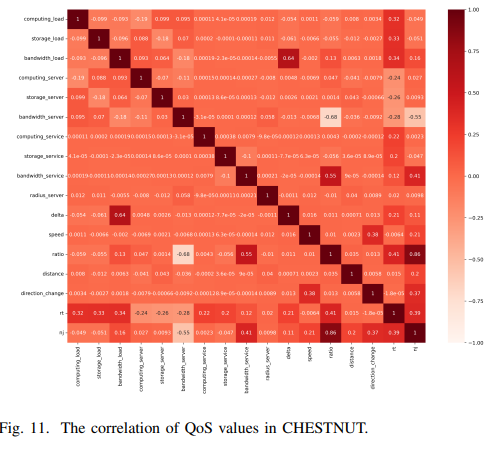
In conclusion, the CHESTNUT dataset advances QoS prediction for mobile edge environments by including dynamic temporal and geographic information. This comprehensive approach aims to support more accurate and efficient QoS prediction models, addressing the gaps left by traditional data sets to adapt to the demands of mobile edge computing. He concluded that the response time is proportional to the load and service resource demands of the edge servers, while it is inversely proportional to the total resources of the edge servers. The CHESTNUT dataset is accurate and reliable data to support future QoS prediction in mobile edge environments.
look at the Paper. All credit for this research goes to the researchers of this project. Also, don't forget to follow us on twitter.com/Marktechpost”>twitter and join our Telegram channel and LinkedIn Grabove. If you like our work, you will love our information sheet.. Don't forget to join our SubReddit over 55,000ml.
(Trend) LLMWare Introduces Model Depot: An Extensive Collection of Small Language Models (SLM) for Intel PCs
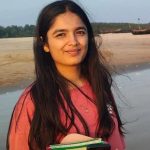
Nazmi Syed is a consulting intern at MarktechPost and is pursuing a bachelor of science degree at the Indian Institute of technology (IIT) Kharagpur. He has a deep passion for data science and is actively exploring the broad applications of artificial intelligence in various industries. Fascinated by technological advancements, Nazmi is committed to understanding and implementing cutting-edge innovations in real-world contexts.
<script async src="//platform.twitter.com/widgets.js” charset=”utf-8″>