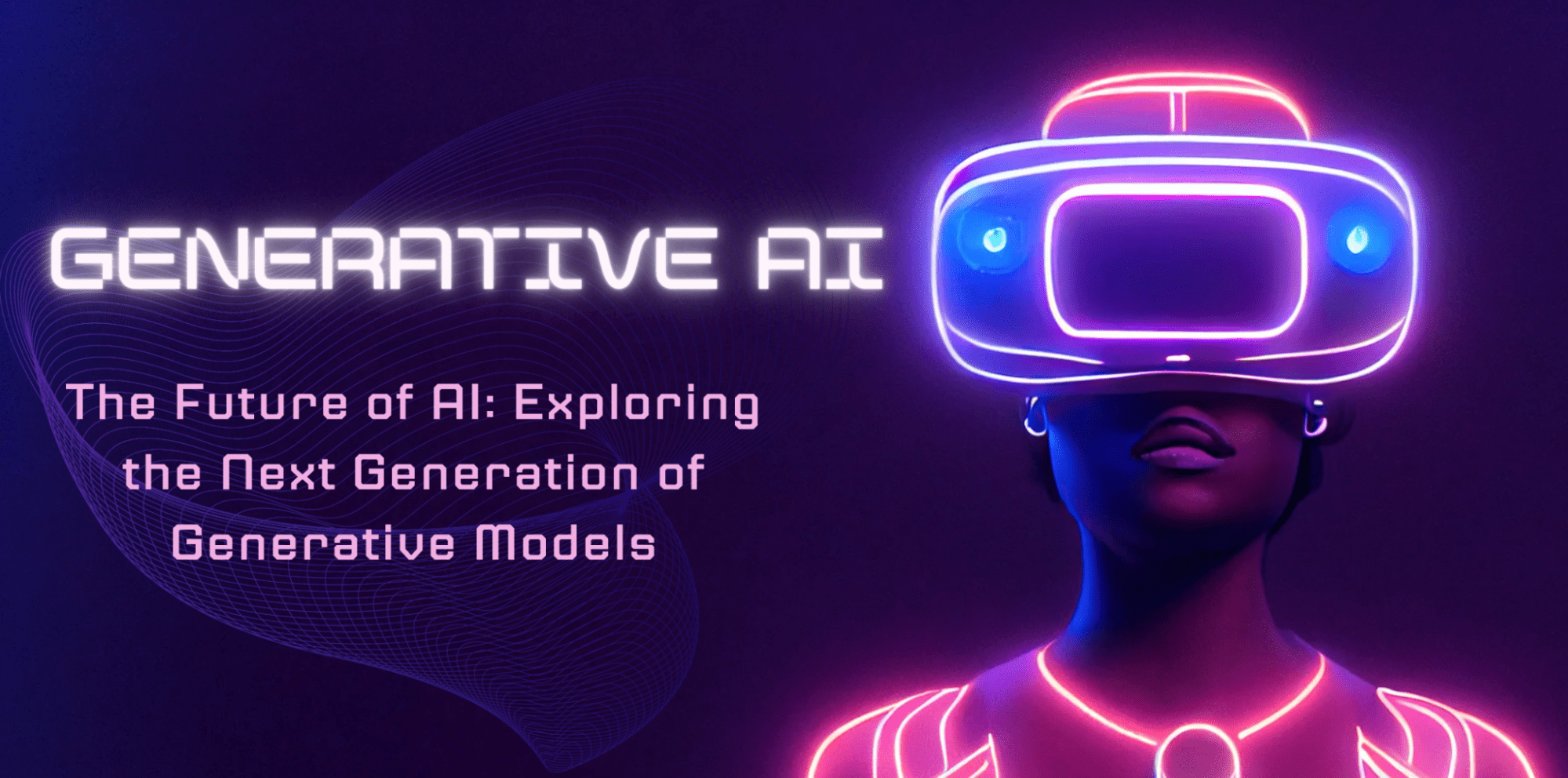
Image by author
If you’re up to date with the world of technology, you know that generative AI is the hottest topic around. We hear a lot about ChatGPT, DALL-E and more.
Recent advances in generative AI will dramatically alter the way we continue to approach content creation and the growth rate of AI tools across industries. Grand View Research stated in its artificial intelligence market size, share and trends analysis report:
“The global artificial intelligence market size was valued at USD 136.55 billion in 2022 and is projected to expand at a compound annual growth rate of 37.3% between 2023 and 2030.”
Every day, more and more organizations, from different sectors or backgrounds, are looking to improve their skills with the use of generative AI.
