Scientific research is often limited by resource limitations and time-consuming processes. Tasks such as hypothesis testing, data analysis, and report writing require significant effort, leaving little room to explore multiple ideas simultaneously. The increasing complexity of research topics further exacerbates these problems, as it requires a combination of field experience and technical skills that are not always available. While ai technologies have shown promise in alleviating some of these burdens, they often lack integration and fail to address the entire research lifecycle in a coherent manner.
In response to these challenges, researchers at AMD and John Hopkins have developed Agent Laba self-contained framework designed to help scientists navigate the research process from start to finish. This innovative system employs large language models (LLMs) to streamline key stages of research, including literature review, experimentation, and report writing.
Agent Laboratory comprises a portfolio of specialized agents tailored to specific investigative tasks. “PhD” agents handle literature reviews, “ML Engineer” agents focus on experimentation, and “Professor” agents compile findings into academic reports. Importantly, the framework allows for varying levels of human involvement, allowing users to guide the process and ensure results align with their goals. By leveraging advanced LLMs like o1-preview, Agent Laboratory offers a practical tool for researchers looking to optimize both efficiency and cost.
Technical approach and key benefits
The Agent Laboratory workflow is structured around three main components:
- Literature Review: The system retrieves and selects relevant research articles using resources such as arXiv. Through iterative refinement, it creates a high-quality baseline to support subsequent stages.
- Experimentation: The “mle-solver” module autonomously generates, tests and refines machine learning code. Its workflow includes command execution, error handling, and iterative improvements to ensure reliable results.
- Report writing: The “paper-solver” module generates academic reports in LaTeX format, adhering to established structures. This phase includes iterative editing and integration of comments to improve clarity and consistency.
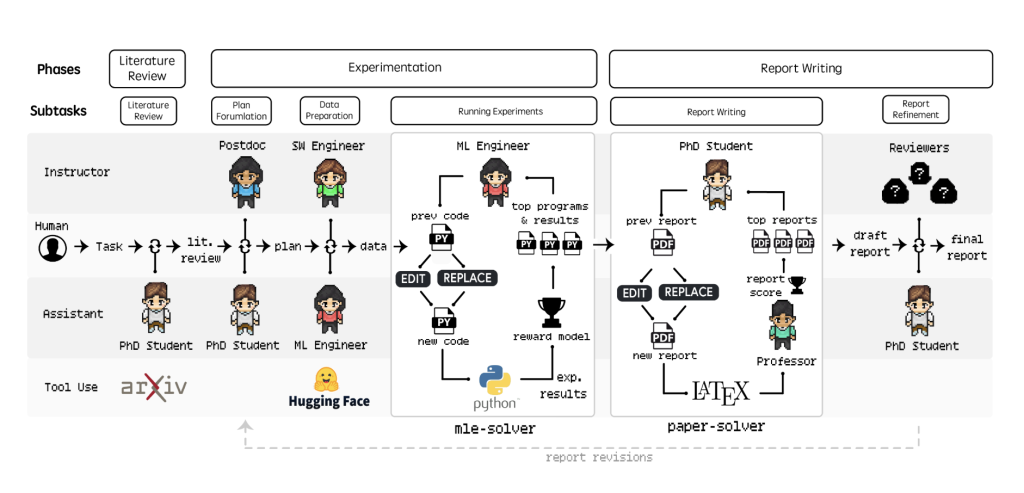
The framework offers several benefits:
- Efficiency: By automating repetitive tasks, Agent Laboratory reduces research costs by up to 84% and shortens project timelines.
- Flexibility: Researchers can choose their level of participation, maintaining control over critical decisions.
- Scalability: Automation frees up time for high-level planning and ideation, allowing researchers to manage larger workloads.
- Reliability: Performance benchmarks like MLE-Bench highlight the system's ability to deliver reliable results on various tasks.
Evaluation and findings
The usefulness of Agent Laboratory has been validated through extensive testing. Articles generated using the o1-preview backend consistently scored high in usefulness and reporting quality, while o1-mini demonstrated strong experimental reliability. The framework's co-pilot mode, which integrates user feedback, was especially effective in producing impactful research results.
Runtime and cost analyzes revealed that the GPT-4o backend was the most cost-effective, completing projects for as little as $2.33. However, the o1 preview achieved a higher success rate of 95.7% across all tasks. In MLE-Bench, Agent Laboratory's mle-solver outperformed its competitors, winning multiple medals and outperforming human bases in several challenges.
