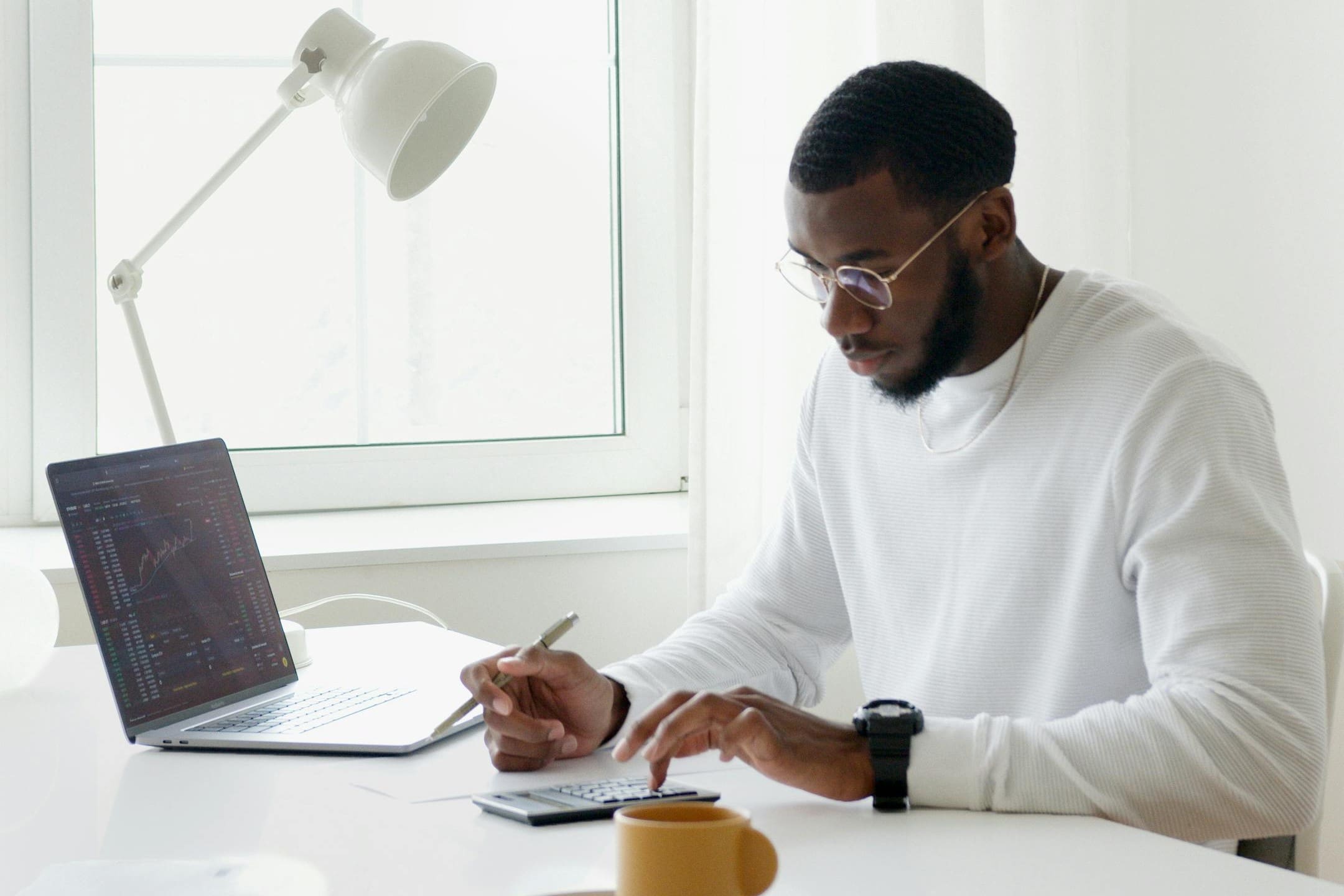
Photo by Anna Nekrashevich
With the advancement of data technology in recent years, we have seen an increase in companies implementing data science. Many companies are now trying to recruit top talent for their data project to gain a competitive advantage. One of those talents is the data scientist.
Data scientists have proven capable of bringing enormous value to companies. However, what sets the skills of data scientists apart from others? It's not an easy question to answer as data scientists are a big umbrella and the job responsibilities and skills required differ for each company. However, there are skills that data scientists will need if they want to differentiate themselves from others.
This article will look at five essential skills for data scientists in 2024. I wouldn't argue Programming language either Machine learning since they are always necessary skills. I'm also not talking about generative ai skills as those are trending skills, but data science is more than that. I would only talk about other emerging skills essential for the 2024 landscape.
What are these skills? Let's get into it.
