Despite the amazing advances and achievements in the field of technology, classical diffusion models still face challenges in imaging, particularly due to their slow sampling rate and the need for extensive parameter tuning. These models, used in computer vision and graphics, have become important in tasks such as creating synthetic data and assisting multimodal models. However, they struggle with high computational demands. Quantum machine learning (QML) offers a solution to these challenges by leveraging quantum mechanics to improve efficiency in machine learning tasks.
Research on quantum diffusion models for imaging is limited, with the Quantum Denoising Diffusion Probabilistic Models (QDDPM) by Dohun Kim et al. being the only notable method. This model uses a single circuit design with shared and time-staggered layers, achieving spatial efficiency by requiring only log2 (pixels) qubits. It addresses the vanishing gradient problem through a restricted circuit depth and employs special unitary (SU) gates for entanglement. However, its parameter efficiency is low, and while it produces recognizable images, it lacks details compared to the original images.
Researchers from LMU Munich have introduced two quantum diffusion models, namely the Q-Dense and Quantum U-Net (QU-Net) architectures. These models are designed to increase the effectiveness of diffusion-based imaging models. The Q-Dense model uses a dense quantum circuit (DQC) with extensive entanglement between qubits. Classical U-Nets inspire the QU-Net and incorporate quantum principles in their architecture.
DQC uses amplitude embedding for input and angle embedding for class orientation, resulting in #layers×3×#qubits trainable parameters. QU-Net employs mask coding for labels and adapts to the quantum context. The unique “unitary single sampling” approach allows the creation of synthetic images in a single step by combining the iterative diffusion process on a unitary matrix U. Experiments were performed using MNIST, Fashion MNIST and CIFAR10 datasets to evaluate the effectiveness of the quantum models. .
In MNIST Digits experiments, the Q-Dense model with 47 layers and 7 qubits significantly outperformed classical networks with 1000 parameters, especially with τ values ranging between 3 and 5. It achieved FID scores of around 100, about 20 points better than classic models. For the painting tasks, the DQC produced consistent samples with minor artifacts. However, the MSE scores of the quantum models were only marginally lower than those of the classical networks with twice the number of parameters. Overall, the quantum models demonstrated effective knowledge transfer and satisfactory results in painting without specific training for these tasks.
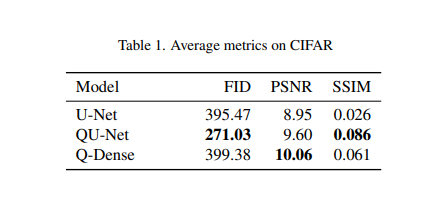
The research successfully introduced quantum denoising diffusion models, offering a new approach to imaging that takes advantage of quantum computing. The Q-Dense and QU-Net models and the unitary single sampling approach outperformed existing classical and quantum models in image generation. This method bridges the gap between quantum diffusion and classical consistency models, potentially accelerating imaging.
Review the Paper. All credit for this research goes to the researchers of this project. Also, don't forget to follow us on Twitter. Join our 36k+ ML SubReddit, 41k+ Facebook community, Discord channeland LinkedIn Grabove.
If you like our work, you will love our Newsletter..
Don't forget to join our Telegram channel
Nikhil is an internal consultant at Marktechpost. He is pursuing an integrated double degree in Materials at the Indian Institute of technology Kharagpur. Nikhil is an ai/ML enthusiast who is always researching applications in fields like biomaterials and biomedical science. With a strong background in materials science, he is exploring new advances and creating opportunities to contribute.
<!– ai CONTENT END 2 –>