The efficiency and retrieval capabilities of linguistic models are fundamental aspects that dictate their usefulness and effectiveness. As artificial intelligence delves deeper into the complexities of human language, the demand for models that can process large amounts of information with high precision and minimal resource consumption has never been more critical. This outlook sets the stage for innovative research that addresses these challenges head-on, presenting solutions that could revolutionize our interaction with technology.
Researchers from Stanford University, the University at Buffalo and Purdue University presented Based, an architecture that differs significantly from traditional approaches, which aims to bridge the gap between the dual objectives of improving recovery while ensuring efficiency. Unlike previous models that often found themselves in a balance between memory use and the ability to recall information accurately, Based emerges as a beacon of balance and versatility.
By integrating linear attention with sliding window attention, the architecture artfully navigates through the complex landscape of memory and efficiency. This hybrid model allows for dynamic adjustment based on the task at hand, effectively adapting its operating mode to mimic the expansive recovery capabilities of full attention models or operate within the limits of a reduced state size, similar to more efficient alternatives. In Memory. Such adaptability shows the architectural finesse of Based and its practical applicability across a spectrum of language processing tasks.
the shine of Based It extends beyond its conceptual design to its implementation, where IoT-aware algorithms play a fundamental role. These algorithms are developed specifically to improve performance on language generation tasks, a critical component that directly affects model performance and usefulness. Based achieves unparalleled efficiency through these optimizations, significantly outperforming established models like FlashAttention-2 in terms of performance. This leap in performance is not only a testament to the architectural innovation of Based but it also highlights the importance of algorithmic efficiency in the evolution of language models.
The empirical evaluation of Based further solidifies its position as an innovative advancement in the field. Through a series of rigorous tests, including perplexity measurements and intensive retrieval tasks, the architecture demonstrates its superiority over existing subquadratic models. Based matches, but occasionally exceeds, the retrieval capabilities of these models, marking an important milestone in the search for highly efficient yet capable language processing tools. These results highlight the potential of Based serve as the fundamental architecture for future language models, paving the way for more sophisticated and practical applications in artificial intelligence.
Beyond its technical achievements, the development of Based represents a broader shift in the landscape of natural language processing. It exemplifies the growing emphasis on creating models that are not only powerful but also resource-efficient, a crucial consideration in an era when the environmental impact of computing is increasingly scrutinized. Based sets a precedent for future research, illustrating the potential of hybrid architectures and optimized algorithms to overcome long-standing challenges.
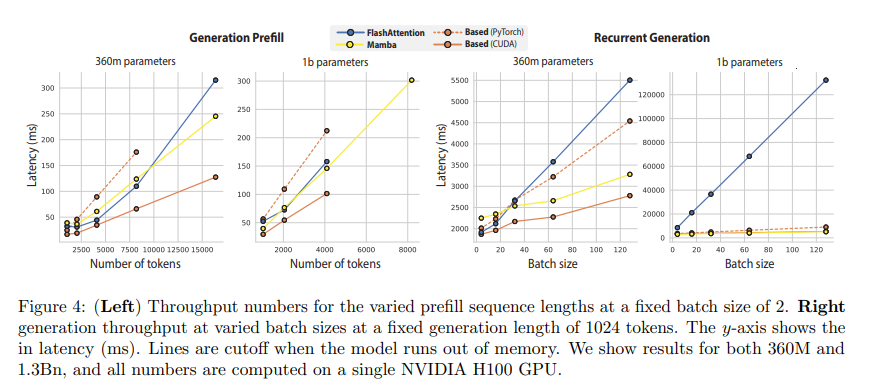
In conclusion, the introduction of Based It marks a crucial moment in the evolution of linguistic models, heralding a new era of efficiency and recovery capabilities. By artfully balancing these two critical aspects, Based Not only does it address a fundamental challenge in natural language processing, it also opens the door to a host of applications that were previously limited by the limitations of existing models. The impact of Based will resonate far beyond the boundaries of academic research and will influence the development of artificial intelligence technologies for years to come.
Review the Paper and GitHub. All credit for this research goes to the researchers of this project. Also, don't forget to follow us on Twitter and Google news. Join our 38k+ ML SubReddit, 41k+ Facebook community, Discord channeland LinkedIn Grabove.
If you like our work, you will love our Newsletter..
Don't forget to join our Telegram channel
You may also like our FREE ai Courses….
Hello, my name is Adnan Hassan. I'm a consulting intern at Marktechpost and soon to be a management trainee at American Express. I am currently pursuing a double degree from the Indian Institute of technology, Kharagpur. I am passionate about technology and I want to create new products that make a difference.
<!– ai CONTENT END 2 –>