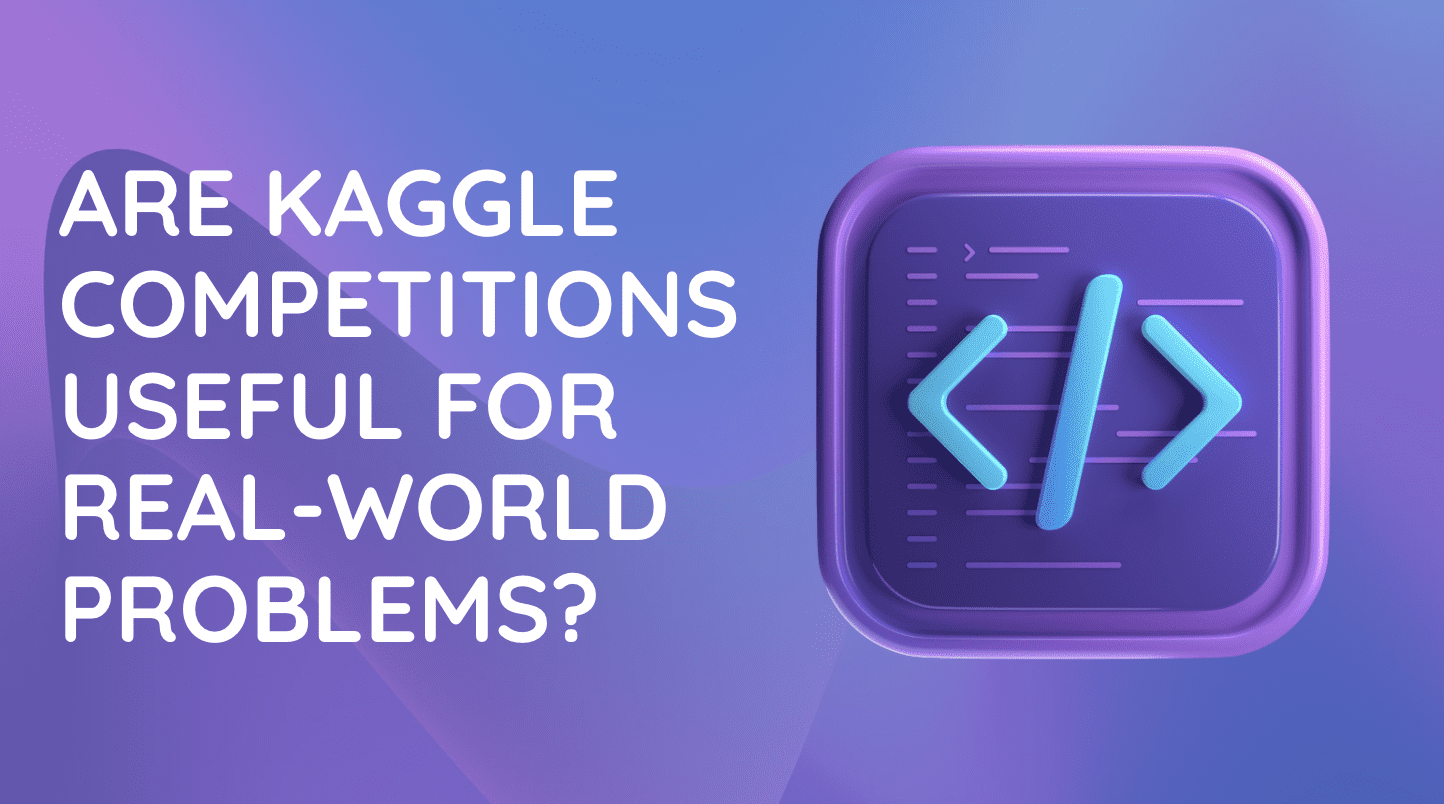
Image by author
If you are getting into the technology industry or have been in it for a while, you will have heard of kaggle. It is a data science competition platform aimed at data scientists and machine learning enthusiasts.
The online platform aims to guide users in their professional careers to achieve their goals on their journey into data science or machine learning with the powerful tools and resources it provides.
As people try to improve and progress in their careers, you will see many people flocking to online courses, contests, and more. Kaggle is an incredible platform for people to challenge themselves, throw themselves into the deep end, and come face to face with the reality of their abilities.
Many people have created projects on the Kaggle platform and had access to a variety of data sets, with great resources such as free access to NVIDIA K80 GPUs in the kernels. The question we are going to ask today is “Are Kaggle competitions useful for real-world problems?”
A question was asked on Quora: Should I spend my time participating in Kaggle or working on interesting side projects? Which will be most beneficial for my career?
With a variety of answers, but as you can see in the screenshot of the image below the answer to your question is explained.
Let’s discuss whether Kaggle competitions are useful for real-world problems.
So we’ve talked about how Kaggle competitions help your learning journey and how some aspects reflect what happens in the real world. But is it useful for real-world problems? The general answer is no. Let me explain why in different aspects.
