In our rapidly advancing world of artificial intelligence (ai), we have witnessed remarkable advancements in natural language processing (NLP) capabilities. From virtual assistants that can converse fluently to language models that can generate human-like text, the potential applications are truly mind-boggling. However, as these ai systems become more sophisticated, they also become increasingly complex and opaque, operating as inscrutable “black boxes,” a cause for concern in critical domains such as healthcare, finance, and justice. penal.
A team of researchers at Imperial College London has proposed a framework for evaluating the explanations generated by artificial intelligence systems, which will allow us to understand the reasons for their decisions.
At the heart of their work is a fundamental question: How can we ensure that ai systems make predictions for the right reasons, especially in high-risk scenarios where human lives or important resources are at stake?
Researchers have identified three distinct classes of explanations that ai systems can provide, each with its own structure and level of complexity:
- Free-form explanations: They are the simplest form and consist of a sequence of propositions or statements that attempt to justify the ai's prediction.
- Deductive explanations: Starting from free-form explanations, deductive explanations link propositions through logical relationships, forming chains of reasoning similar to a human thought process.
- Argumentative explanations: Argumentative explanations, the most intricate of the three, mimic human debates by presenting arguments with premises and conclusions, connected through relationships of support and attack.
Researchers have laid the foundation for a comprehensive evaluation framework by defining these classes of explanation. But his work doesn't end there.
To ensure the validity and usefulness of these explanations, researchers have proposed a set of properties adapted to each class of explanation. For example, the coherence of free-form explanations is evaluated, ensuring that propositions do not contradict each other. On the other hand, deductive explanations are evaluated for relevance, non-circularity, and non-redundancy, ensuring that chains of reasoning are logically sound and free of extraneous information.
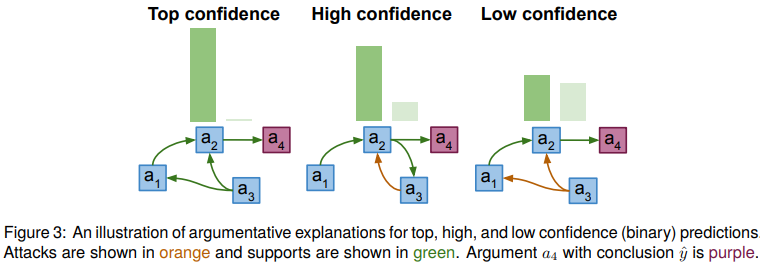
Argumentative explanations, being the most complex, are subject to rigorous evaluation through properties such as dialectical fidelity and acceptability. These properties ensure that explanations accurately reflect the ai system's confidence in its predictions and that the arguments presented are logically consistent and defensible.
But how do we quantify these properties? Researchers have devised clever metrics that assign numerical values to explanations based on their compliance with defined properties. For example, the coherence metric (Coh) measures the degree of coherence in free-form explanations, while the acceptability metric (Acc) evaluates the logical soundness of argumentative explanations.
The importance of this research cannot be underestimated. We take a crucial step toward building trust in these systems by providing a framework for evaluating the quality and human-likeness of ai-generated explanations. Imagine a future where ai assistants in healthcare can not only diagnose diseases but also provide clear, structured explanations for their decisions, allowing doctors to examine reasoning and make informed decisions.
Additionally, this framework has the potential to foster accountability and transparency in ai systems, ensuring that they do not perpetuate biases or make decisions based on flawed logic. As ai permeates more aspects of our lives, these safeguards become paramount.
The researchers have laid the foundation for future advances in explainable ai, inviting collaboration from the broader scientific community. With continued effort and innovation, we will one day be able to unlock the full potential of ai while maintaining human oversight and control, a testament to the harmony between technological progress and ethical responsibility.
Review the Paper. All credit for this research goes to the researchers of this project. Also, don't forget to follow us on twitter.com/Marktechpost”>twitter. Join our Telegram channel, Discord Channeland LinkedIn Grabove.
If you like our work, you will love our Newsletter..
Don't forget to join our 39k+ ML SubReddit
Vibhanshu Patidar is a Consulting Intern at MarktechPost. He is currently pursuing a bachelor's degree at the Indian Institute of technology (IIT) Kanpur. He is a robotics and machine learning enthusiast with a knack for unraveling the complexities of algorithms that bridge theory and practical applications.
<script async src="//platform.twitter.com/widgets.js” charset=”utf-8″>