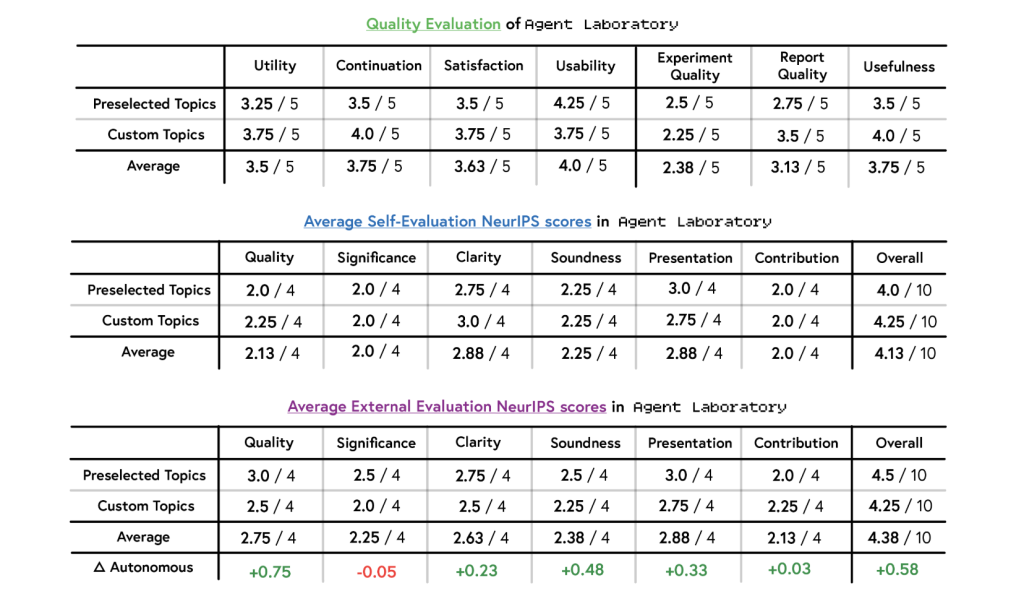
Conclusion
Agent Laboratory offers a thoughtful approach to addressing bottlenecks in modern research workflows. By automating routine tasks and improving human-ai collaboration, it allows researchers to focus on innovation and critical thinking. While the system has limitations, including occasional inaccuracies and challenges with automated assessment, it provides a solid foundation for future advancements.
Looking ahead, further improvements to Agent Laboratory could expand its capabilities, making it an even more valuable tool for researchers across disciplines. As adoption grows, it has the potential to democratize access to advanced research tools, fostering a more inclusive and efficient scientific community.
Verify he Paper, Codeand Project page. All credit for this research goes to the researchers of this project. Also, don't forget to follow us on <a target="_blank" href="https://x.com/intent/follow?screen_name=marktechpost” target=”_blank” rel=”noreferrer noopener”>twitter and join our Telegram channel and LinkedIn Grabove. Don't forget to join our SubReddit over 60,000 ml.
UPCOMING FREE ai WEBINAR (JANUARY 15, 2025): <a target="_blank" href="https://info.gretel.ai/boost-llm-accuracy-with-sd-and-evaluation-intelligence?utm_source=marktechpost&utm_medium=newsletter&utm_campaign=202501_gretel_galileo_webinar”>Increase LLM Accuracy with Synthetic Data and Assessment Intelligence–<a target="_blank" href="https://info.gretel.ai/boost-llm-accuracy-with-sd-and-evaluation-intelligence?utm_source=marktechpost&utm_medium=newsletter&utm_campaign=202501_gretel_galileo_webinar”>Join this webinar to learn practical information to improve LLM model performance and accuracy while protecting data privacy..
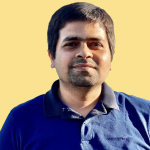
Asif Razzaq is the CEO of Marktechpost Media Inc.. As a visionary entrepreneur and engineer, Asif is committed to harnessing the potential of artificial intelligence for social good. Their most recent endeavor is the launch of an ai media platform, Marktechpost, which stands out for its in-depth coverage of machine learning and deep learning news that is technically sound and easily understandable to a wide audience. The platform has more than 2 million monthly visits, which illustrates its popularity among the public.