Reconstructing unmeasured causal factors of complex time series from observed response data represents a fundamental challenge in various scientific domains. Latent variables, including genetic regulators or environmental factors, are essential in determining the dynamics of a system, but they are rarely measured. Challenges with current approaches arise from data noise, high dimensionality of systems, and the capabilities of existing algorithms to handle nonlinear interactions. This will be of great help when modeling, predicting and controlling high-dimensional systems in systems biology, ecology and fluid dynamics.
The most widely used techniques for reconstructing causal factors are usually based on signal processing or machine learning frameworks. Some of the most common include mutual information methods, neural network applications, and dynamic attractor reconstruction. While these techniques work well in some situations, they have important limitations. Most require large, high-quality data sets that are rarely found in real-world applications. They are very prone to measurement noise, resulting in low reconstruction accuracy. Some require computationally expensive algorithms and are therefore not suitable for real-time applications. Furthermore, many models lack physical principles, which reduces their interpretability and applicability across domains.
Researchers at the University of Texas introduce a physics-based unsupervised learning framework called SHREC (Shared Recurrences) to reconstruct causal factors from time series data. The approach is based on the theory of skew-product dynamical systems and topological data analysis. The innovation includes the use of recurring events in time series to infer common causal structures between responses, the construction of a consensus recurrence graph that is traversed to expose latent driver dynamics, and the introduction of a new integrated network that adapts to noisy environments and sparse data sets using fuzzy simplicial complexes. Unlike existing methods, the SHREC framework captures noisy and nonlinear data well, requires minimal parameter tuning, and provides useful information about the physical dynamics underlying driver response systems.
The SHREC algorithm is implemented in multiple stages. The measured response time series are mapped to weighted recurrence networks using topological embeddings, where an affinity matrix is constructed for each time series based on nearest neighbor distances and adaptive thresholds. Recurrence charts are combined from individual time series to obtain a consensus chart that captures the collective dynamics. Discrete-time controllers have been linked to decomposition by community detection algorithms, including the Leiden method, to provide distinct equivalence classes. For continuous conductors, on the other hand, the Laplacian decomposition of the graph reveals transient modes corresponding to states of the conductors. The algorithm was tested with various data: gene expression, plankton abundance and turbulent flows. It showed excellent reconstruction of drivers under challenging conditions such as high noise and missing data. The framework structure is based on graph-based representations. Therefore, it avoids costly gradient-based iterative optimization and makes it computationally efficient.
SHREC performed remarkably well and consistently on data sets that challenged benchmarks. The methodology successfully reconstructed causal determinants from gene expression datasets, thereby uncovering essential regulatory components, even in the presence of sparse and noisy data. In experiments with turbulent flow, this approach successfully detected sinusoidal forcing factors, demonstrating superiority over traditional signal processing techniques. Regarding ecological data sets, SHREC revealed temperature-induced trends in plankton populations, despite considerable missing data, illustrating its resilience to incomplete and noisy data. Comparison with other approaches has highlighted the higher computational accuracy and efficiency of SHREC, especially in the presence of higher noise levels and complex nonlinear dependencies. These findings highlight its wide applicability and reliability in many fields.
