Avatar technology has become ubiquitous on platforms such as Snapchat, Instagram and video games, improving user engagement by replicating human actions and emotions. However, the search for a more immersive experience led researchers from Goal and BAIR introduce “Audio2Photorealistic”, an innovative method to synthesize photorealistic avatars capable of maintaining natural conversations.
Imagine engaging in a telepresent conversation with a friend represented by a photorealistic 3D model, dynamically expressing emotions aligned with your speech. The challenge lies in overcoming the limitations of textureless meshes, which fail to capture subtle nuances such as gaze or smile, resulting in a robotic and strange interaction (see Figure 1, half). The research aims to close this gap, presenting a method to generate photorealistic avatars based on the audio of a dyadic conversation.
The approach involves synthesizing various high-frequency gestures and expressive facial movements synchronized with speech. Leveraging both a VQ-based autoregressive method and a diffusion model for the body and hands, the researchers strike a balance between frame rate and motion details. The result is a system that generates photorealistic avatars capable of transmitting complex facial, body and hand movements in real time.
To support this research, the team presents a unique multi-view conversational dataset, which provides a photorealistic reconstruction of long, unscripted conversations. Unlike previous data sets focused on upper body or facial movement, this data set captures the dynamics of interpersonal conversations and offers a more complete understanding of conversational gestures.
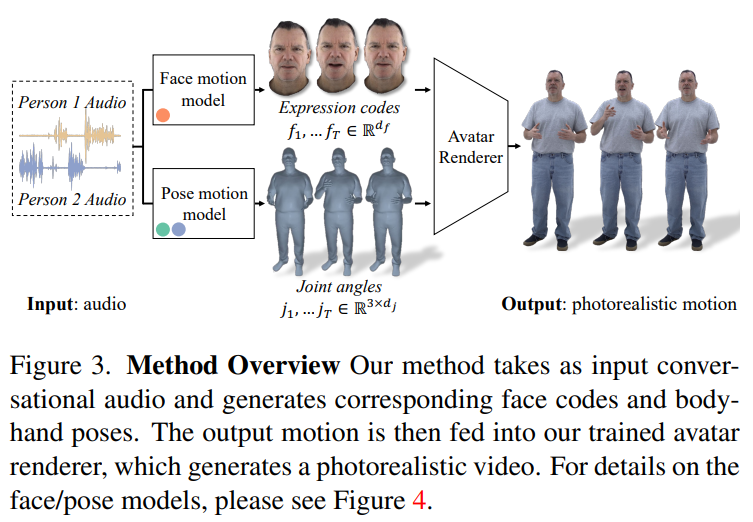
The system uses a two model (shown in Figure 3) approach to the synthesis of face and body movement, each addressing the unique dynamics of these components. He facial movement model (Figure 4(a)), a diffusion model conditioned on audio input and lip vertices, focuses on generating facial details consistent with speech. Unlike, the body movement model uses an audio-conditioned autoregressive transformer to predict approximate guiding postures (Figure 4(b)) at 1 fps, then refined by the diffusion model (Figure 4(c)) for diverse but plausible body movements.
