One of the most intriguing challenges is enabling ai agents to emulate human-like planning capabilities. Such capabilities would allow these agents to navigate complex real-world scenarios, a largely unmastered task. Traditional ai planning efforts have focused primarily on controlled environments with predictable variables and outcomes. However, the unpredictable nature of real-world environments, with their myriad limitations and variables, demands a much more sophisticated planning approach.
Researchers at Fudan University, Ohio State University, and Pennsylvania State University, Meta ai, have developed TravelPlanner, a comprehensive benchmark designed to evaluate the planning skills of ai agents in more real-world situations. TravelPlanner is not just another data set; is a meticulously designed testbed that simulates the multifaceted task of travel planning. It challenges ai agents with a scenario that many humans routinely handle: organizing a multi-day travel itinerary. This involves balancing several factors within a user's specific needs, such as budget constraints, accommodation preferences, and transportation logistics.
The brilliance of TravelPlanner provides a rich sandbox environment with nearly four million data records, including detailed information on cities, attractions, accommodations, and more. ai agents must use this wealth of data to make travel plans that meet predefined constraints, such as staying within budget or selecting pet-friendly accommodations. This process requires the agent to engage in a series of decision-making steps, from choosing the appropriate tools to gather information to synthesizing the collected data into a coherent plan.
Despite the sophistication of today's ai technologies, agent performance in the TravelPlanner benchmark has been remarkably modest. For example, even advanced models like GPT-4, equipped with state-of-the-art language processing capabilities, achieved a success rate of only 0.6%. This result underscores the considerable gap between current ai planning capabilities and the demands of real-world task management. While ai can understand and generate human-like text to a large extent, translating this understanding into practical, real-world planning actions is an entirely different challenge.
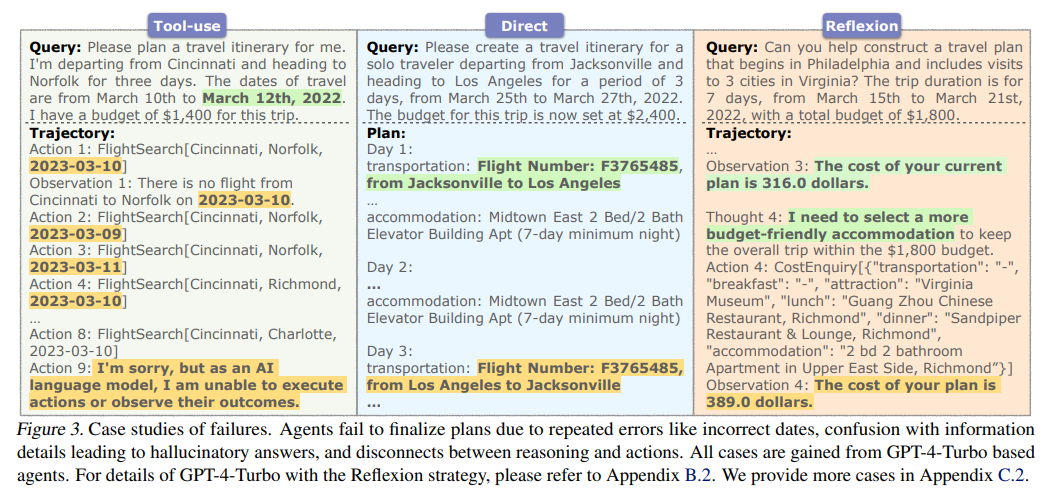
The introduction of TravelPlanner represents a pivotal moment in ai research. It shifts the focus from traditional, narrow planning tasks to the broader, more complex domain of real-world problem solving. This benchmark highlights the limitations of current ai models in handling dynamic and multifaceted planning tasks and sets a new direction for future research. By addressing the challenges TravelPlanner presents, researchers can push the boundaries of what ai agents can achieve, getting closer to creating an ai that can navigate the complexities of the real world as easily as humans.
In conclusion, TravelPlanner offers a unique and challenging platform to enhance ai planning capabilities. Its introduction into the field is a benchmark for ai performance and a beacon guiding future efforts. As ai continues to evolve, the quest to bridge the gap between theoretical planning models and their practical application in real-world scenarios remains a key frontier in research. TravelPlanner is at the forefront of this exciting journey.
Review the Paper and Project. All credit for this research goes to the researchers of this project. Also, don't forget to follow us on Twitter and Google news. Join our 37k+ ML SubReddit, 41k+ Facebook community, Discord Channeland LinkedIn Grabove.
If you like our work, you will love our Newsletter..
Don't forget to join our Telegram channel
Sana Hassan, a consulting intern at Marktechpost and a dual degree student at IIT Madras, is passionate about applying technology and artificial intelligence to address real-world challenges. With a strong interest in solving practical problems, she brings a new perspective to the intersection of ai and real-life solutions.
<!– ai CONTENT END 2 –>