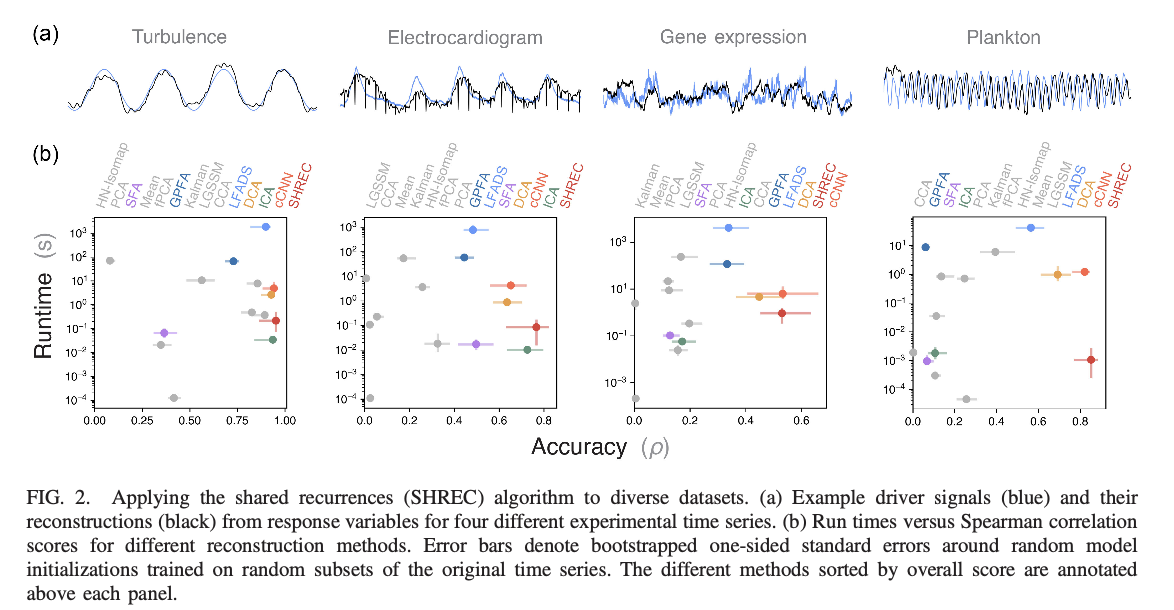
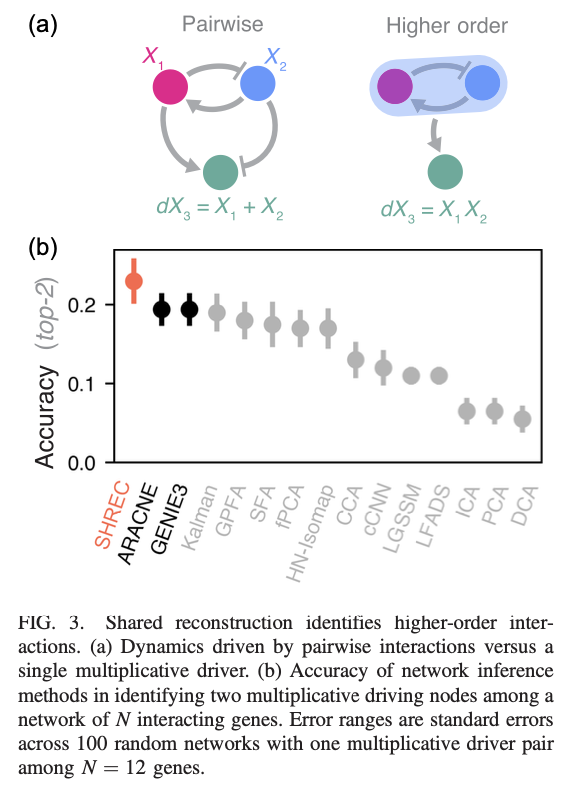
SHREC is a physics-based unsupervised learning framework that enables the reconstruction of unobserved causal factors from complex time series data. This new approach addresses serious drawbacks of contemporary techniques, including susceptibility to noise and high computational cost, by using recurrence structures and topological embeddings. The successful feasibility of SHREC on diverse data sets underlines its broad applicability with the ability to improve ai-based modeling in biology, physics, and engineering disciplines. This methodology improves the accuracy of the reconstruction of causal factors and, at the same time, establishes a framework based on the principles of dynamical systems theory and sheds new light on the essential characteristics of information transfer within interconnected systems.
Verify he Paper. All credit for this research goes to the researchers of this project. Also, don't forget to follow us on <a target="_blank" href="https://x.com/intent/follow?screen_name=marktechpost” target=”_blank” rel=”noreferrer noopener”>twitter and join our Telegram channel and LinkedIn Grabove. Don't forget to join our SubReddit over 65,000 ml.
<a target="_blank" href="https://nebius.com/blog/posts/studio-embeddings-vision-and-language-models?utm_medium=newsletter&utm_source=marktechpost&utm_campaign=embedding-post-ai-studio” target=”_blank” rel=”noreferrer noopener”> (Recommended Reading) Nebius ai Studio Expands with Vision Models, New Language Models, Embeddings, and LoRA (Promoted)
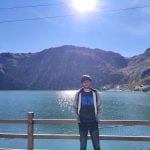
Aswin AK is a Consulting Intern at MarkTechPost. He is pursuing his dual degree from the Indian Institute of technology Kharagpur. He is passionate about data science and machine learning, and brings a strong academic background and practical experience solving real-life interdisciplinary challenges.