The main goal of ai is to create interactive systems capable of solving various problems, including those of medical ai aimed at improving patient outcomes. Large language models (LLMs) have demonstrated significant problem-solving capabilities, outperforming human scores on exams such as the USMLE. While LLMs can improve healthcare accessibility, they still face limitations in real-world clinical settings due to the complexity of clinical tasks involving sequential decision making, uncertainty management, and compassionate patient care. Current assessments focus primarily on static multiple-choice questions, without fully capturing the dynamic nature of clinical work.
The USMLE evaluates medical students in terms of fundamental knowledge, clinical application, and independent practice skills. In contrast, the Objective Structured Clinical Examination (OSCE) assesses practical clinical skills through simulated scenarios, offering direct observation and a comprehensive assessment. Language models in medicine are primarily assessed using knowledge-based benchmarks such as Medqa, which consists of challenging pairs of medical questions. Recent efforts are focused on refining applications of language models in healthcare through red teams and the creation of new benchmarks such as EquityMedQA to address bias and improve evaluation methods. Additionally, advances in clinical decision-making simulations, such as AMIE, show promise for improving diagnostic accuracy in medical ai.
Researchers from Stanford University, Johns Hopkins University and the Albert Einstein Israeli Hospital present AgentClinic, an open source benchmark for simulating clinical environments using language, patients, clinicians, and measurement agents. Expands previous simulations by including medical exams (e.g., temperature, blood pressure) and ordering medical images (e.g., MRI, x-ray) through dialogue. Additionally, AgentClinic supports 24 biases found in clinical settings.
AgentClinic features four language agents: patient, doctor, measurer, and moderator. Each agent has specific functions and unique information to simulate clinical interactions. The patient agent provides symptom information without knowing the diagnosis, the measuring agent provides medical readings and test results, the medical agent evaluates the patient and orders tests, and the moderator evaluates the doctor's diagnosis. AgentClinic also includes 24 biases relevant to clinical settings. Agents are created using medical questions selected from USMLE and NEJM case challenges to create structured scenarios for evaluation using language models such as GPT-4.
