Proteins, essential molecular machines evolved over billions of years, perform critical life-sustaining functions encoded in their sequences and revealed through their three-dimensional structures. Decoding their functional mechanisms remains a central challenge in biology despite advances in experimental and computational tools. While AlphaFold and similar models have revolutionized structure prediction, the gap between structural knowledge and functional understanding persists, compounded by the exponential growth of unannotated protein sequences. Traditional tools rely on evolutionary similarities, which limits their reach. Emerging protein language models are promising, leveraging deep learning to decode protein “language,” but limited, diverse, and context-rich training data limit their effectiveness.
Researchers at Westlake University and Nankai University developed Evola, an 80 billion-parameter multimodal protein language model designed to interpret the molecular mechanisms of proteins through natural language dialogue. Evola integrates a protein language model (PLM) as an encoder, an LLM as a decoder, and an alignment module, enabling accurate predictions of protein function. Empowered with an unprecedented dataset of 546 million protein pairs, Q&As, and 150 billion tokens, Evola leverages recall augmented generation (RAG) and direct preference optimization (DPO) to improve relevance and the quality of the response. Evola, evaluated using the novel Instructional Response Space (IRS) framework, provides expert-level insights, advancing proteomics research.
Evola is a multimodal generative model designed to answer questions about functional proteins. Integrate protein-specific knowledge with LLM for accurate, context-aware answers. Evola features a frozen protein encoder, a trainable sequence compressor and aligner, and a pre-trained LLM decoder. It employs DPO to make adjustments based on preferences scored by GPT and RAG to improve response accuracy using Swiss-Prot and ProTrek data sets. Applications include protein function annotation, enzyme classification, gene ontology, subcellular localization, and disease association. Evola is available in two versions: a 10B parameter model and a 80B parameter model still in formation.
The study presents Evola, an advanced 80 billion-parameter multimodal protein language model designed to interpret protein functions through natural language dialogue. Evola integrates a protein language model as an encoder, a large language model as a decoder, and an intermediate module for compression and alignment. It employs RAG to incorporate external knowledge and DPO to improve response quality and refine results based on preference signals. The evaluation using the IRS framework demonstrates Evola's ability to generate accurate and contextually relevant insights into protein functions, thereby advancing proteomics and functional genomics research.
The results show that Evola outperforms existing models in predicting protein function and in natural language dialogue tasks. Evola was evaluated on diverse data sets and achieved state-of-the-art performance in generating accurate and context-sensitive answers to protein-related questions. Benchmarking against the IRS framework revealed its high accuracy, interpretability, and response relevance. Qualitative analysis highlighted Evola's ability to address nuanced functional queries and generate protein annotations comparable to expert-curated knowledge. Furthermore, ablation studies confirmed the effectiveness of their training strategies, including augmented generation by retrieval and direct preference optimization, in improving response quality and alignment with biological contexts. This establishes Evola as a strong tool for proteomics.
In conclusion, Evola is an 80 billion parameter generative protein language model designed to decode the molecular language of proteins. Using natural language dialogue, it links protein sequences, structures and biological functions. Evola's innovation lies in its training on an ai-synthesized data set of 546 million protein question-answer pairs, spanning 150 billion tokens, an unprecedented scale. By employing DPO and RAG, you refine the quality of the response and integrate external knowledge. Assessed by the IRS, Evola offers expert-level insights, advancing proteomics and functional genomics, while providing a powerful tool to unravel the molecular complexity of proteins and their biological functions.
Verify he Paper. All credit for this research goes to the researchers of this project. Also, don't forget to follow us on <a target="_blank" href="https://x.com/intent/follow?screen_name=marktechpost” target=”_blank” rel=”noreferrer noopener”>twitter and join our Telegram channel and LinkedIn Grabove. Don't forget to join our SubReddit over 60,000 ml.
UPCOMING FREE ai WEBINAR (JANUARY 15, 2025): <a target="_blank" href="https://info.gretel.ai/boost-llm-accuracy-with-sd-and-evaluation-intelligence?utm_source=marktechpost&utm_medium=newsletter&utm_campaign=202501_gretel_galileo_webinar”>Increase LLM Accuracy with Synthetic Data and Assessment Intelligence–<a target="_blank" href="https://info.gretel.ai/boost-llm-accuracy-with-sd-and-evaluation-intelligence?utm_source=marktechpost&utm_medium=newsletter&utm_campaign=202501_gretel_galileo_webinar”>Join this webinar to learn practical information to improve LLM model performance and accuracy while protecting data privacy..
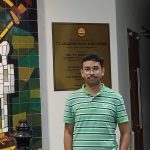
Sana Hassan, a consulting intern at Marktechpost and a dual degree student at IIT Madras, is passionate about applying technology and artificial intelligence to address real-world challenges. With a strong interest in solving practical problems, he brings a new perspective to the intersection of ai and real-life solutions.