Introduction
Welcome to the world of Stable Diffusion techniques for creating custom images, where creativity knows no bounds. In the realm of ai-powered image generation, DreamBooth emerges as a game-changer, granting individuals the remarkable ability to craft bespoke visuals tailored to their unique ideas. Stable Diffusion breathes life into the creative process, elevating ordinary images to extraordinary heights.
In this exploration, we’ll introduce you to DreamBooth, a groundbreaking platform that empowers users to transform ordinary images into extraordinary works of art through Stable Diffusion. Together, we’ll unravel the magic behind Stable Diffusion and discover how it can manipulate and enhance images in captivating ways.
Learning Objectives:
- Learn Stable Diffusion for text-to-image generation.
- Master DreamBooth’s customization with minimal images, name token selection, and captioning.
- Apply DreamBooth for hands-on fine-tuning, image selection, aspect ratio matching, and effective naming.
Understanding the Power of Stable Diffusion in Image Generation
Stable Diffusion is not just another image generation technique; it’s a revolutionary approach that brings text-to-image conversion to life. It enables the transformation of textual descriptions into visually stunning and high-quality images. Imagine typing a description like “a serene mountain lake at dawn” and having it transformed into a lifelike image capturing the essence of that scene.
In the realm of generative ai, Stable Diffusion has made a significant impact by providing remarkable edge preservation, creating images that exhibit incredible detail and realism. It’s a technique inspired by fluid mechanics, simulating how gases diffuse, and it has changed the game regarding image quality.
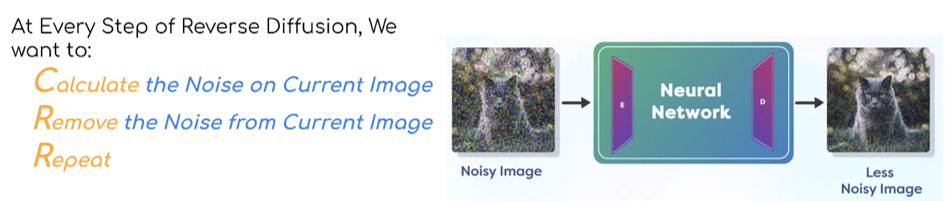
The Intricacies of DreamBooth’s Fine-Tuning Process
DreamBooth takes the power of Stable Diffusion and places it in the hands of users, allowing them to fine-tune pre-trained models to create custom images based on their unique concepts. What sets DreamBooth apart is its ability to achieve this customization with just a handful of images—typically 10 to 20—making it accessible and efficient.
The core idea behind DreamBooth is to teach the model a new concept, and this is done through a process called fine-tuning. You start with a pre-existing Stable Diffusion model (the red figure) and provide it with a set of images that represent your concept. This could be anything from images of your pet dog to a specific artistic style. DreamBooth then guides the model to generate images that align with your concept, using a designated token (often denoted as ‘V’ in rectangular braces) to represent your concept.
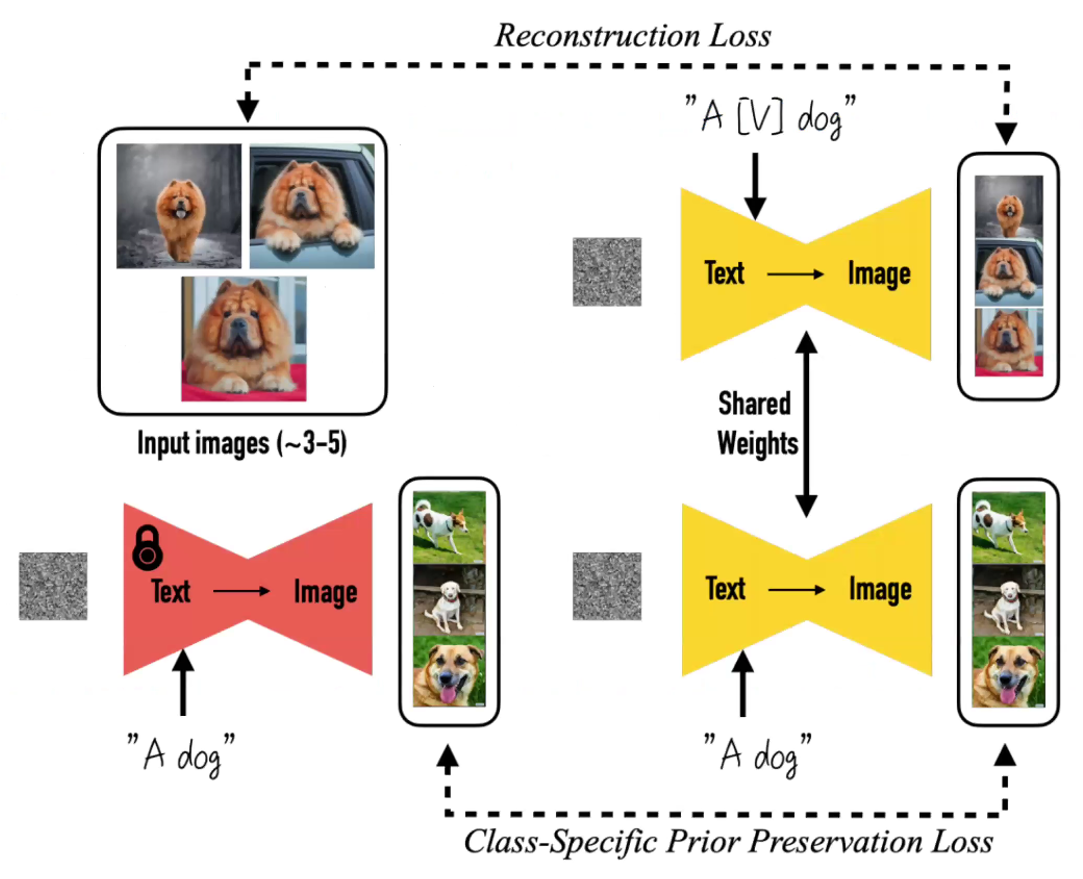
Name Token Selection and Custom Concept Generation
