Large language models (LLMs) have gained significant attention for their impressive performance on a variety of tasks, from summarizing news to writing code to answering trivia questions. Their effectiveness extends to real-world applications, with models such as GPT-4 successfully passing legal and medical licensing exams. However, LLMs face two critical challenges: hallucinations and performance disparities. Hallucination, where LLMs generate plausible but inaccurate text, poses risks in factual recall tasks. Performance disparities manifest as inconsistent reliability across different subsets of input, often tied to sensitive attributes such as race, gender, or language. These issues underscore the need for continued development of diverse benchmarks to assess the reliability of LLMs and identify potential equity issues. Creating comprehensive benchmarks is crucial not only to assess overall performance, but also to quantify and address performance disparities, ultimately working toward building models that perform equitably across all user groups.
Existing research on LLM students’ factual recall has yielded mixed results, with models demonstrating some competence but also being prone to data fabrication. Studies have linked accuracy to entity popularity but have primarily focused on overall error rates rather than geographic disparities. While some researchers have explored geographic information recall, these efforts have been limited in scope. In the broader context of ai bias, disparities have been observed across diverse demographic groups in different domains. However, a comprehensive and systematic examination of country-by-country disparities in LLM students’ factual recall has been lacking, highlighting the need for a more robust and geographically sensitive assessment approach.
Researchers at the University of Maryland and Michigan State University propose a robust benchmark called world Bank WorldBench is a benchmarking tool to investigate potential geographic disparities in the data retrieval capabilities of large language models (LLMs). This approach aims to determine whether LLMs demonstrate different levels of accuracy when answering questions about different parts of the world. WorldBench uses country-specific indicators from the World Bank, employing an indicator-agnostic automated request and parsing process. The benchmark incorporates 11 diverse indicators for approximately 200 countries, generating 2,225 questions per LLM. The study evaluates 20 state-of-the-art LLMs released in 2023, including open-source models such as Llama-2 and Vicuna, as well as private commercial models such as GPT-4 and Gemini. This comprehensive evaluation method allows for a systematic analysis of LLM performance across multiple geographic regions and income groups.
WorldBench is based on statistics from the World Bank, a global organization that tracks numerous development indicators in nearly 200 countries. This approach offers several unique advantages: equitable representation of all countries, guaranteed data quality from a reliable source, and flexibility in indicator selection. The benchmark incorporates 11 different indicators, resulting in 2,225 questions reflecting an average of 202 countries per indicator.
The evaluation process involves a standardized application method using a template with basic instructions and an example. An automated analysis system extracts numerical values from the LLM results, and the absolute relative error is used as a comparison metric. The effectiveness of the evaluation process was validated through manual inspection studies, which confirmed its completeness and accuracy. Ground truth values are determined by averaging statistics from the past three years to maximize country inclusion. This comprehensive methodology allows for systematic analysis of LLM performance across multiple geographic regions and income groups.
The study reveals significant geographic disparities in master’s students’ memorization of facts across different regions and income groups. On average, North America and Europe and Central Asia experienced the lowest error rates (0.316 and 0.321 respectively), while sub-Saharan Africa had the highest (0.461), about 1.5 times higher than North America. Error rates increased steadily as countries’ income levels decreased; high-income countries had the lowest error (0.346) and low-income countries the highest (0.480).
In terms of countries, the disparities were even more pronounced. The 15 countries with the lowest error rates were all high-income, mostly European, while the 15 with the highest were all low-income. Strikingly, error rates almost tripled between these two groups. These disparities were consistent across all 20 LLMs assessed and all 11 indicators used, and the observed disparities far exceeded those expected from a random categorization of countries. Even the best-performing LLMs showed substantial room for improvement, with the lowest mean absolute relative error at 0.19 and most models close to 0.4.
