Since the Industrial Revolution, the burning of fossil fuels and changes in land use, especially deforestation, have driven increases in atmospheric carbon dioxide (CO2). While terrestrial vegetation and oceans serve as natural carbon sinks, absorbing some of this CO2, emissions have consistently exceeded their annual capacity. This imbalance has continually increased atmospheric CO2 concentrations, fueling global warming and extreme weather events. Understanding the carbon budget (how CO2 is obtained and absorbed) has become essential to combating climate change, especially as countries strive to achieve carbon neutrality.
The main challenge lies in accurately estimating the carbon budget and its environmental impact. The carbon budget measures the balance between emissions from fossil fuels, cement production, land use changes and natural sources of CO2 against the absorption capacity of carbon sinks. It is easier to address the growing climate crisis with accurate and timely data on CO2 levels and carbon sinks. Existing methods fail to track changes in global carbon sinks quickly enough, especially when environmental perturbations (such as wildfires or El Niño) alter carbon dynamics in unpredictable ways.
Traditional carbon budgeting methods typically rely on numerical simulations of the Earth's carbon cycle. While these models can simulate complex Earth system processes, they often face significant delays. For example, the Global Carbon Budget 2023 report, which uses data through the end of 2022, illustrates the one-year lag in carbon budget information. This delay limits the effectiveness of current models in providing timely climate data that can guide real-world action. Researchers need a faster, more reliable way to capture the sudden changes in carbon dynamics that affect global warming.
To address these limitations, researchers at Microsoft Research Asia, in collaboration with Tsinghua University, the French Laboratory for Climate and Environmental Sciences, and other global research organizations, introduced a ai-powered method to budget carbon emissions in near real time. By integrating satellite data, dynamic global vegetation models, and ocean model emulators, the research team developed a near-instantaneous carbon sink model capable of predicting carbon budgets with unprecedented speed and accuracy. This model leverages the power of convolutional neural networks (CNN) and semi-supervised learning techniques to deliver low-latency results.