Selecting the right name token for your concept is crucial for successful fine-tuning. The name token serves as a unique identifier for your concept within the model. Choosing a name that won’t clash with existing concepts already known to the model is important. Here are some guidelines:
- Uniqueness: Ensure your name token is unique and unlikely to be associated with pre-existing concepts in the model’s knowledge base.
- Length: Longer tokens, ideally five letters or more, are preferable. Short, common tokens may lead to confusion.
- Testing: Before fine-tuning, test your chosen token on the base model to see what kind of images it generates. This helps you understand the model’s existing interpretation of the token.
- Vowel Removal: Consider dropping vowels from the token name. This can reduce the likelihood of conflicts with existing concepts.
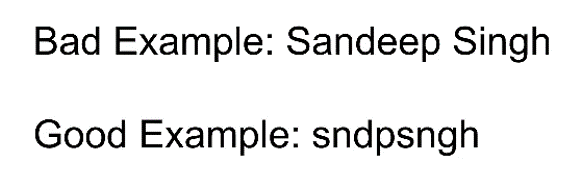
Hands-On Experience with DreamBooth: Fine-Tuning for Custom Images
Now that you have a grasp of the fundamentals let’s dive into a practical demonstration of how DreamBooth works. We’ll fine-tune a Stable Diffusion model with a set of custom images and create stunning, personalized visual content. Whether you’re an artist looking to imbue your style into your creations or a hobbyist eager to explore the potential of Stable Diffusion, this hands-on experience will empower you to unlock the full potential of DreamBooth.
Selecting and Preparing Your Images
The key to successful image personalization with DreamBooth lies in your selection and preparation of images. Unlike off-the-shelf Stable Diffusion models, DreamBooth requires a specific approach to make it understand and generate images according to your concepts. Here are some tips to help you select and prepare your images to personalize the model better.
- Number of Images: While the original papers may suggest using just 3 to 5 images for training, it’s often more practical to start with 20 to 25 images. Remember, these models are highly demanding when it comes to training, and a larger dataset helps them learn more effectively.
- Variation in Images: Don’t limit yourself to similar images. The key is to provide variations, such as different backgrounds, clothing, lighting conditions, and poses. This diversity ensures that the model can generalize your concept across various settings.
- Aspect Ratio: Ensure that the aspect ratio of your images matches that of the pre-trained Stable Diffusion model you plan to use. Consistency in aspect ratios helps in the fine-tuning process.
- Image Resizing Made Easy: A handy tool for resizing and cropping images to your desired aspect ratio is ‘big image resizing made easy’ (birme.net). This user-friendly website allows you to upload images and easily select the size and aspect ratio you need.
