Rapid advances in large language models (LLM) have introduced significant opportunities for various industries. However, its implementation in real-world scenarios also presents challenges, such as the generation of harmful content, hallucinations, and potential ethical misuse. LLMs can produce socially biased, violent, or profane results, and adversarial actors often exploit vulnerabilities through jailbreaking to bypass security measures. Another critical problem lies in retrieval augmented generation (RAG) systems, where LLMs integrate external data but may provide contextually irrelevant or factually incorrect answers. Addressing these challenges requires strong safeguards to ensure responsible and safe use of ai.
To address these risks, IBM has introduced Granite Guardian, a set of open source security measures for risk detection in LLM. This suite is designed to detect and mitigate multiple dimensions of risk. The Granite Guardian suite identifies harmful prompts and responses, covering a broad spectrum of risks, including social bias, profanity, violence, unethical behavior, sexual content, and hallucination-related issues specific to RAG systems. Launched as part of IBM's open source initiative, Granite Guardian aims to promote transparency, collaboration and responsible development of ai. With a comprehensive risk taxonomy and training datasets enriched with human annotations and synthetic adversarial samples, this suite provides a versatile approach for risk detection and mitigation.
Technical details
Granite Guardian models, based on IBM's Granite 3.0 framework, are available in two variants: a lightweight 2 billion parameter model and a more comprehensive 8 billion parameter version. These models integrate diverse data sources, including human-annotated datasets and adversarial-generated synthetic samples, to improve their generalization across various risks. The system effectively addresses jailbreak detection, which is often overlooked by traditional security frameworks, using synthetic data designed to mimic sophisticated adversary attacks. Additionally, the models incorporate capabilities to address specific RAG risks, such as context relevance, substantiation, and response relevance, ensuring that generated results align with user intentions and factual accuracy.
A notable feature of Granite Guardian is its adaptability. The models can be integrated into existing ai workflows as guardrails or real-time evaluators. Its high-performance metrics, including AUC scores of 0.871 and 0.854 for harmful content and RAG hallucination benchmarks, respectively, demonstrate its applicability in various scenarios. Additionally, the open source nature of Granite Guardian encourages community-driven improvements, encouraging improvements in ai security practices.
Outlook and results
Extensive benchmarking highlights the effectiveness of Granite Guardian. On public datasets for harmful content detection, variant 8B achieved an AUC of 0.871, outperforming baselines such as Llama Guard and ShieldGemma. Their precision-recall trade-offs, represented by an AUPRC of 0.846, reflect their ability to detect harmful cues and responses. In RAG-related evaluations, the models demonstrated strong performance, with model 8B achieving an AUC of 0.895 in identifying grounding issues.
The models' ability to generalize across diverse data sets, including conflicting indications and queries from real-world users, shows their robustness. For example, in the ToxicChat dataset, Granite Guardian demonstrated high recall, effectively flagging harmful interactions with minimal false positives. These results indicate the suite's ability to provide reliable and scalable risk detection solutions in practical ai implementations.
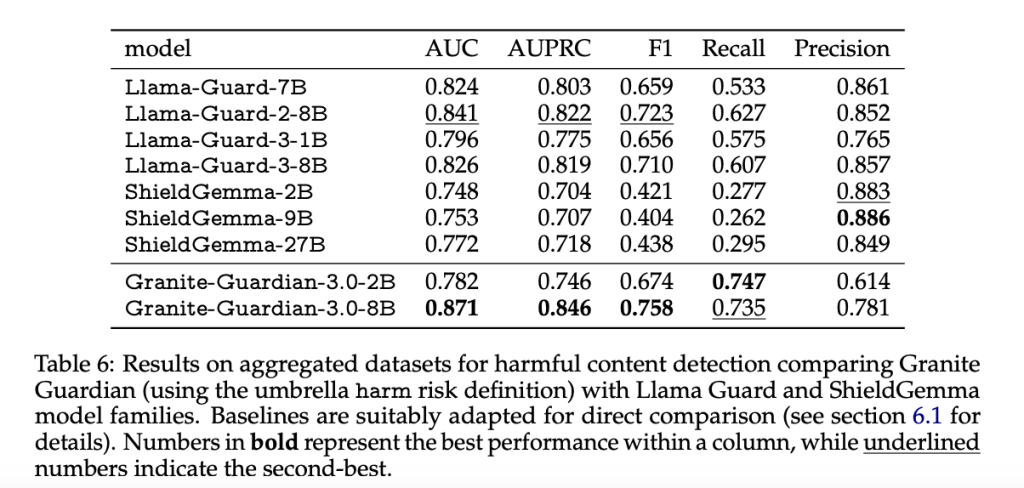
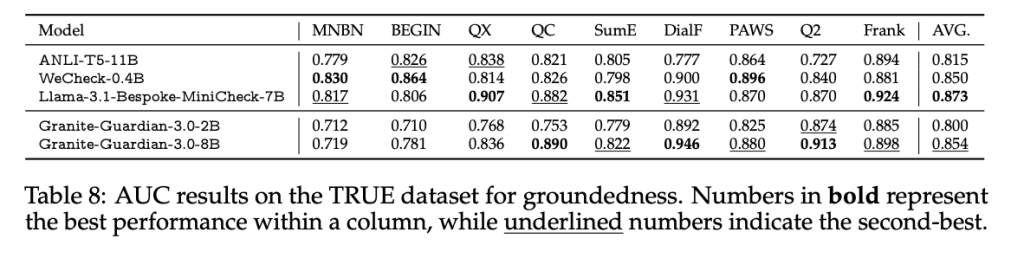
Conclusion
IBM's Granite Guardian offers a comprehensive solution to protect LLMs from risk, emphasizing security, transparency and adaptability. Its ability to detect a wide range of risks, combined with open source accessibility, makes it a valuable tool for organizations looking to implement ai responsibly. As LLMs continue to evolve, tools like Granite Guardian ensure that this progress is accompanied by effective safeguards. By supporting collaboration and community-driven improvements, IBM helps improve ai security and governance, promoting a safer ai landscape.
Verify he Paper, Granite Guardian 3.0 2B, Granite Guardian 3.0 8B and GitHub page. All credit for this research goes to the researchers of this project. Also, don't forget to follow us on <a target="_blank" href="https://twitter.com/Marktechpost”>twitter and join our Telegram channel and LinkedIn Grabove. Don't forget to join our SubReddit over 60,000 ml.
Trending: LG ai Research launches EXAONE 3.5 – three frontier-level bilingual open-source ai models that deliver unmatched instruction following and broad context understanding for global leadership in generative ai excellence….
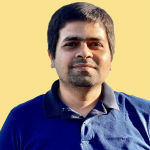
Asif Razzaq is the CEO of Marktechpost Media Inc.. As a visionary entrepreneur and engineer, Asif is committed to harnessing the potential of artificial intelligence for social good. Their most recent endeavor is the launch of an ai media platform, Marktechpost, which stands out for its in-depth coverage of machine learning and deep learning news that is technically sound and easily understandable to a wide audience. The platform has more than 2 million monthly visits, which illustrates its popularity among the public.
<script async src="//platform.twitter.com/widgets.js” charset=”utf-8″>