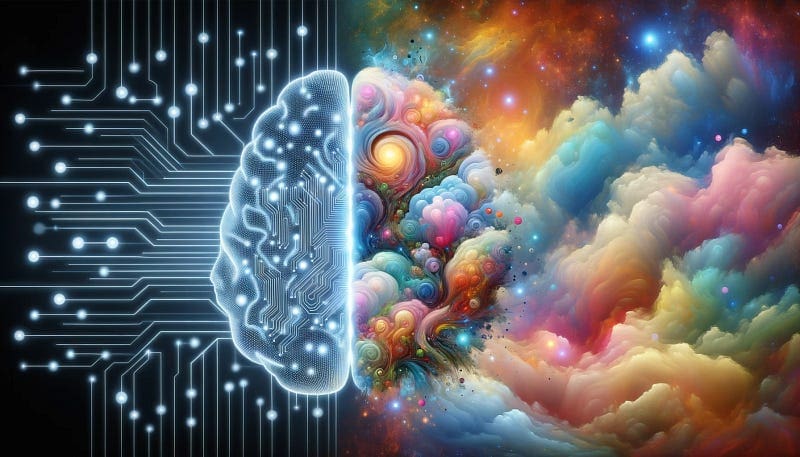
Image generated by DALL-E
In the digital age, the wonders of artificial intelligence have transformed the way we interact, work and even think.
From voice assistants curating our playlists to predictive algorithms forecasting market trends, ai has been seamlessly integrated into our daily lives.
But as with any technological advance, it is not without twists.
A large language model or LLM is a trained machine learning model that generates text based on the message you provided. To generate good responses, models take advantage of all the knowledge retained during their training phase.
Recently, LLMs have demonstrated impressive and growing capabilities, including generating compelling responses to any type of user prompts.
However, although LLMs have an incredible ability to generate text, it is difficult to know whether this generation is accurate or not.
And this is precisely what is commonly known as hallucinations.
But what are these hallucinations and how do they affect the reliability and usefulness of ai?
LLMs are geniuses when it comes to text generation, translations, creative content and more.
Despite being powerful tools, the LLM has some important deficiencies:
- The decoding techniques used can produce results that are boring, lacking coherence or prone to monotonous repetition.
- Its knowledge base is “static” in nature and presents challenges in fluid updates.
- A common problem is the generation of meaningless or inaccurate text.
The last point is known as hallucination, which is a concept extended by ai to humans.
For humans, hallucinations represent experiences perceived as real despite being imaginary. This concept extends to ai models, where hallucinated text appears accurate even if it is fake.
In the context of LLMs, “hallucination” refers to a phenomenon where the model generates incorrect, meaningless, or non-real text.
Image of Dall-E
LLMs are not designed as databases or search engines, so they do not refer to specific sources or knowledge in their answers.
I bet most of you are wondering… How can this be possible?
Well… these models produce text based on the given message. The generated response is not always directly supported by specific training data, but is instead designed to align with the context of the message.
In simpler terms:
They can confidently spout information that is factually incorrect or simply doesn’t make sense.
Identifying hallucinations in humans has always posed a significant challenge. This task becomes even more complex given our limited ability to access a reliable baseline for comparison.
While detailed insights, such as the output probability distributions of large language models, can help in this process, such data is not always available, adding another layer of complexity.
The question of hallucination detection remains unresolved and is the subject of ongoing research.
- The blatant lies: LLMs can evoke events or figures that never existed.
- The too precise: They could share too much, which could lead to the spread of sensitive information.
- The absurd: Sometimes the result can be pure nonsense.
Why do these hallucinations occur?
The main cause lies in the training data. LLMs learn from vast sets of data, which can sometimes be incomplete, outdated, or even contradictory. This ambiguity can lead them astray, causing them to associate certain words or phrases with inaccurate concepts.
Additionally, the sheer volume of data means that LLMs may not have a clear “source of truth” to verify the information they generate.
Interestingly, these hallucinations can be a blessing in disguise. If you’re looking for creativity, you’ll want LLMs like ChatGPT to blow your mind.
Image generated by DALL-E
Imagine asking for a unique fantasy story plot; you will want a new narrative, not a replica of an existing one.
Similarly, during a brainstorm, hallucinations can offer a large number of diverse ideas.
Awareness is the first step in addressing these hallucinations. Here are some strategies to keep them under control:
- Consistency checks: Generate multiple responses to the same message and compare.
- Semantic similarity checks: Use tools like BERTScore to measure semantic similarity between generated texts.
- Training on updated data: Regularly update training data to ensure relevance. You can even tune the GPT model to improve its performance in some specific fields.
- User awareness: Educate users about possible hallucinations and the importance of cross-referencing information.
And last but not least… EXPLORE!
This article has laid the groundwork for LLM hallucinations, but the implications for you and your application may differ considerably.
Furthermore, your interpretation of these phenomena may not exactly correspond to reality. The key to fully understanding and appreciating the impact of LLM hallucinations on your endeavors is through an in-depth exploration of LLMs.
The journey of ai, especially LLMs, is akin to navigating uncharted waters. While the vast ocean of possibilities is exciting, it is essential to be wary of mirages that could lead us astray.
By understanding the nature of these hallucinations and implementing strategies to mitigate them, we can continue to harness the transformative power of ai, ensuring its accuracy and reliability in our ever-evolving digital landscape.
Joseph Ferrer He is an analytical engineer from Barcelona. He graduated in physical engineering and currently works in the field of Data Science applied to human mobility. He is a part-time content creator focused on data science and technology. You can contact him at LinkedIn, Twitter either Half.