Generative AI has gained significant interest in the computer vision community. Recent advances in text-based image and video synthesis, such as Text-to-Image (T2I) and Text-to-Video (T2V), spurred by the advent of diffusion models, have exhibited a remarkable fidelity and generative quality. These advances demonstrate considerable potential for image and video synthesis, editing, and animation. However, synthesized images/videos are still far from perfect, especially for human-centric applications like human dance synthesis. Despite the long history of human dance synthesis, existing methods suffer greatly from the gap between synthesized content and real-world dance settings.
Beginning in the era of generative adversarial networks (GANs), researchers have sought to extend the video-to-video transfer style to transfer dance moves from a source video to a target individual, often requiring adjustment. Human-specific fine on target. person.
Recently, a line of work leverages pre-trained diffusion-based T2I/T2V models to generate text message-conditioned dance images/videos. Such a coarse-grained condition dramatically limits the degree of controllability, making it nearly impossible for users to precisely specify anticipated themes, ie, human appearance, as well as dance moves, ie, human pose.
Although the introduction of ControlNet partially alleviates this problem by incorporating pose control with human geometry points, it is not clear how ControlNet can ensure the consistency of rich semantics, such as human appearance, in the reference image, due to its reliance on the text prompts. Furthermore, almost all existing methods trained on limited dance video data sets suffer from limited subject attributes or overly simplistic scenes and backgrounds. This leads to a poor generalization from zero shot to invisible compositions of human subjects, poses, and backgrounds.
To support real-life applications, such as the generation of user-specific short video content, the human dance generation must adhere to real-world dance scenarios. Therefore, the generative model is expected to synthesize human dance images/videos with the following properties: fidelity, generalization, and compositionality.
The generated images/videos must exhibit fidelity by preserving the appearance of human subjects and backgrounds consistent with the reference images while accurately following the provided pose. The model must also demonstrate generalizability by handling invisible human subjects, backgrounds, and poses without the need for human-specific adjustments. Lastly, the generated images/videos must show composition, allowing arbitrary combinations of human subjects, backgrounds, and poses from different images/videos.
In this sense, a novel approach called DISCO is proposed for the generation of human dance in real world settings. The overview of the approach is presented in the figure below.
DISCO incorporates two key designs: a novel model architecture with untangled control to improve fidelity and composition, and a pretraining strategy called human attributes pre-training for a better generalization. DISCO’s novel model architecture ensures that the generated dance images/videos faithfully capture the desired human subjects, backgrounds and poses while allowing for flexible composition of these elements. Additionally, untangled control enhances the model’s ability to maintain faithful rendering and adapt to various compositions. In addition, DISCO employs the human attributes pretraining strategy to strengthen the generalization of the model. This pre-training technique equips the model with the ability to handle invisible human attributes, allowing it to generate high-quality dance content that extends beyond the limitations of the training data. Overall, DISCO presents a comprehensive solution that combines a sophisticated model architecture with an innovative pre-training strategy, effectively addressing the challenges of human dance generation in real-world scenarios.
The results are presented below, along with a comparison of DISCO with the most advanced techniques for generating human dance.
