Large language models (LLMs) have been shown to be able to adapt to target tasks during inference through a process known as few-shot proofs, sometimes known as in-context learning. This ability has become increasingly obvious as model sizes increase, and LLMs show emerging features. An emerging talent is the ability to generalize unknown tasks by following instructions. Instruction wrapping, or RLHF, is one of the suggested instruction learning approaches to improve this capability. Previous research, however, has focused primarily on fine-tuning instruction-learning techniques. The model is multitasking tight on numerous tasks with instructions, which requires many backpropagation procedures.
A group of researchers from KAIST and LG Research shows that instruction-in-context learning (ICIL), which involves learning to follow instructions during inference through learning in context, is advantageous both for readily available pretrained models and for the models that have been specifically developed. tuned to follow instructions, as shown in Figure 1. The indicator used by ICIL comprises many cross-task examples, each of which is an instance of education, input, and output of a task. Because they completely exclude the functions used for the proofs from the evaluation set and because they employ the same set of protests for all the evaluation tasks, treating them as a single fixed indicator, as illustrated in Figure 2, ICIL is an approach zero shot learning.
They create a set of fixed examples using a simple heuristics-based sampling method that works well for various downstream tasks and model sizes. They can test and duplicate initial zero-trigger performance for new tasks or target models without relying on external tools by prepending the same fixed demo set for all jobs. Figure 1 shows that ICIL significantly improves the zero-throw challenge generalization performance of several pretrained LLMs that are not tuned to obey instructions.
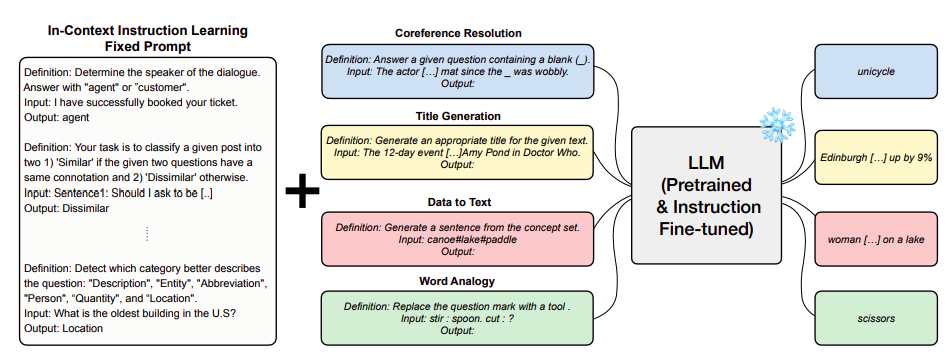
Their data demonstrates that selecting classification tasks that present clear response options in the instruction is what makes ICIL successful. It is important to note that even smaller LLMs with ICIL perform better than larger language models without ICIL. For example, the size 6B ICIL GPT-J outperforms the standard Zero-shot GPT-3 Davinci size 175B by 30. Second, they demonstrate how adding ICIL to instruction-adjusted LLMs improves their ability to follow instruction instructions. zero shots. , particularly for models with more than 100B elements. This suggests that the impact of ICIL is additive to the impact of the instruction modification.
This is true even for target generation tasks, contrary to previous research that suggested that learning in the context of few shots requires retrieving examples comparable to the target task. Even more surprising, they find that performance is not noticeably affected when random phrases are substituted for the distribution of input instances of each example. Based on this approach, they propose that LLMs, instead of relying on the complicated connection between instruction, input, and output, learn the correspondence between the response choice provided in the instruction and the production of each proof during inference. The purpose of ICIL, according to this theory, is to help LLMs focus on target instruction to discover cues for the response distribution of the target task.
review the Paper and Github. All credit for this research goes to the researchers of this project. Also, don’t forget to join our 15k+ ML SubReddit, discord channeland electronic newsletterwhere we share the latest AI research news, exciting AI projects, and more.
Aneesh Tickoo is a consulting intern at MarktechPost. She is currently pursuing her bachelor’s degree in Information Science and Artificial Intelligence at the Indian Institute of Technology (IIT), Bhilai. She spends most of her time working on projects aimed at harnessing the power of machine learning. Her research interest is image processing and she is passionate about creating solutions around her. She loves connecting with people and collaborating on interesting projects.