The proposed ai-based model uses observations of environmental variables and historical data to predict global carbon sink levels. The model integrates 12 months of historical data, monthly characteristics and target results. CNNs process this data to calculate predictions, while semi-supervised learning provides an unsupervised loss function to improve the accuracy of predictions. The model processes environmental data from ocean and land sinks and satellite fire emissions to provide real-time updates on CO2 sinks. This methodology ensures that predictions are made with a margin of error of less than 2%, offering a fast and responsive alternative to traditional carbon budgeting methods.
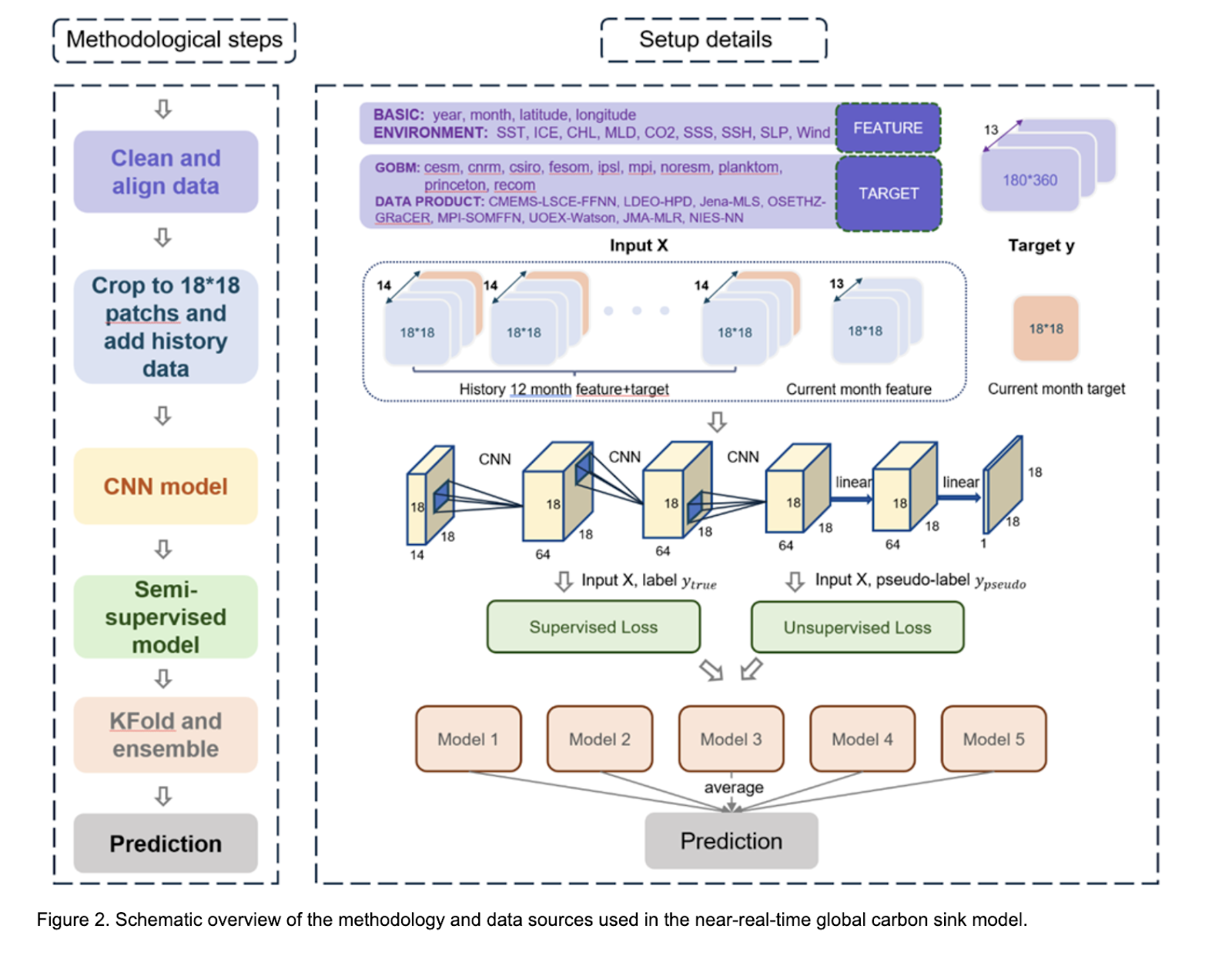
The results of this near real-time carbon sink model showed promising accuracy. In particular, the model was able to track a dramatic decline in the terrestrial carbon sink in 2023. The amazon rainforest, severely affected by drought, showed a carbon sink loss of 0.31 ± 0.19 GtC. The model also accurately predicted carbon emissions from the 2023 North American wildfires, contributing 0.58 ± 0.10 GtC to atmospheric CO2. Additionally, the model detected a shift from the La Niña phase to a moderate El Niño phase, which significantly impacted global carbon dynamics. These findings highlight the effectiveness of the ai model in capturing dynamic environmental changes and producing actionable data in near real-time.
In conclusion, the rapid decline of terrestrial carbon sinks poses a serious threat to the effectiveness of global carbon neutrality efforts. The ai-based carbon budget model presented by the research team at Microsoft Research Asia, Tsinghua University and the French Laboratory for Climate and Environmental Sciences provides an innovative solution to the challenges of carbon budget estimation . This model's ability to produce real-time predictions and track environmental changes more accurately than traditional methods is a crucial step forward in global efforts to combat climate change. By reducing the delay in carbon data updates, this approach enables more effective climate action and policymaking in response to urgent environmental threats.
Verify he Details here. All credit for this research goes to the researchers of this project. Also, don't forget to follow us on <a target="_blank" href="https://twitter.com/Marktechpost”>twitter and join our Telegram channel and LinkedIn Grabove. Don't forget to join our SubReddit over 60,000 ml.
(You must subscribe): Subscribe to our newsletter to receive updates on ai research and development
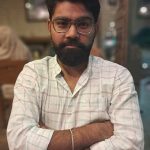
Nikhil is an internal consultant at Marktechpost. He is pursuing an integrated double degree in Materials at the Indian Institute of technology Kharagpur. Nikhil is an ai/ML enthusiast who is always researching applications in fields like biomaterials and biomedical science. With a strong background in materials science, he is exploring new advances and creating opportunities to contribute.
<script async src="//platform.twitter.com/widgets.js” charset=”utf-8″>