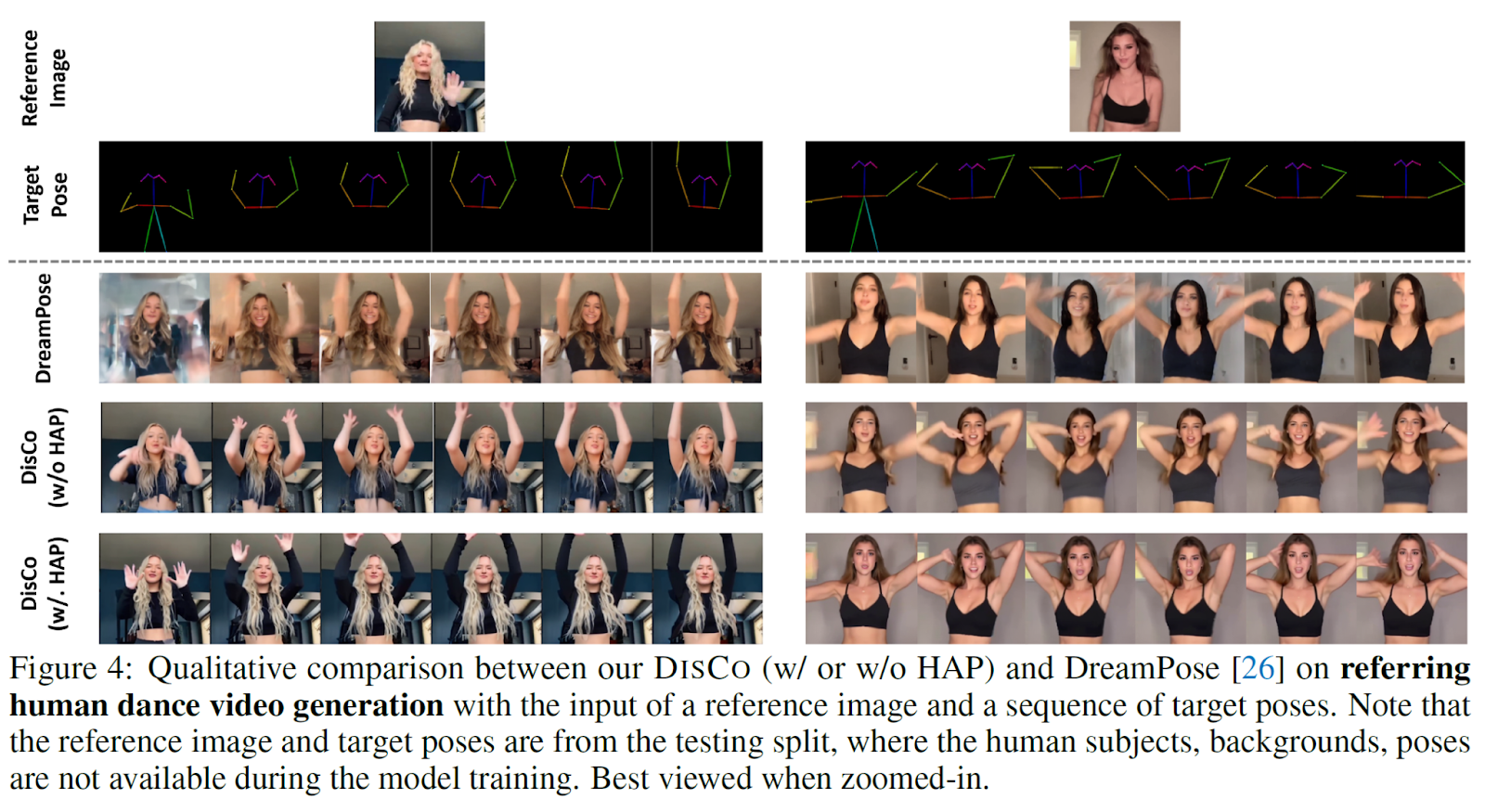
This was the brief for DISCO, a novel AI technique for generating human dance. If you are interested and would like to learn more about this job, you can find out more by clicking on the links below.
review the Paper, Project, and github link. Don’t forget to join our 26k+ ML SubReddit, discord channel, and electronic newsletter, where we share the latest AI research news, exciting AI projects, and more. If you have any questions about the article above or if we missed anything, feel free to email us at [email protected]
🚀 Check out over 800 AI tools at AI Tools Club
Daniele Lorenzi received his M.Sc. in ICT for Internet and Multimedia Engineering in 2021 from the University of Padua, Italy. He is a Ph.D. candidate at the Institute of Information Technology (ITEC) at the Alpen-Adria-Universität (AAU) Klagenfurt. He currently works at the Christian Doppler ATHENA Laboratory and his research interests include adaptive video streaming, immersive media, machine learning and QoS / QoE evaluation.