Identifying the problem
As a data scientist or machine learning engineer, your first task is to identify the problem or understand the current business problem that needs to be solved. For example, you may need to distinguish whether the problem type is supervised or unsupervised, decide which model you will use, etc.
This is one of the most important decisions you will make. If you don’t have a general understanding of the organization, it will make your life more difficult as you won’t be able to identify the root of the problem.
Real world: Identify the problem or understand the current business problem that needs to be solved
kaggle: You are given a detailed description of the problem and what you are evaluating.
Data preparation
With Kaggle competitions, the contest host provides you with prepared datasets along with a detailed description of the problem at hand. This saves data scientists a lot of time collecting, cleaning and structuring data, which happens in the real world.
Some believe that Kaggle spoon-fed new data scientists and machine learning engineers the data provided, allowing them to get straight to work. Data preparation is an important phase in the data science lifecycle and Kaggle has proven to do it all for users.
In the real world, your company may or may not provide you with data. If not, you’ll have to collect it yourself, make sure it aligns with the problem at hand, clean it up, and structure it. You are also allowed to freely search for additional relevant data, whereas on Kaggle you are restricted to using external data.
Real world: Data collection and preparation will help you solve the identified problem.
kaggle: Provides you with prepared data that is aligned with a detailed description of the problem at hand.
Feature Engineering
Once you have your data and it’s all shiny and clean, your next step as a data scientist is to jump in and become a feature engineer. Feature engineering is rooted in the problem at hand, what you’re trying to solve, and how you’re going to solve it.
With this, you will have a better understanding of how much time you will spend on feature engineering and whether other elements of the data science lifecycle are more important.
However, in Kaggle competitions, feature engineering plays an important role in finishing on the leaderboard. Yes, feature engineering is part of the data science lifecycle, but real-world data science projects focus more on the factor that drives your model, rather than small incremental gains.
Real world: The level of feature engineering depends on the problem at hand and where it is focused.
kaggle: Feature engineering level is used as an incentive to move up the ranks.
Modeling
Choosing the right model is based on many factors, such as the explainability of the model, the data being used, the performance of the model, and the launch of the model into production. All of these are in line with your problem at hand, as it is up to you to determine which one suits your business needs.
While on Kaggle, users are more concerned about which model works best and processes the data they are working with. The factors taken into account when choosing your model are much less realistic than those addressed in the real world.
Real world: Choose the right model based on a variety of factors related to your business problem at hand.
kaggle: Choose the right model based on performance while participating in a competition.
Validation
Validation is an aspect in which both Kaggle and the real world show similarity. Validating the performance of your model is an important aspect as it allows you to explore where you can make changes to improve your model and shows you if your model has value in the real world.
Kaggle competitions show you how building a robust model is useful in the real world.
Model in production
In the real world, most of the models you build are destined to go into production. This is because there is a purpose behind his model: he was trying to solve a real-world problem. Your model, in one way or another, will find a way to integrate into the business process to assist in future decision making.
On the other hand, when you participate in a Kaggle competition, your number one concern is where you placed on the leaderboard and not how your model will be implemented and used in the future.
Real world: Every model you build has a purpose and you want to bring it to production to solve your business’s current problem.
Kaggle: The overall goal of creating your model was to see where you ranked on the leaderboard and what you can do better next time compared to your competitors.
Kaggle teaches you a lot. Through Kaggle competitions and working on different tasks and data sets, you can learn a lot. Personally, I don’t think there is anything wrong with learning more and facing challenges. Simply learn how to overcome these challenges by reflecting on your weaknesses and how to turn them into strengths.
Would you rather know more before landing your dream job or not know? The answer is quite simple and depends on what you want from your career.
Kaggle competitions show you how your model is performing, which is good for your learning journey. As indicated in the screenshot above, you might assume that your model’s performance is really good, only to realize that it wasn’t as good as others from the same competition.
That being said, Kaggle competitions propel you along your learning journey, allowing you to compete with people around the world and improve your skills as an individual.
In the real world, when you work on projects, you are given deadlines. Deadlines help you stay on top of your tasks, which are aligned with the organization’s business plan. Every deadline is the start of a new project.
Kaggle competitions have deadlines that reflect what your daily tasks would normally be like. This is a great way to understand how your time is being used and to overcome procrastination.
According to the points we reviewed, the usefulness of Kaggle competitions depends exclusively on individuals. Yes, every aspect of a Kaggle competition may not reflect what happens in the real world, but many of us can say the same about some of the things we learned in school.
Is that enough to say it’s not useful for real world problems?
Kaggle competitions provide you with a great learning experience and allow you to explore skills you may have never focused on before. There is a lot of experience that can come from Kaggle competitions that you can use in your career later.
nisha arya is a data scientist and freelance technical writer. She is particularly interested in providing professional data science advice or tutorials and theory-based insights into data science. She also wants to explore the different ways in which artificial intelligence can benefit the longevity of human life. A great student looking to expand her technological knowledge and writing skills, while she helps guide others.