Foundation models have emerged as transformative digital technologies, introducing new capabilities and risks that have captured unprecedented public attention. However, the current basic model ecosystem lacks transparency, reflecting the problems faced by previous digital technologies such as social media platforms. The 2023 Foundation Model Transparency Index revealed that top developers scored an average of just 37 out of 100 points in transparency. This opacity presents significant challenges to understanding and governing these powerful ai systems. As foundation models continue to evolve and impact society, there is a growing need for comprehensive and standardized transparency practices. Governments around the world are beginning to address this issue through various legislative and regulatory initiatives, with the goal of requiring public reporting and increasing accountability in the ai industry.
Existing attempts to address transparency challenges in ai have primarily focused on evaluations of models and documentation frameworks. Model evaluations aim to clarify strengths and weaknesses, but often lack a broader social context. Documentation approaches such as data sheets and model cards provide more complete information by asking open-ended questions about data set creation, model development, and their limitations. Ecosystem cards have been introduced specifically for core models, emphasizing the importance of tracking relationships between data sets, models, and applications.
These methods, however, face limitations in standardization and completeness. For example, the Llama 2 model card, while addressing many high-level categories, omits several lower-level questions from the original model card framework. In addition to this, reproducibility checklists required by ai conferences have attempted to enforce some transparency standards. Despite these efforts, the current ai transparency landscape remains fragmented and inconsistent, highlighting the need for a more structured and comprehensive approach to core model transparency reporting.
Researchers from Stanford University, the Massachusetts Institute of technology and Princeton University propose Foundation model transparency Reports, which offer a structured approach to address transparency challenges in the ai industry. These reports are designed to be published periodically by core model developers and provide essential information in a standardized format. This method builds on the recommendations of the G7 voluntary code of conduct and the White House's voluntary commitments, while incorporating the 100 transparency indicators defined in the Foundation's Model Transparency Index.
The proposed approach aims to consolidate crucial information, making it easily accessible to stakeholders and facilitating analysis and comparison between different developers. Transparency reports go beyond current government policies by specifying a precise scheme for information disclosure, covering the entire supply chain of foundation models. By implementing these reporting practices, developers can establish higher transparency standards across the ai ecosystem, which could improve compliance with multiple jurisdictions and reduce overall compliance burden. The methodology also includes examples of reporting entries based on publicly available information, setting a clear precedent for future transparency efforts in the foundation modeling industry.
Foundation model transparency reports are designed based on six key principles derived from the strengths and weaknesses of social media transparency reports. These principles aim to create a more comprehensive and standardized approach to transparency in the ai industry. The first three principles build on the strengths of existing social media transparency reports: (1) Consolidation of information in a centralized location, providing stakeholders with a single, predictable source of relevant data. (2) Structured reports that address specific queries, typically organized into four top-level sections, setting clear expectations for the content of the report. (3) Extensive contextualization of information to ensure appropriate interpretation by various stakeholders with different levels of experience.
The remaining three principles address the shortcomings of current social media transparency practices: (4) Independent specification of the information to be included, avoiding selective reporting by the platforms. (5) Total standardization of both form and content, allowing easy comparison and aggregation of data between different platforms. (6) Clear specification of methodologies for calculating statistics to avoid misinterpretations and ensure consistency in reporting. These principles aim to create a more robust and meaningful transparency framework for foundation models.
Based on these principles, the Foundation Model Transparency Reports incorporate indicators derived from the Foundation Model Transparency Index. This approach ensures comprehensive coverage of the base model ecosystem, addressing various aspects of the supply chain. The reports are designed to provide specific, standardized information that allows meaningful comparisons between different developers and models.
The structure of these reports is carefully designed to balance detail with accessibility. They typically include sections that cover key areas such as model development, training data, model architecture, performance metrics, and deployment practices. Each section contains clearly defined indicators that developers must report on, ensuring consistency and comparability.
To facilitate implementation, the methodology includes examples of how developers can report information related to these indicators. These examples serve as templates and demonstrate the level of detail and formatting expected in reports. By providing such guidance, the Framework Model Transparency Reports aim to establish a uniform standard for transparency in the ai industry, making it easier for stakeholders to access, interpret and analyze crucial information about core models.
Foundation Model Transparency Reports are designed to align with existing and emerging government policies, facilitating compliance in different jurisdictions. The methodology tracks six major policies, including the EU ai Law and the US Executive Order on ai, and relates the report's indicators to specific requirements within these regulations.
This alignment serves multiple purposes. First, it incentivizes foundation model developers to adopt the transparency reporting framework, as much of the information disclosed will also satisfy legal requirements. Second, it provides a clear picture of how different jurisdictions prioritize various aspects of ai transparency, highlighting potential gaps or overlaps in regulatory approaches.
However, the analysis reveals a relatively low level of alignment between current government policies and the comprehensive set of indicators proposed in the transparency reports. This discrepancy underscores the lack of granularity in many government transparency requirements for ai. By offering a more detailed and standardized reporting structure, the Foundation's Model Transparency Reports aim to not only meet but exceed current regulatory standards, which could influence future policy development in the field of transparency governance. ai.
To illustrate the practical implementation of Foundation Model Transparency Reports, researchers constructed example entries from the practices of nine leading foundation model developers. This approach was necessitated by current lackluster transparency practices across the industry, as revealed by the 2023 Foundation Model Transparency Index (FMTI).
The sample report focuses on 82 out of 100 indicators where at least one developer demonstrated some level of transparency. For each indicator, the researchers selected the developer whose practices best exemplified transparency, resulting in a composite report showing a variety of best practices in different aspects of the development and implementation of the basic model.
This exercise revealed several key insights:
1. There are still 18 indicators for which no major developer currently provides transparent information, particularly in areas related to labor and usage statistics.
2. Even for the 82 indicators with some level of disclosure, there is significant room for improvement in terms of contextualization and methodological clarity.
3. The lack of a common conceptual framework among developers creates inconsistencies in the way information is reported, particularly regarding data channels and labor participation.
4. For many indicators, it remains unclear whether the information disclosed is complete or partial.
These findings underscore the need for more standardized and comprehensive transparency practices across the core model ecosystem, highlighting areas where developers can set meaningful precedents and improve their reporting methodologies.
Transparency in foundation model development serves multiple crucial functions, from improving public accountability to improving risk management. As the field evolves, it becomes increasingly important to establish strong regulations and industry standards for transparency. Different aspects of transparency serve specific societal objectives and stakeholder groups. Transparency in data, work practices, IT usage, assessments and usage statistics directly informs understanding of model biases, working conditions, development costs, capabilities, risks and impact economic. By fostering a culture of openness, the ai community can collectively address challenges, improve understanding, and ultimately improve the societal impact of core models.
look at the Paper. All credit for this research goes to the researchers of this project. Also, don't forget to follow us on twitter.com/Marktechpost”>twitter and join our Telegram channel and LinkedIn Grabove. If you like our work, you will love our information sheet..
Don't forget to join our 52k+ ML SubReddit
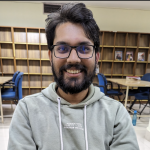
Asjad is an internal consultant at Marktechpost. He is pursuing B.tech in Mechanical Engineering from Indian Institute of technology, Kharagpur. Asjad is a machine learning and deep learning enthusiast who is always researching applications of machine learning in healthcare.
<script async src="//platform.twitter.com/widgets.js” charset=”utf-8″>