Physics-based character animation, a field at the intersection of computer graphics and physics, aims to create realistic and responsive character movements. This domain has long been the basis of digital animation, which seeks to replicate the complexities of real-world movement in a virtual environment. The challenge lies in the technical aspects of animation and capturing the subtleties and fluidity of natural human movement.
A major challenge in this area is achieving intuitive control over animations through high-level human instructions. Traditional methods often fail to seamlessly integrate these instructions with the dynamic and complex nature of physical environments. Existing techniques, including motion tracking and language-conditioned controllers, offer some levels of control. However, they need to be improved in handling the intricate nuances of human language and the varied scenarios of physical simulations. This gap makes it difficult to create faithfully instructional and physically realistic animations.
Researchers from S-Lab Nanyang Technological University, National University of Singapore and Dyson Robot Learning Lab present InsActor. This generative framework uses advances in diffusion-based human motion models. This framework is a significant leap in creating instruction-based animations for physics-based characters. InsActor excels at capturing the intricate relationship between complex human instructions and character movements, a feat that has been challenging to achieve with existing technologies.
Digging deeper into InsActor's methodology reveals its innovative two-tier approach. At a high level, InsActor employs a state diffusion policy to generate actions in the character's joint space. This policy is conditional on human participation, allowing movement plans to be created that respond to a variety of instructions. The lower level of the InsActor architecture involves a skill discovery process, which addresses the challenge of invalid states and infeasible state transitions often encountered in planned moves. This process maps each state transition to an embodied ability within a compact latent space. Combining these two levels allows InsActor to interpret human instructions into coherent movement plans and ensure that these plans are physically plausible and executable within the simulated environment.
In terms of performance, InsActor demonstrates impressive results. The framework significantly outperforms existing methods in generating physically plausible animations that follow high-level human instructions. InsActor's versatility is shown in its ability to handle various tasks, including motion generation and instruction-based waypoint heading. Its performance is characterized by its adaptability to different animation scenarios and its ability to handle complex instruction sets, which has been a challenge for previous methods.
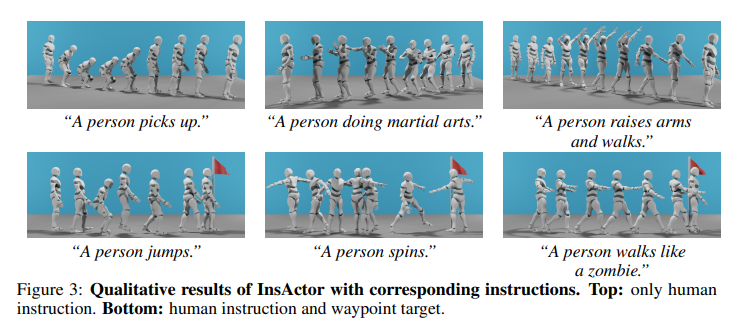
In conclusion, InsActor represents an innovative development in physics-based character animation. It addresses a long-standing challenge in the field by bridging the gap between high-level human instructions and generating realistic character movements. Its innovative approach to interpreting and executing complex instructions in realistic animations opens up new possibilities in diverse applications, ranging from virtual reality experiences to advanced animation in film. The framework's ability to translate the richness of human language into fluidity of motion sets a new standard in digital animation.
Review the Paper. All credit for this research goes to the researchers of this project. Also, don't forget to join. our SubReddit of more than 35,000 ml, 41k+ Facebook community, Discord channel, LinkedIn Graboveand Electronic newsletterwhere we share the latest news on ai research, interesting ai projects and more.
If you like our work, you'll love our newsletter.
Sana Hassan, a consulting intern at Marktechpost and a dual degree student at IIT Madras, is passionate about applying technology and artificial intelligence to address real-world challenges. With a strong interest in solving practical problems, she brings a new perspective to the intersection of ai and real-life solutions.
<!– ai CONTENT END 2 –>