Cloud computing is a service over the Internet (“Cloud”) that may include servers, analytics software, networking, security, and many more. It is designed to adapt to user preferences and offer resources as needed.
In the current data science trend, many companies have started implementing cloud computing to scale their businesses or minimize infrastructure costs. From small startups to large enterprises, the use of cloud computing has become evident. This is why you can start to see that the current data science job role would require you to have cloud computing experience.
There are many cloud computing services, but it is not necessary to learn them all, as mastering one means navigating more easily to the other platforms. If you're having a hard time deciding which one to learn initially, you can start with a larger platform, such as AWS, GCP, or Azure.
You can learn more about cloud computing with this article from Aryan Garg's Beginner's Guide to Cloud Computing.
Machine Learning Operations, or MLOps, is a collection of techniques and tools for deploying ML models in production. MLOps aims to avoid the technical debt from our Machine Learning application by streamlining the deployment of ML models into production, improving model quality and performance while implementing best practices in CI/CD, with continuous monitoring of machine learning models.
MLOps has become one of the most sought-after skills for data scientists and you can see the increase in MLOps requirements in job postings. Previously, MLOps jobs could be delegated to a machine learning engineer. However, the requirements for data scientists to understand MLOps have become greater than ever. This is because data scientists must ensure that their machine learning model is ready to integrate with the production environment, which only the model creator knows best.
This is why learning about MLOps in 2024 will be beneficial if you want to advance your career in data science. For more information on the MLOps topic, check out KDnuggets' first tech Brief, which discusses everything about MLOps.
Big Data can be described as the Three Vs, which comprise Volume, which refers to the massive amounts of data generated; Speed, which explains the speed with which data is produced and processed; and Variety, which refers to various types of data (structured to unstructured).
Big Data technologies have become important in many companies, as many of the insights and products depend on how they can do something with the Big Data they have. It is one thing to have big data, but only by processing it can companies obtain value from it. This is the reason why many companies are trying to hire data scientists who possess skills in big data technology.
Many technologies are included in these terms when we talk about Big Data Technologies. However, it could be classified into four types: data warehousing, data mining, data analysis, and data visualization.
Below are some popular tools that job postings often list as necessary:
-Apache Hadoop
-Apache Spark
-MongoDB
-Chart
– Fast miner
You don't have to master all the tools available, but understanding some of them will certainly improve your career. To learn more about Big Data technologies, here is an introductory article called Working with Big Data: Tools and Techniques by Nate Rosidi that could jump-start your Big Data journey.
Data scientists need technical skills and strong experience in the field to advance their careers. A junior data scientist might want to model machine learning to achieve the highest technical metrics, but the senior understands that our model must put business values before everything else.
Domain expertise means we understand the business of the industry we are working in. By understanding the business, we could better align with the business user, select better metrics for the model, and frame projects in a way that impacts the business. In 2024, it will become especially important as companies begin to understand how data science could bring significant value.
The problem with acquiring domain expertise is that it can only be learned effectively if we are already working as data scientists in that industry. So how could we acquire this skill if we don't work in the industry we want? There are a few ways, including:
– Take online courses and certifications in related industries.
– Active networking on social networks.
– Contribute to the open source project.
– Have a side project related to the industry.
– Find a mentor
– Do an internship
These are suggested ways to gain domain expertise, but you can be more creative in finding expertise. The article “Is domain knowledge a barrier to starting a career in data?” by Vaishali Lambe can also help you gain domain expertise.
Some may view the data as numbers or words in the database without worrying about the individual that this data describes. However, much of this data was private information that could harm users and the company if mishandled. The topic is becoming even more important in this modern era as data collection and processing becomes easier.
Ethics in data science deals with the moral principles that guide how data scientists should work. The field covers the potential impact of our data science project on individuals and society, which should follow the best moral decision we can make. The topic often has to do with bias, fairness, explainability, and consent.
On the other hand, data privacy is a field related to the legality of how we collect, process, manage and share data. Its objective is to protect personal information coming from the individual and prevent its misuse. Each area may have a different data privacy framework; For example, the General Data Protection Regulation (GDPR) in Europe generally applies only to personal data in Europe.
Knowledge of data ethics and privacy has become essential skills for data scientists, as the consequences of violating them are serious. Nisha Arya's article on Ethics and Data Privacy could become his starting point to better understand these issues.
This article discusses five essential skills every data scientist will need in 2024. The skills include:
- Cloud Computing
- MLOps
- big data technology
- Experience in the field
- Ethics and Data Privacy
I hope that helps! Share your thoughts on the skills listed here and add your comment below.
Cornellius Yudha Wijaya He is an assistant data science manager and data writer. While working full-time at Allianz Indonesia, she loves sharing Python tips and data through social media and print media.