Generative AI are algorithms that are used to create new and unique content, such as text, audio, code, images, and more. With the development of AI, generative AI has the potential to take over various industries by helping them with tasks that people thought were once impossible.
Generative AI is already creating art that can imitate artists like Van Gogh. The fashion industry can potentially use generative AI to create new designs for its next line. Interior designers can use generative AI to build someone their dream home in days, instead of weeks and months.
Generative AI is fairly new, a work in progress, and still needs time to perfect. However, apps like ChatGPT have set the bar very high and we should expect to see more innovative apps released in the coming years.
The role of generative AI
There are no specific limitations on what generative AI can currently do, as mentioned above it is still a work in progress. However, today, we can categorize it into 3 parts:
- Produce new content/information:
- Replace repetitive tasks:
- Custom data:
This can range from creating a new blog, a video tutorial, or some fancy new art for your wall. However, it can also help in the development of a new drug.
Generative AI can take over tedious and repetitive employee tasks such as emails, presentation summaries, coding, and other types of operations.
Generative AI can create content for specific customer experiences, and this can be used as data to ensure success, ROI, marketing techniques, and customer engagement. Using consumer behavior patterns, companies will be able to distinguish effective strategies and methods.
Below is an example of one of the most popular types of generative AI models, diffusion models.
diffusion model
The diffusion model is designed to learn the underlying structure of a data set by assigning it to a lower dimensional latent space. Latent diffusion models are a type of deep generative neural network, developed by the CompVis group at LMU Munich and Runway.
The diffusion process is when you slowly add or diffuse noise to the compressed latent representation and produce an image that is just noise. However, the diffusion model goes in the opposite direction and performs the reverse process of diffusion. Noise is gradually reduced from the image in a controlled way, so that the image slowly appears to resemble the original.
Image by author
Generative AI has been widely adopted by many organizations from different sectors. It has enabled them to adopt the tools to help fine tune their current processes and methods and elevate them more effectively. For example:
Media
If you are creating a new article, a new image to put on the website or a cool video. Generative AI has taken the media sector by storm, enabling them to produce efficient content at a faster rate while lowering its cost. Personalized content has enabled organizations to take their customer engagement to the next level, providing a more effective customer retention strategy.
Finance
AI tools like Intelligent Document Processing (IDP) for KYC and AML processes. However, generative AI has allowed financial institutions to take their customer analytics further by uncovering new patterns in consumer spending and pinpointing potential issues.
Health care
Generative AI can assist with imaging such as X-rays and CT scans to provide more accurate visualizations, better define images, and detect diagnoses at a faster rate. For example, the use of tools such as illustration-to-photo conversion via Generative Adversarial Networks (GANs) has enabled healthcare professionals to gain a deeper understanding of a patient’s current medical status.
With something big, it goes wrong, right? The rise of generative AI has led to the emergence of how governments will be able to control the use of generative AI tools.
For some time now, the field of AI has been open for organizations to do whatever they want. However, it was a matter of time before someone came in and created fixed regulations around AI. Many are concerned about the oversight of generative AI models and how it will affect the socio-economics, as well as other issues like intellectual property and privacy infringement.
The main challenges currently facing generative AI in terms of governance are:
- Data privacy: Generative AI models require a large amount of data in order to successfully export accurate results. Data privacy is a challenge faced by all AI companies and tools due to the potential misuse of sensitive information.
- Ownership: Intellectual property rights to any content or information that has been created by generative AI is still an open debate. Some may say that the content is unique, while others may say that the text-generated content has been paraphrased from a variety of internet sources.
- Quality – With the large volume of data being fed into generative AI models, the number one concern would be investigating the quality of the data and then the accuracy of the output that has been generated. Fields like medicine are areas of great concern, as dealing with misinformation can have a huge impact.
- Bias: As we analyze the quality of the data, we must also assess the possible bias present in the training data. This can lead to discriminatory results, making the AI unpalatable in many people’s eyes.
Generative AI still has a lot of work to do before it is positively accepted by everyone. These AI models need a better understanding of human speech from different cultural backgrounds. For us, common sense when talking to someone comes naturally to us, however it is not very common for AI systems. They struggle to adapt to different circumstances as they are programmed to be trained on factual information.
It will be interesting to see what role generative AI will play in the future. We have to wait and see.
nisha aria He is a data scientist, freelance technical writer, and community manager at KDnuggets. She is particularly interested in providing Data Science career tips or tutorials and theory-based knowledge about Data Science. She also wants to explore the different ways that Artificial Intelligence is or can benefit the longevity of human life. An enthusiastic student looking to expand her technological knowledge and her writing skills as she helps mentor others.