Multilingual Natural Language Processing (NLP) is a rapidly advancing field that aims to develop language models capable of understanding and generating text in multiple languages. These models facilitate effective communication and access to information between different linguistic backgrounds. The importance of this field lies in its potential to bridge the gap between speakers of different languages, making technological advances in ai accessible globally. However, the development of such models presents significant challenges due to the complexities of handling multiple languages simultaneously.
One of the main problems with multilingual natural language processing is the predominant focus on a few major languages, such as English and Chinese. This limited concentration results in a significant performance gap for models when applied to less widely spoken languages. Consequently, many languages still need to be represented, limiting the applicability and fairness of ai technologies. Addressing this disparity requires innovative approaches to improve the quality and diversity of multilingual datasets, ensuring that ai models can perform effectively across a broad spectrum of languages.
Traditional methods for improving multilingual language models typically involve translating preference data from English into other languages. While this strategy helps to some extent, it introduces several problems, including translation artifacts that can degrade model performance. Relying heavily on translation can lead to a lack of diversity in the data, which is crucial for robust model training. Collecting high-quality multilingual preference data using human annotations is one potential solution, but it is expensive and time-consuming, making it impractical for large-scale applications.
Researchers at Cohere For ai have developed a novel and scalable method for generating high-quality multilingual feedback data. This method aims to balance data coverage and improve the performance of multilingual large language models (LLMs). The research team introduced a unique approach that leverages diverse multilingual prompts and completions generated by multiple LLMs. This strategy not only increases data diversity but also helps avoid common issues associated with translation artifacts. The models used in this research include Cohere’s Command and Command R+, which are specifically designed for multilingual capabilities.
The methodology involves translating approximately 50,000 English prompts into 22 additional languages using the NLLB 3.3B model. These prompts are then used to generate full responses in each language, ensuring high diversity and quality in the data. The research team also compared full responses generated directly in the target language to those translated from English and found that the former significantly reduced the occurrence of translation artifacts. This approach resulted in a diverse set of multilingual preference pairs crucial for effective preference optimization.
The performance of the preference-trained model was evaluated against several state-of-the-art multilingual LLMs. The results were impressive: the preference-trained model achieved a 54.4% success rate against Aya 23 8B, the current leading multilingual LLM in its benchmark class. Furthermore, the model showed a 69.5% or higher success rate against other widely used models such as Gemma-1.1-7B-it, Meta-Llama3-8B-Instruct, and Mistral-7B-Instruct-v0.3. These results highlight the effectiveness of the researchers’ approach to improving the performance of multilingual LLMs through improved preference optimization.
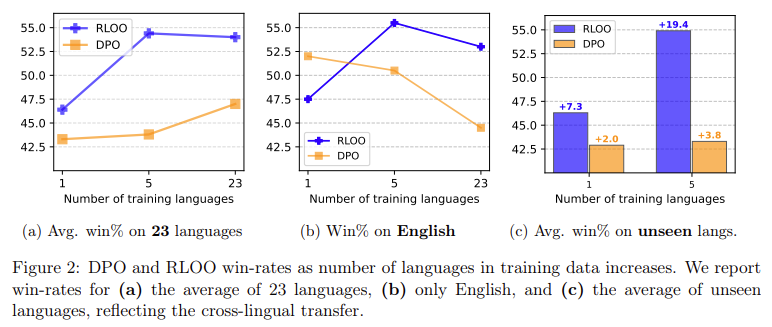
Further analysis revealed that increasing the number of languages in the training data consistently improved model performance. For example, training with five languages resulted in a 54.9% success rate on unseen languages, compared to 46.3% when training on English alone. Furthermore, online preference optimization methods, such as reinforcement learning from human feedback (RLHF), proved to be more effective than offline methods such as direct preference optimization (DPO). Online techniques achieved higher success rates, with RLOO outperforming DPO by a margin of 10.6% in some cases.
In conclusion, the research conducted by Cohere For ai demonstrates the critical importance of high-quality, diverse, and multilingual data for training effective multilingual language models. The innovative methods introduced by the research team address the challenges of data sparsity and quality, resulting in performance improvements across a wide range of languages. The study not only sets a new benchmark for multilingual preference optimization but also underlines the value of online training methods for achieving superior interlingual transfer and overall model performance.
Review the Paper. All credit for this research goes to the researchers of this project. Also, don't forget to follow us on twitter.com/Marktechpost”>twitter.
Join our Telegram Channel and LinkedIn GrAbove!.
If you like our work, you will love our Newsletter..
Don't forget to join our Subreddit with over 46 billion users
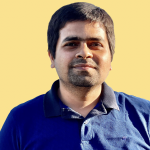
Asif Razzaq is the CEO of Marktechpost Media Inc. As a visionary engineer and entrepreneur, Asif is committed to harnessing the potential of ai for social good. His most recent initiative is the launch of an ai media platform, Marktechpost, which stands out for its in-depth coverage of machine learning and deep learning news that is technically sound and easily understandable to a wide audience. The platform has over 2 million monthly views, illustrating its popularity among the public.
<script async src="//platform.twitter.com/widgets.js” charset=”utf-8″>