- File Naming: After resizing, make sure to rename your files with a common prefix representing your concept. This consistency helps DreamBooth understand and differentiate between concepts during training.
Running DreamBooth
Once you’ve prepared your images, running DreamBooth becomes surprisingly straightforward. You don’t need extensive coding skills; instead, you’ll mostly interact with the Jupyter Notebook interface provided.
How to Run DreamBooth
- Start the Training
Using the provided DreamBooth shell, initiate the training process. The default number of training steps is around 1,500, but you can adjust it as needed.
- Wait for Completion
The training process may take a few minutes or longer depending on your hardware. Be patient and let the model learn your concept.
- Testing the Model
After training, you can test your model. DreamBooth uses Gradio-based deployment, providing you with a URL for interaction.
- Real-Time Customization
While DreamBooth doesn’t allow real-time personalization during inference, this area has ongoing developments. Some companies are working on ai models that quickly adapt to new subjects or concepts during conversations.
The Power of Captioning
Captioning plays a crucial role in DreamBooth to fine-tune and guide the model’s understanding of your concept. It helps the model differentiate between core features and additional elements. For example, if you’re training a face with a hat, including a caption like “Yvnsngh wearing a hat” explicitly defines the concept. Captioning ensures that the model generates images that align with your precise vision.
Stable Diffusion vs. DreamBooth: Key Differences
It’s essential to distinguish between Stable Diffusion and DreamBooth:
- Stable Diffusion: It is ideal for generating general images but lacks personalization. Moreover, it requires a large amount of training data and doesn’t easily adapt to specific concepts.
- DreamBooth: It is tailored for personalization and customization in image generation. It requires a much smaller dataset and allows the generation of images with specific subjects in various scenes, poses, and views.
The Future of Image Generation
As we look ahead, the field of ai-generated images is evolving rapidly. Keeping up with ongoing research is crucial. While there’s no centralized repository for the latest developments, you can follow experts and organizations on social media platforms like Twitter and LinkedIn to stay updated.
The next year promises exciting advancements in this technology. With innovations happening at an unprecedented pace, we can expect more accessible and powerful tools for image personalization, making it possible for anyone to unleash their creativity with ai-generated visuals.
Conclusion
Stable Diffusion techniques, exemplified by DreamBooth, have revolutionized image generation. They empower users to create custom visuals effortlessly. Stable Diffusion’s remarkable realism and DreamBooth’s efficient customization process make this technology accessible to all. In this article, we’ve explored DreamBooth’s fine-tuning intricacies, image preparation, and running process, highlighting its unique capabilities for personalization. Looking forward, the world of ai-generated images is evolving rapidly, promising more accessible and powerful tools for creativity. Embrace the enchanting magic of DreamBooth and unlock your creative potential in the ever-evolving landscape of ai-generated visuals.
Key Takeaways:
- Stable Diffusion transforms text into life-like images with remarkable realism.
- DreamBooth customizes Stable Diffusion models with a few images and a unique name token for personalized creations.
- Success with DreamBooth depends on diverse images, matching aspect ratios, and effective captioning to guide the model’s understanding.
Frequently Asked Questions
Ans. Stable Diffusion is ideal for generating general images but lacks personalization, requiring extensive training data. In contrast, DreamBooth is tailored for customization, demands a smaller dataset, and excels in generating images with specific subjects in various scenarios.
Ans. While the original papers suggest 3 to 5 images, practicality often dictates starting with 20 to 25 images for effective training, ensuring the model learns your concept thoroughly.
Ans. Currently, DreamBooth doesn’t support real-time personalization during inference. However, there are ongoing developments in this area, with some companies working on ai models capable of adapting to new subjects or concepts during conversations.
About the Author: Sandeep Singh
Sandeep Singh epitomizes leadership in the domain of applied artificial intelligence (ai) and Computer Vision, particularly within the geospatial industry of Silicon Valley. He spearheads the advancement of pioneering technologies devised to capture, dissect, and comprehend satellite imagery, visual data, and geolocation information. Possessing profound knowledge of the intricacies of computer vision algorithms, machine learning mechanisms, image processing techniques, and applied ethics, Sandep’s role encompasses the conceptualization and manifestation of avant-garde solutions.
DataHour Page: https://community.analyticsvidhya.com/c/datahour/datahour-dreambooth-stable-diffusion-for-custom-images
LinkedIn: ai/” target=”_blank” rel=”noreferrer noopener”>https://www.linkedin.com/in/san-deeplearning-ai/