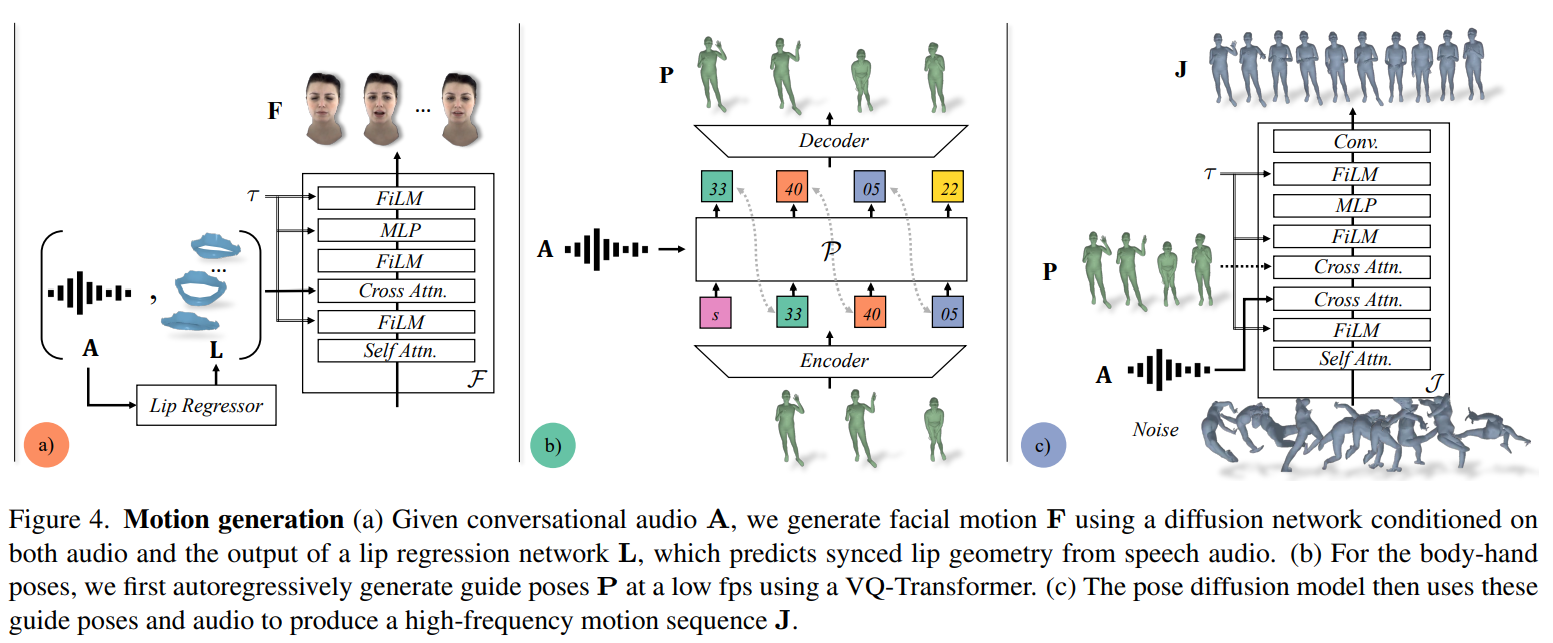
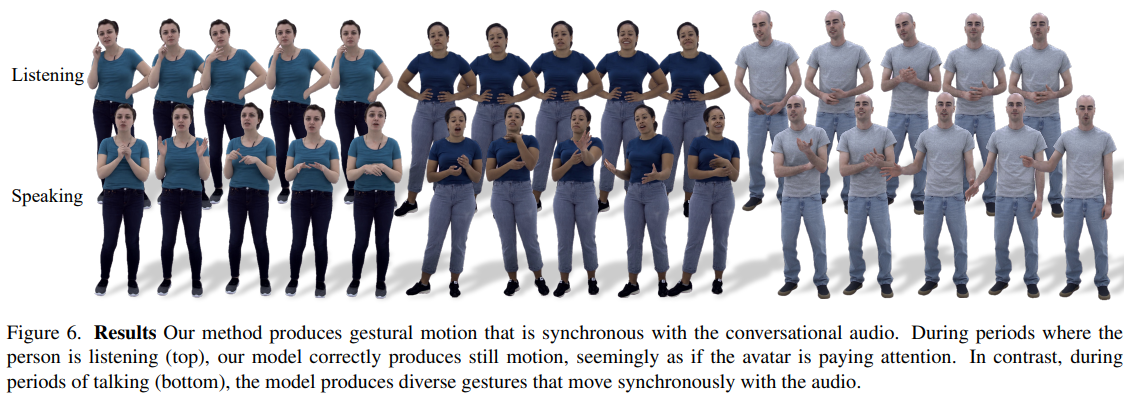
The evaluation demonstrates the effectiveness of the model (shown in Figure 6) in generating realistic and diverse conversational movements, surpassing several baselines. Photorealism is crucial for capturing subtle nuances, as highlighted in perceptual evaluations. The quantitative results show the method's ability to balance realism and diversity, outperforming previous work in terms of motion quality.
While the model excels at generating convincing and plausible gestures, it works with short-range audio, which limits its long-term language understanding ability. Additionally, ethical considerations of consent are addressed by including only consenting participants in the data set.
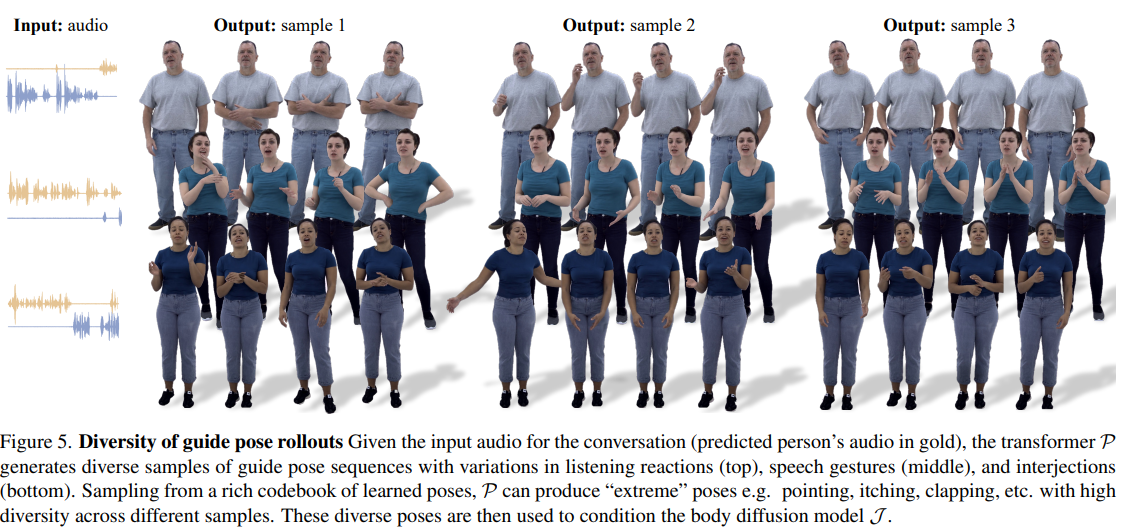
In conclusion, “Audio2Photoreal” represents a significant leap in the synthesis of conversational avatars, offering a more immersive and realistic experience. The research not only presents a novel dataset and methodology, but also opens avenues to explore ethical considerations in photorealistic motion synthesis.
Review the Paper and Project. All credit for this research goes to the researchers of this project. Also, don't forget to follow us on Twitter. Join our 36k+ ML SubReddit, 41k+ Facebook community, Discord channeland LinkedIn Grabove.
If you like our work, you'll love our newsletter.
Vineet Kumar is a Consulting Intern at MarktechPost. She is currently pursuing her bachelor's degree from the Indian Institute of technology (IIT), Kanpur. He is a machine learning enthusiast. He is passionate about research and the latest advances in Deep Learning, Computer Vision and related fields.
<!– ai CONTENT END 2 –>