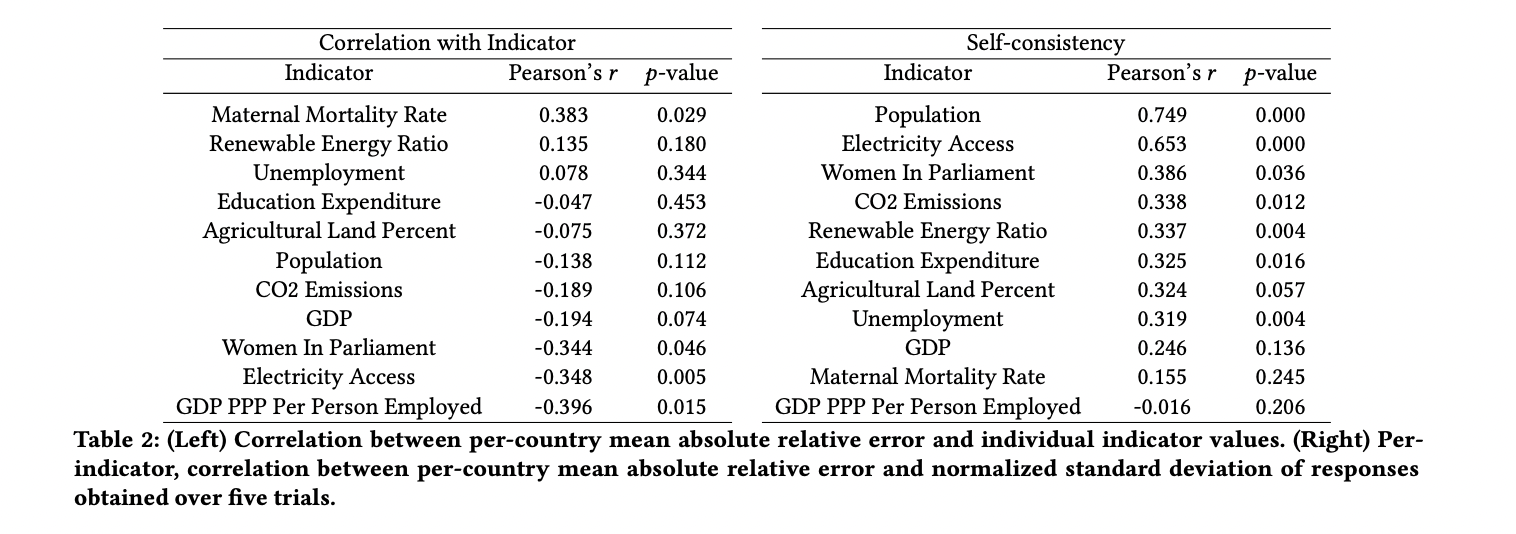
This study presents WorldBench, a robust benchmark for quantifying geographic disparities in master’s students’ fact recall, which reveals widespread and consistent biases across 20 evaluated master’s programs. The study demonstrates that Western and higher-income countries consistently experience lower error rates on fact recall tasks. By using data from the World Bank, WorldBench offers a flexible and continuously updated framework for assessing these disparities. This benchmark serves as a valuable tool for identifying and addressing geographic biases in master’s programs, which could aid in the development of future models that work equitably across regions and income levels. Ultimately, WorldBench aims to contribute to the creation of more inclusive and fair language models globally that can effectively serve users from all parts of the world.
Review the Paper. All credit for this research goes to the researchers of this project. Also, don't forget to follow us on twitter.com/Marktechpost”>twitter.
Join our Telegram Channel and LinkedIn GrAbove!.
If you like our work, you will love our Newsletter..
Don't forget to join our Subreddit with over 46 billion users
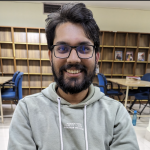
Asjad is a consultant intern at Marktechpost. He is pursuing Bachelors in Mechanical Engineering from Indian Institute of technology, Kharagpur. Asjad is a Machine Learning and Deep Learning enthusiast who is always researching the applications of Machine Learning in the healthcare domain.
<script async src="//platform.twitter.com/widgets.js” charset=”utf-8″>