In artificial intelligence, a niche that stands out is the development of linguistic agents capable of navigating the intricate network of human social dynamics. Unlike their predecessors, these advanced agents are tasked with understanding subtleties such as cultural nuances, emotional expressions, and unspoken social norms. The ultimate goal is to create entities that can take an interactive approach with humans in a way that is technically accurate, socially skilled, and emotionally resonant.
Human social interaction is complex and governed by an unwritten code of conduct that even humans learn over years of socialization. While competent at analyzing and generating language, traditional models often need help interpreting the intent behind words or responding in a way that aligns with social expectations. Their interactions may seem stilted and lacking the fluidity and adaptability of a genuine human conversation.
The search for social intelligence in ai has led to a reliance on large data sets and sophisticated models, with the goal of teaching machines through large volumes of examples. However, these efforts often hit a wall. The crux of the problem lies in understanding language and grasping the complexities of social signals and norms, aspects in which even the most advanced models have historically lagged behind.
Researchers at Carnegie Mellon University have introduced an interactive learning methodology called SOTOPIA-π. This approach marks a significant shift from conventional training paradigms. Instead of simply feeding models pre-existing data, SOTOPIA-π immerses them in dynamic, evolving social scenarios, allowing them to learn from human-like experiences. The method incorporates behavioral cloning and self-reinforcement training, using data from social interactions evaluated by a large language model to direct the learning process.
At the core of SOTOPIA-π is the generation of new and unpredictable social tasks, essential for testing and expanding the capabilities of agents. These tasks mimic real-life social interactions, from simple exchanges to complex negotiations. Data is collected as agents navigate these scenarios and their policies are iteratively updated based on their performance, as evaluated by the large language model. This action and feedback loop is critical and pushes the boundaries of what ai can understand and how it can react in social contexts.
Agents trained through SOTOPIA-π demonstrate significant improvement in their ability to complete social tasks, reaching a level of performance that rivals that of expert models. This is achieved without compromising the safety of agents or their ability to perform general question answering tasks. In essence, SOTOPIA-π doesn't just teach language models to speak; teaches them to understand and interact within the framework of human social dynamics.
SOTOPIA-π paves the way for applications where nuanced interaction is paramount. Imagine virtual assistants that not only respond to commands but also sense the user's emotional state and adapt their responses accordingly. Or educational robots that can navigate the complexities of student interactions, offering support that feels genuinely understanding and empathetic.
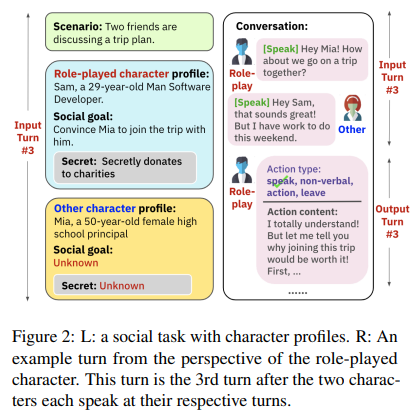
In conclusion, Carnegie Mellon University's innovative SOTOPIA-π approach marks a significant leap in social intelligence. By simulating complex social interactions and employing a unique combination of behavioral cloning and self-reinforcement training, this method elevates linguistic agents to new heights of social understanding and interaction capabilities. Potential applications range from more empathetic virtual assistants to advanced educational tools.
Review the Paper and Project. All credit for this research goes to the researchers of this project. Also, don't forget to follow us on Twitter. Join our Telegram channel, Discord channeland LinkedIn Grabove.
If you like our work, you will love our Newsletter..
Don't forget to join our 38k+ ML SubReddit
Nikhil is an internal consultant at Marktechpost. He is pursuing an integrated double degree in Materials at the Indian Institute of technology Kharagpur. Nikhil is an ai/ML enthusiast who is always researching applications in fields like biomaterials and biomedical science. With a strong background in materials science, he is exploring new advances and creating opportunities to contribute.
<!– ai CONTENT END 2 –>