The accuracy of the different language models (GPT-4, Mixtral-8x7b, GPT-3.5 and LLAMA 2 70B-CHAT) is evaluated in AgentClinic-MEDQA, where each model acts as a medical agent that diagnoses patients through dialogue . GPT-4 achieved the highest accuracy at 52%, followed by GPT-3.5 at 38%, Mixtral-8x7B at 37%, and Llama 2 with 70B-chat at 9%. Comparison with MedQA accuracy showed weak predictability for AgentClinic-MedQA accuracy, similar to studies on medical residents' performance relative to the USMLE.
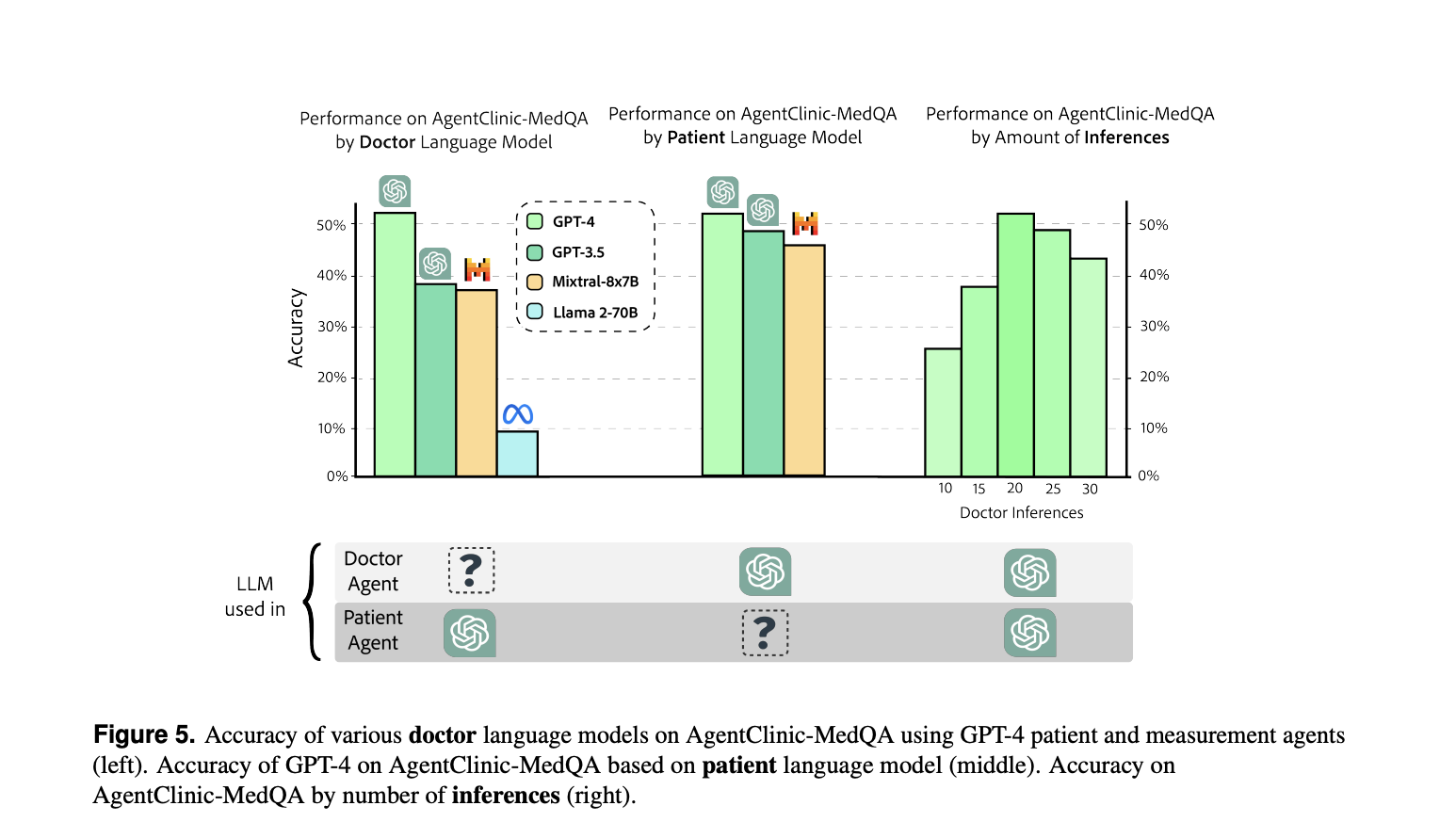
In summary, the researchers of this work present AgentClinic, a benchmark for simulating clinical environments with 15 multimodal language agents and 107 single language agents based on USMLE cases. These agents exhibit 23 biases, affecting diagnostic accuracy and doctor-patient interactions. GPT-4, the highest performing model, shows reduced accuracy (1.7%-2%) with cognitive biases and larger reductions (1.5%) with implicit biases, affecting patient willingness and confidence in monitoring. Cross-talk between patient and doctor models improves accuracy. Limited or excessive interaction time decreases accuracy, with a 27% reduction at N=10 interactions and a 4%-9% reduction at N>20 interactions. GPT-4V achieves around 27% accuracy in a NEJM case-based multimodal clinical setting.
Review the Paper and Project. All credit for this research goes to the researchers of this project. Also, don't forget to follow us on twitter.com/Marktechpost”>twitter. Join our Telegram channel, Discord channeland LinkedIn Grabove.
If you like our work, you will love our Newsletter..
Don't forget to join our 42k+ ML SubReddit
Aswin AK is a consulting intern at MarkTechPost. He is pursuing his dual degree from the Indian Institute of technology Kharagpur. He is passionate about data science and machine learning, bringing a strong academic background and practical experience solving real-life domain challenges.
<script async src="//platform.twitter.com/widgets.js” charset=”utf-8″>