Mobile apps are an integral part of daily life and serve countless purposes, from entertainment to productivity. However, the complexity and diversity of mobile user interfaces (UIs) often pose challenges in accessibility and usability. These interfaces are characterized by unique features, such as elongated aspect ratios and densely packed elements, including icons and text, that conventional models have difficulty interpreting accurately. This gap in technology underscores the pressing need for specialized models capable of deciphering the intricate landscape of mobile applications.
Existing research and methodologies in mobile UI understanding have introduced frameworks and models such as the RICO dataset, Pix2Struct, and ILuvUI, focusing on structural analysis and language vision modeling. CogAgent leverages screen images for UI navigation, while Spotlight applies vision and language models to mobile interfaces. Models like Ferret, Shikra, and Kosmos2 improve referencing and grounding capabilities, but are primarily aimed at natural images. MobileAgent and AppAgent employ MLLM for on-screen navigation, indicating a growing emphasis on intuitive interaction mechanisms despite their reliance on external modules or predefined actions.
Apple researchers have presented Ferret-UI, a model developed specifically to advance the understanding and interaction with mobile UIs. Distinguishing itself from existing models, Ferret-UI incorporates an “any resolution” capability, adapting to screen aspect ratios and focusing on fine details within user interface elements. This approach ensures a deeper and more nuanced understanding of mobile interfaces.
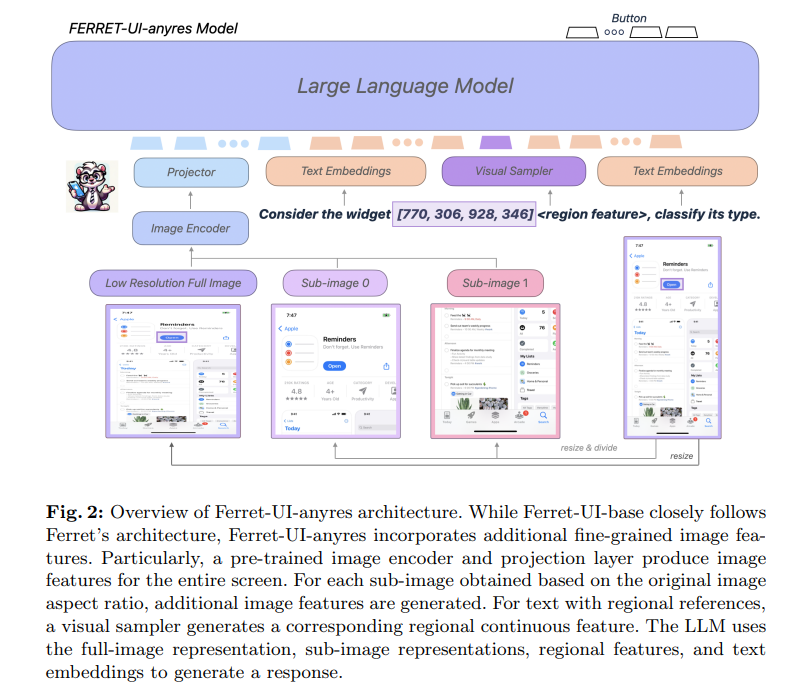
Ferret-UI's methodology revolves around adapting its architecture for mobile UI displays, using an “any resolution” strategy to handle various aspect ratios. The model processes the UI screens by dividing them into subimages, ensuring a detailed approach to the elements. The training includes the RICO dataset for Android and proprietary data for iPhone screens, covering elementary and advanced UI tasks. This includes widget classification, icon recognition, OCR, and grounding tasks such as finding widgets and finding icons, leveraging GPT-4 to generate advanced task data. Subimages are encoded separately, using visual features of varying granularity to enrich the model's understanding and interaction capabilities with mobile UIs.
Ferret-UI is more than a promising model; He is a proven artist. It outperformed open source front-end MLLMs and GPT-4V, showing a significant jump in performance on specific tasks. On icon recognition tasks, Ferret-UI achieved an accuracy rate of 95%, a substantial 25% increase over the closest competing model. It achieved a 90% success rate in widget classification, outperforming GPT-4V by 30%. Grounding tasks such as finding widgets and icons saw Ferret-UI maintain 92% and 93% accuracy, respectively, marking a 20% and 22% improvement compared to existing models. These figures underscore Ferret-UI's improved capability in understanding the mobile user interface, setting new benchmarks in accuracy and reliability for the field.
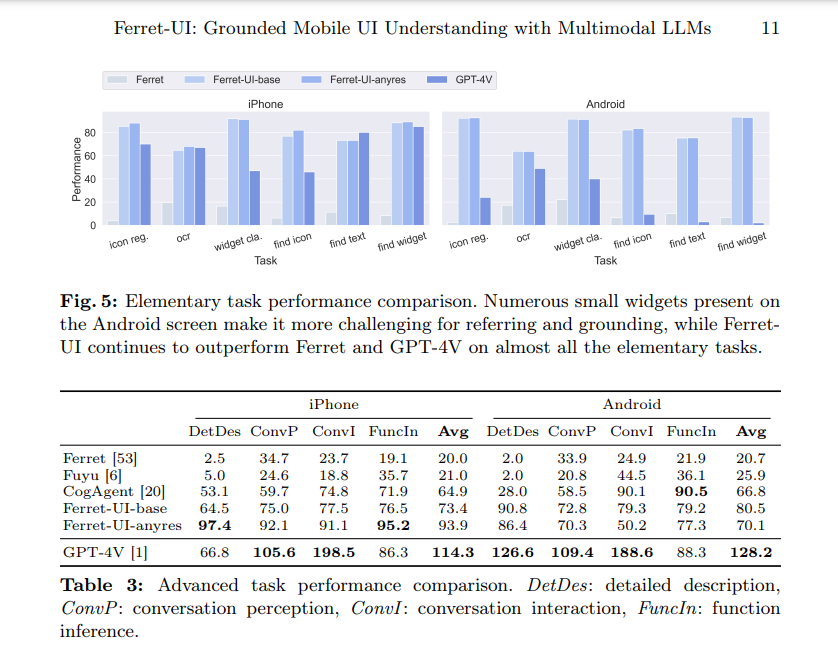
In conclusion, the research presented Ferret-UI, Apple's novel approach to improving understanding of the mobile user interface through an “any resolution” strategy and a specialized training regimen. By leveraging detailed aspect ratio tuning and comprehensive data sets, Ferret-UI significantly advanced task-specific performance metrics, notably outperforming those of existing models. The quantitative results underline the improved interpretive capabilities of the model. But it's not just about the numbers. The success of Ferret-UI illustrates the potential for more intuitive and accessible mobile app interactions, paving the way for future advances in user interface understanding. It's a model that can really make a difference in the way we interact with mobile user interfaces.
Review the Paper. All credit for this research goes to the researchers of this project. Also, don't forget to follow us on twitter.com/Marktechpost”>twitter. Join our Telegram channel, Discord channeland LinkedIn Grabove.
If you like our work, you will love our Newsletter..
Don't forget to join our SubReddit over 40,000ml
Nikhil is an internal consultant at Marktechpost. He is pursuing an integrated double degree in Materials at the Indian Institute of technology Kharagpur. Nikhil is an ai/ML enthusiast who is always researching applications in fields like biomaterials and biomedical science. With a strong background in materials science, he is exploring new advances and creating opportunities to contribute.
<script async src="//platform.twitter.com/widgets.js” charset=”utf-8″>