Video-based technologies have become essential tools for information retrieval and understanding complex concepts. Videos combine visual, temporal and contextual data, providing a multimodal representation that surpasses static images and text. With the growing popularity of video-sharing platforms and the vast repository of educational and informational videos available online, leveraging videos as sources of knowledge offers unprecedented opportunities to answer queries that require detailed context, spatial understanding, and process demonstration.
Augmented generation retrieval systems, which combine retrieval and response generation, often neglect the full potential of video data. These systems typically rely on textual information or occasionally include static images to support responses to queries. However, they fail to capture the richness of videos, which include visual dynamics and multimodal cues essential for complex tasks. Conventional methods predefine videos relevant to the query without retrieving them or convert videos to textual formats, losing critical information such as visual context and temporal dynamics. This insufficiency makes it difficult to provide accurate and informative answers to real-world multimodal queries.
Current methodologies have explored text- or image-based retrieval, but have not fully utilized video data. In traditional RAG systems, video content is represented as subtitles, focusing solely on textual aspects or being reduced to preselected frames for specific analysis. Both approaches limit the multimodal richness of the videos. Furthermore, the absence of techniques to dynamically retrieve and embed query-relevant videos further restricts the effectiveness of these systems. The lack of comprehensive video integration leaves an untapped opportunity to improve the augmented recall generation paradigm.
The KaiST and DeepAuto.ai research teams proposed a novel framework called VideoRAG to address the challenges associated with using video data in recovery augmented generation systems. VideoRAG dynamically retrieves query-relevant videos from a large corpus and incorporates visual and textual information into the generation process. Leverage the capabilities of advanced large video language models (LVLMs) for seamless integration of multimodal data. The approach represents a significant improvement over previous methods by ensuring that the retrieved videos are contextually aligned with user queries and maintaining the temporal richness of the video content.
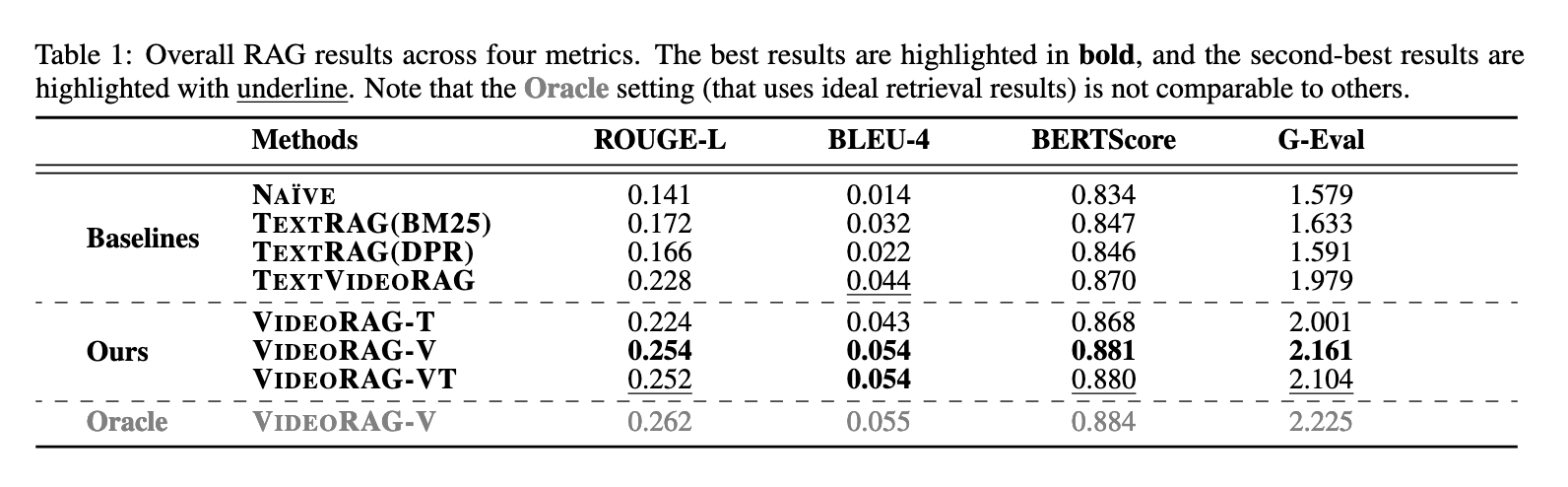
The proposed methodology involves two main stages: recovery and generation. It then identifies the videos by their similar visual and textual aspects related to the query during retrieval. VideoRAG applies automatic speech recognition to generate auxiliary textual data for a video that is not available with subtitles. This stage ensures that response generation from all videos gets meaningful contributions from each video. The relevant retrieved videos are fed into the frame generation module, where multimodal data such as frames, subtitles, and query text are integrated. These inputs are processed comprehensively in the LVLMs, allowing them to produce long, rich, precise, and contextually appropriate responses. VideoRAG's focus on combinations of visual and textual elements makes it possible to represent complexities in complex processes and interactions that cannot be described using static modalities.
VideoRAG has been extensively experimented on datasets such as WikiHowQA and HowTo100M. These data sets cover a wide spectrum of queries and video content. In particular, the approach revealed better response quality, according to several metrics, such as ROUGE-L, BLEU-4, and BERTScore. Thus, regarding the VideoRAG method, the score was 0.254 according to ROUGE-L, while for the text-based methods, RAG reported 0.228 as the maximum score. The same was also demonstrated with BLEU-4, n-gram overlay: for VideoRAG; this is 0.054; for the text-based one, it was only 0.044. The framework variant, which used video frames and transcripts, further improved performance and achieved a BERTScore score of 0.881, compared to 0.870 for the baseline methods. These results highlight the importance of multimodal integration to improve response accuracy and underscore the transformative potential of VideoRAG.
