Recent advancements in deep neural networks have enabled new approaches to address anatomical segmentation. For instance, state-of-the-art performance in the anatomical segmentation of biomedical images has been attained by deep convolutional neural networks (CNNs). Conventional strategies adopt standard encoder-decoder CNN architectures to predict pixel-level segmentation using annotated datasets. While this approach suits scenarios where the topology isn’t preserved across individuals, such as lesion segmentation, it might not be ideal for anatomical structures with regular topology. Deep segmentation networks are often trained to minimize pixel-level loss functions, which might not ensure anatomical plausibility due to insensitivity to global shape and topology. This can result in artifacts like fragmented structures and topological inconsistencies.
To mitigate these issues, incorporating prior knowledge and shape constraints becomes crucial, especially for downstream tasks like disease diagnosis and therapy planning. Instead of dense pixel-level masks, alternatives like statistical shape models or graph-based representations offer a more natural way to include topological constraints. Graphs, in particular, provide a means to represent landmarks, contours, and surfaces, enabling the incorporation of topological correctness. Geometric deep learning has extended CNNs to non-Euclidean domains, facilitating the development of discriminative and generative models for graph data. These advancements enable accurate predictions and the generation of realistic graph structures aligned with specific distributions.
Following the mentioned considerations, the novel HybridGNet architecture has been introduced to exploit the benefits of landmark-based segmentation in standard convolutions for image feature encoding.
The architecture overview is presented in the figure below.
HybridGNet is coupled with generative models based on graph neural networks (GCNNs) to create anatomically accurate segmented structures. It processes input images through standard convolutions and generates landmark-oriented segmentations by sampling a “bottleneck latent distribution,” a compact encoded representation containing the essential information about the image. Sampling from this distribution allows the model to create diverse and plausible segmented outputs based on the encoded image features. After sampling, follows reshaping and graph domain convolutions.
Additionally, under the hypothesis that local image features may help to produce more accurate estimates of landmark positions, an Image-to-Graph Skip Connection (IGSC) module is presented. Analogous to UNet skip connections, the IGSC module, combined with graph unpooling operations, allows feature maps to flow from encoder to decoder, thus enhancing the model’s capacity to recover fine details.
Sample outcome results selected from the study are depicted in the image below. These visuals provide a comparative overview between HybridGNet and state-of-the-art approaches.
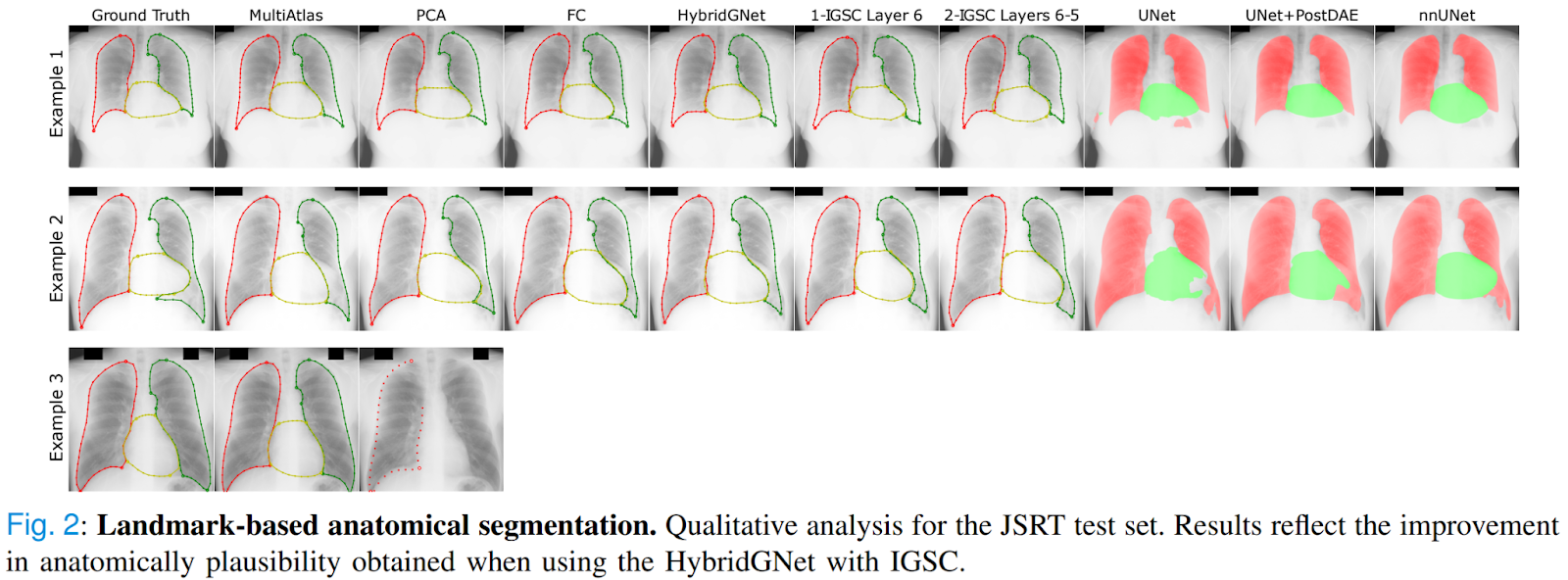
This was the summary of HybridGNet, a novel AI encoder-decoder neural architecture that leverages standard convolutions for image feature encoding and graph convolutional neural networks (GCNNs) to decode plausible representations of anatomical structures. If you are interested and want to learn more about it, please feel free to refer to the links cited below.
Check out the Paper and Github. All Credit For This Research Goes To the Researchers on This Project. Also, don’t forget to join our 29k+ ML SubReddit, 40k+ Facebook Community, Discord Channel, and Email Newsletter, where we share the latest AI research news, cool AI projects, and more.
If you like our work, you will love our newsletter..
Daniele Lorenzi received his M.Sc. in ICT for Internet and Multimedia Engineering in 2021 from the University of Padua, Italy. He is a Ph.D. candidate at the Institute of Information Technology (ITEC) at the Alpen-Adria-Universität (AAU) Klagenfurt. He is currently working in the Christian Doppler Laboratory ATHENA and his research interests include adaptive video streaming, immersive media, machine learning, and QoS/QoE evaluation.