Proteins, macromolecules essential for biological processes such as metabolism and immune response, follow the sequence-structure-function paradigm, where amino acid sequences determine three-dimensional structures and functions. Computational protein science aims to decode this relationship and design proteins with desired properties. Traditional ai models have achieved significant success in specific protein modeling tasks such as structure prediction and design. However, these models face challenges in understanding the “grammar” and “semantics” of protein sequences and lack generalization across tasks. Recently, protein language models (pLMs) have emerged that leverage LLM techniques, enabling advances in protein understanding, function prediction, and design.
Researchers from institutions such as Hong Kong Polytechnic University, Michigan State University, and Mohamed bin Zayed University of artificial intelligence have advanced computational protein science by integrating LLM to develop pLM. These models effectively capture protein knowledge and address sequence, structure, and function reasoning problems. This survey systematically classifies pLMs into multimodal, sequence-based, structure-function-enhanced models, and explores their applications in protein structure prediction, function prediction, and design. It highlights the impact of pLMs on antibody design, enzyme engineering, and drug discovery, while discussing challenges and future directions, providing insights for ai and biology researchers in this growing field.
Protein structure prediction is a critical challenge in computational biology due to the complexity of experimental techniques such as x-ray crystallography and NMR. Recent advances such as AlphaFold2 and RoseTTAFold have significantly improved structure prediction by incorporating geometric and evolutionary constraints. However, these methods still face challenges, especially with orphan proteins that lack homologous sequences. To address these issues, single sequence prediction methods, such as ESMFold, use pLM to predict protein structures without relying on multiple sequence alignments (MSA). These methods offer faster and more universal predictions, particularly for proteins without homology, although there is still room to improve accuracy.
pLMs have had a significant impact on experimental and computational protein science, particularly in applications such as antibody design, enzyme design, and drug discovery. In antibody design, pLMs can propose antibody sequences that specifically bind to target antigens, offering a more controlled and cost-effective alternative to traditional animal-based methods. These models, such as PALMH3, have successfully engineered antibodies targeting several SARS-CoV-2 variants, demonstrating improved neutralization and affinity. Similarly, pLMs play a key role in enzyme design by optimizing wild-type enzymes for improved stability and new catalytic functions. For example, InstructPLM has been used to redesign enzymes such as PETase and L-MDH, improving their efficiency compared to the wild type.
In drug discovery, pLMs help predict interactions between drugs and target proteins, accelerating the screening of potential drug candidates. Models like TransDTI can classify drug-target interactions, helping to identify promising compounds for diseases. Furthermore, ConPLex leverages contrastive learning to predict kinase-drug interactions, successfully confirming several high-affinity binding interactions. These advances in pLM applications streamline the drug discovery process and contribute to the development of more effective therapies with improved efficiency and safety profiles.
In conclusion, the study provides an in-depth look at the role of LLMs in protein science, covering both fundamental concepts and recent advances. The biological basis of protein modeling, the categorization of pLMs based on their ability to understand sequence, structure, and functional information, and their applications in predicting protein structure, function, and design are discussed. The review also highlights the potential of pLMs in practical fields such as antibody design, enzyme engineering, and drug discovery. Finally, it outlines promising future directions in this rapidly advancing field, emphasizing the transformative impact of ai on computational protein science.
Verify he Paper. All credit for this research goes to the researchers of this project. Also, don't forget to follow us on <a target="_blank" href="https://x.com/intent/follow?screen_name=marktechpost” target=”_blank” rel=”noreferrer noopener”>twitter and join our Telegram channel and LinkedIn Grabove. Don't forget to join our SubReddit over 70,000 ml.
<a target="_blank" href="https://nebius.com/blog/posts/studio-embeddings-vision-and-language-models?utm_medium=newsletter&utm_source=marktechpost&utm_campaign=embedding-post-ai-studio” target=”_blank” rel=”noreferrer noopener”> (Recommended Reading) Nebius ai Studio Expands with Vision Models, New Language Models, Embeddings, and LoRA (Promoted)
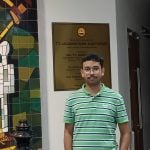
Sana Hassan, a consulting intern at Marktechpost and a dual degree student at IIT Madras, is passionate about applying technology and artificial intelligence to address real-world challenges. With a strong interest in solving practical problems, he brings a new perspective to the intersection of ai and real-life solutions.