In recent times, there has been a growing fascination with the task of acquiring a 3D generative model from 2D images. With the advent of Neural Radiance Fields (NeRF), the quality of images produced from a 3D model has witnessed a significant advancement, rivaling the photorealism achieved by 2D models. While specific approaches focus solely on 3D representations to ensure consistency in the third dimension, this often comes at the expense of reduced photorealism. More recent studies, however, have shown that a hybrid approach can overcome this limitation, resulting in intensified photorealism. Nonetheless, a notable drawback of these models lies in the intertwining of scene elements, including geometry, appearance, and lighting, which hinders user-defined control.
Various approaches have been proposed to untangle this complexity. However, they demand collections of multiview images of the subject scene for effective implementation. Unfortunately, this requirement poses difficulties when dealing with images taken under real-world conditions. While some efforts have relaxed this condition to encompass pictures from different scenes, the necessity for multiple views of the same object persists. Furthermore, these methods lack generative capabilities and necessitate individual training for each distinct object, rendering them unable to create novel objects. When considering generative methodologies, the interlaced nature of geometry and illumination remains challenging.
The proposed framework, known as FaceLit, introduces a method for acquiring a disentangled 3D representation of a face exclusively from images.
An overview of the architecture is presented in the figure below.
At its core, the approach revolves around constructing a rendering pipeline that enforces adherence to established physical lighting models, similar to prior work, tailored to accommodate 3D generative modeling principles. Moreover, the framework capitalizes on readily available lighting and pose estimation tools.
The physics-based illumination model is integrated into the recently developed Neural Volume Rendering pipeline, EG3D, which uses tri-plane components to generate deep features from 2D images for volume rendering. Spherical Harmonics are utilized for this integration. Subsequent training focuses on realism, taking advantage of the framework’s inherent adherence to physics to generate lifelike images. This alignment with physical principles naturally facilitates the acquisition of a disentangled 3D generative model.
Crucially, the pivotal element enabling the methodology is the integration of physics-based rendering principles into neural volume rendering. As previously indicated, the strategy is designed for seamless integration with pre-existing, readily available illumination estimators by leveraging Spherical Harmonics. Within this framework, the diffuse and specular aspects of the scene are characterized by Spherical Harmonic coefficients attributed to surface normals and reflectance vectors. These coefficients encompass diffuse reflectance, material specular reflectance, and normal vectors, which are generated through a neural network. This seemingly straightforward setup, however, effectively untangles illumination from the rendering process.
The proposed approach is implemented and tested across three datasets: FFHQ, CelebA-HQ, and MetFaces. According to the authors, this yields state-of-the-art FID scores, positioning the method at the forefront of 3D-aware generative models. Some of the results produced by the discussed method are reported below.
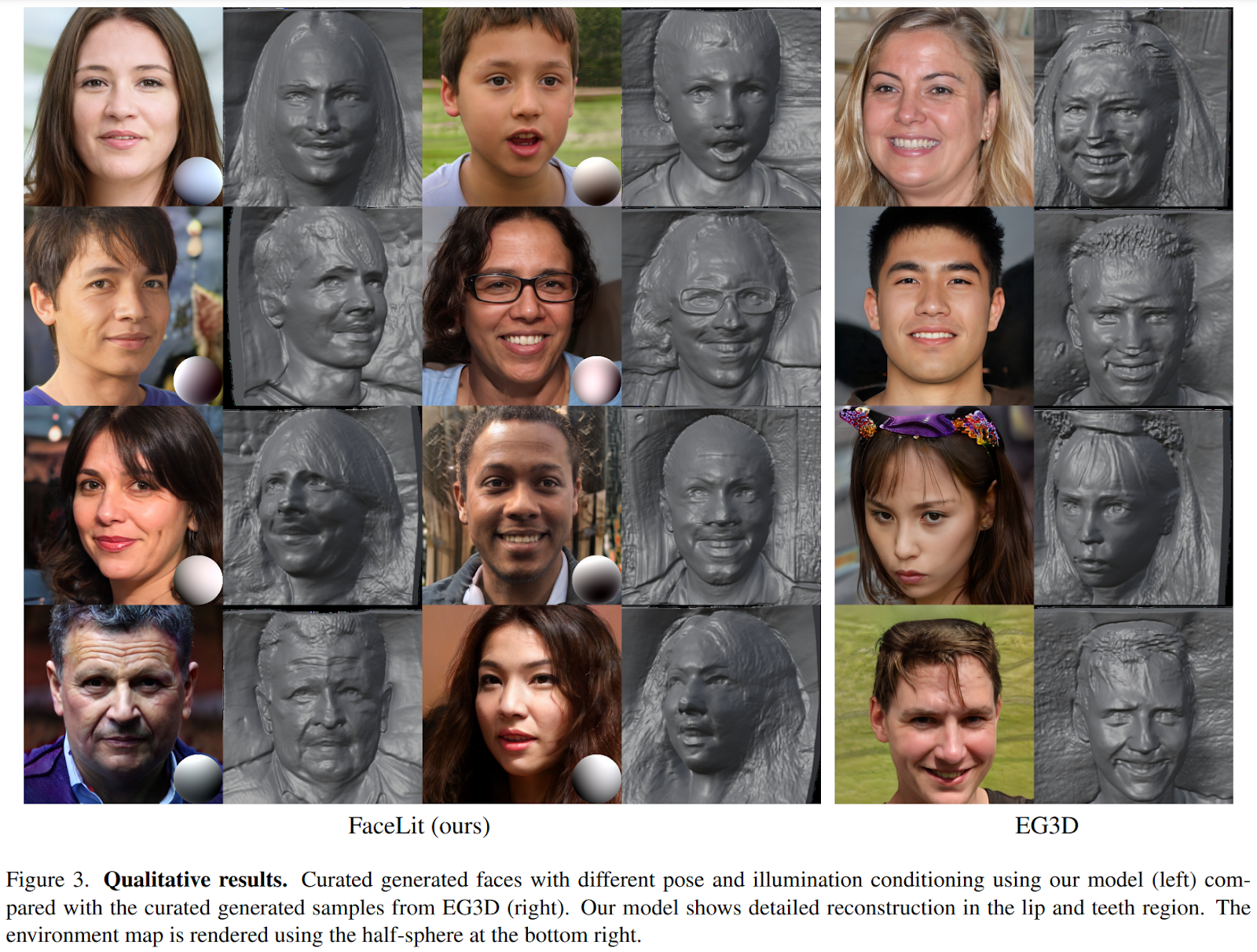
This was the summary of FaceLit, a new AI framework for acquiring a disentangled 3D representation of a face exclusively from images. If you are interested and want to learn more about it, please feel free to refer to the links cited below.
Check out the Paper and Github. All Credit For This Research Goes To the Researchers on This Project. Also, don’t forget to join our 28k+ ML SubReddit, 40k+ Facebook Community, Discord Channel, and Email Newsletter, where we share the latest AI research news, cool AI projects, and more.
Daniele Lorenzi received his M.Sc. in ICT for Internet and Multimedia Engineering in 2021 from the University of Padua, Italy. He is a Ph.D. candidate at the Institute of Information Technology (ITEC) at the Alpen-Adria-Universität (AAU) Klagenfurt. He is currently working in the Christian Doppler Laboratory ATHENA and his research interests include adaptive video streaming, immersive media, machine learning, and QoS/QoE evaluation.