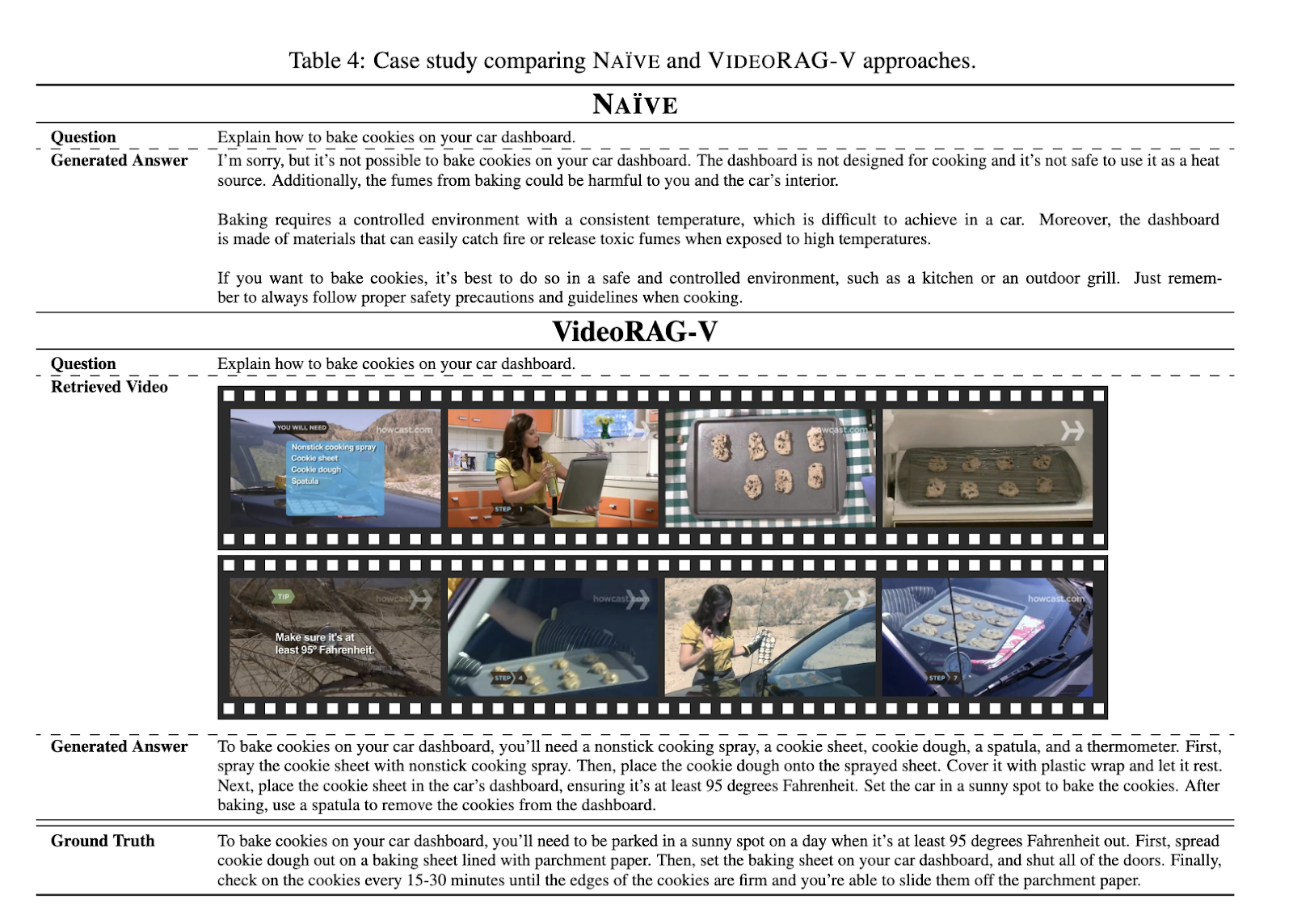
The authors demonstrated that VideoRAG's ability to dynamically combine visual and textual elements leads to more contextually rich and accurate responses. Compared to traditional RAG systems that rely solely on static image or textual data, VideoRAG excels in scenarios that require detailed spatial and temporal understanding. Including auxiliary text generation for videos without subtitles further ensures consistent performance across diverse data sets. By enabling video corpus-based retrieval and generation, the framework addresses the limitations of existing methods and establishes a benchmark for future multimodal augmented retrieval systems.
Simply put, VideoRAG represents a big step forward in augmented recall generation systems because it leverages video content to improve response quality. This model combines state-of-the-art retrieval techniques with the power of LVLMs to deliver accurate, context-rich responses. Methodologically, it successfully addresses the shortcomings of current systems, thereby providing a robust framework for incorporating video data into knowledge generation channels. With its superior performance across multiple metrics and datasets, VideoRAG establishes itself as a novel approach for including videos in augmented recall generation systems.
Verify he Paper. All credit for this research goes to the researchers of this project. Also, don't forget to follow us on <a target="_blank" href="https://x.com/intent/follow?screen_name=marktechpost” target=”_blank” rel=”noreferrer noopener”>twitter and join our Telegram channel and LinkedIn Grabove. Don't forget to join our SubReddit over 65,000 ml.
Recommended open source ai platform: 'Parlant is a framework that transforms the way ai agents make decisions in customer-facing scenarios.' (Promoted)
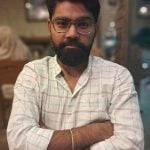
Nikhil is an internal consultant at Marktechpost. He is pursuing an integrated double degree in Materials at the Indian Institute of technology Kharagpur. Nikhil is an ai/ML enthusiast who is always researching applications in fields like biomaterials and biomedical science. With a strong background in materials science, he is exploring new advances and creating opportunities to contribute.