The study of collective decision making in biological and artificial systems addresses critical challenges to understanding how groups achieve consensus through simple interactions. These processes underpin behaviors in animal herds, human groups, and robot swarms. Recent advances in neuroscience have explored how neuronal dynamics, oscillations, and phase-locking mechanisms facilitate these decisions in biological systems. However, it is still necessary to explore the application of these dynamics in multi-agent systems. Closing this gap can improve group decision-making models, enabling more adaptive and socially intelligent agents for navigation, search and rescue tasks.
A fundamental issue in this field is the balance between internal dynamics, environmental feedback and social influences. Agents must adapt their behavior in response to external stimuli while coordinating with their peers to reach a shared decision. For example, agents deciding between two resource locations must integrate their sensory information and social interactions to achieve convergence. Overreliance on internal states or external cues can hinder your ability to make effective decisions. This interaction is particularly relevant in dynamic environments with conflicting stimuli.
Traditional models, such as opinion dynamics or rules based on heuristics, have provided ideas for consensus building. These approaches are typically based on simple majority rules or predefined alignment algorithms. While useful, these models often ignore the complex neural and sensorimotor mechanisms underlying decision-making in biological systems. For example, models such as Kuramoto oscillators describe synchronization but lack a direct link to embodied behavior. Few existing approaches address the neural dynamics that drive coordination between agents in real-world scenarios.
Researchers from Université Libre de Bruxelles, Université de Montréal, Universiteit Gent and Mila—Quebec ai Institute introduced a multi-agent model that incorporates biologically plausible neural dynamics designed to mimic sensorimotor feedback and brain oscillations observed in nature . The system used oscillatory models governed by Haken-Kelso-Bunz equations to simulate metastable neural states, allowing agents to dynamically adapt to environmental and social conditions. Agents featured sensory and motor oscillators that interacted within a closed loop, allowing them to navigate stimulus gradients and coordinate movements with their partners.
The architecture of the proposed system included four oscillators: two sensory nodes for stereovision and two motor nodes for differential steering. Sensory information was integrated into the neural controller, allowing agents to detect stimulus gradients and adjust their direction accordingly. Social interactions modeled as stimulus delivery improved coordination between agents, where agents influenced each other based on proximity. Neural dynamics were tuned by adjusting coupling parameters, sensory sensitivity, and social influence, creating a balance between environmental responsiveness and group alignment.
The performance was evaluated in 50 simulations with different parameters. Agents achieved peak performance when internal coupling ranged between 0.8 and 1.5, with sensory sensitivity set to five and social influence set to 1. Agents showed high metastability at these values, allowing for flexible behavior. but coordinated. In binary decision-making scenarios, agents were able to select one of two stimulus sources, and performance improved as the difference in quality between the stimuli increased. When social influences dominated or internal dynamics became too rigid, performance declined, demonstrating the need for a balanced approach.
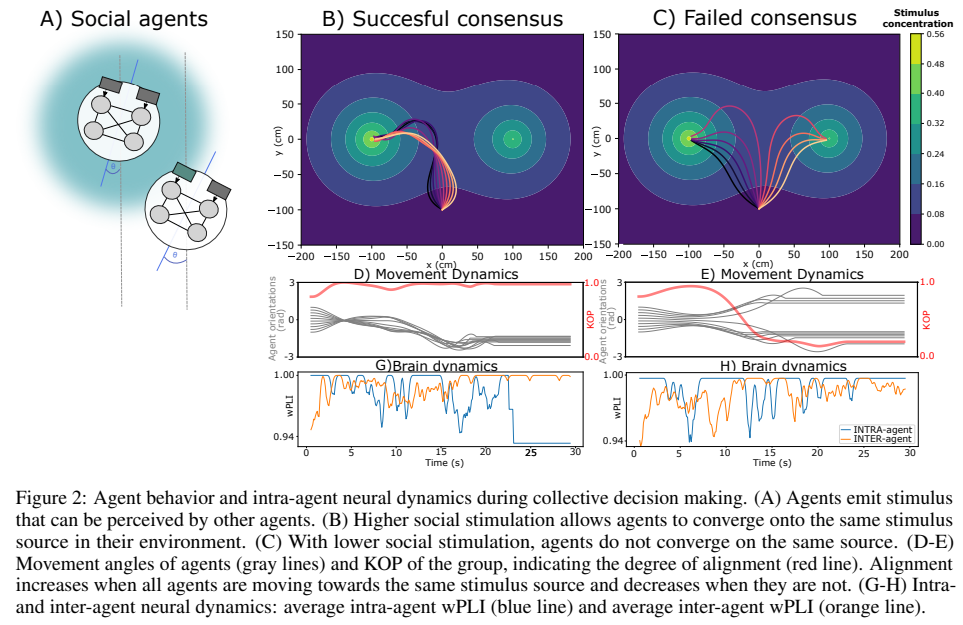
The results revealed several key takeaways from the study:
- Optimal coupling: Agents performed best with moderate internal coupling (0.8 to 1.5), balancing flexibility and alignment.
- Environmental sensitivity: Sensory input significantly influenced neural dynamics, and increased input drove rapid state changes but required restraint to avoid instability.
- Social influence: Effective coordination required social influence values of approximately 1, beyond which agents became overly dependent on their peers and failed to interact adaptively with the environment.
- Consensus challenges: Variations in agents' initial orientations and stimulus source quality indices affected convergence, highlighting the interplay between individual and group dynamics.
- Metastability: Agents operating in a metastable neural regime demonstrated greater adaptability, successfully navigating conflicting stimuli and achieving group alignment.
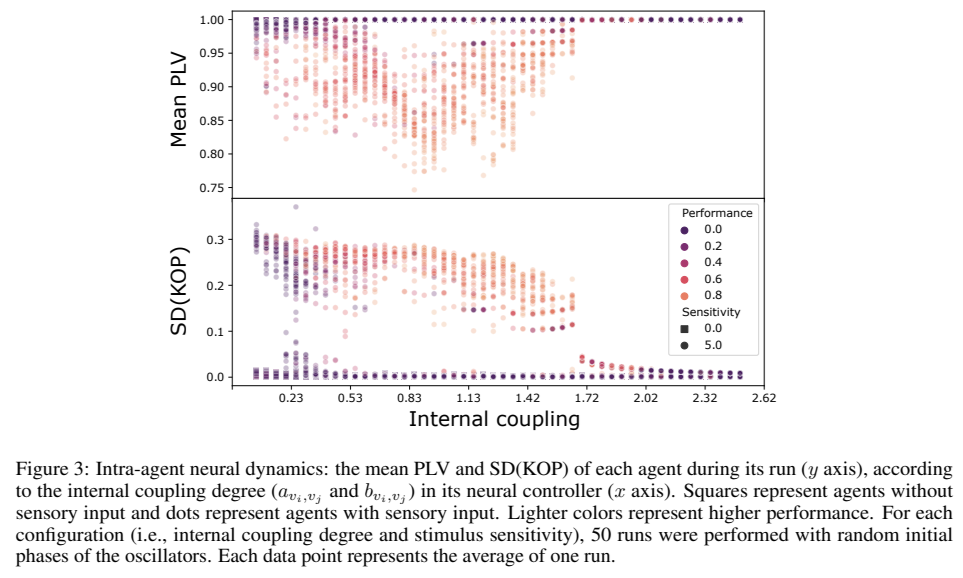
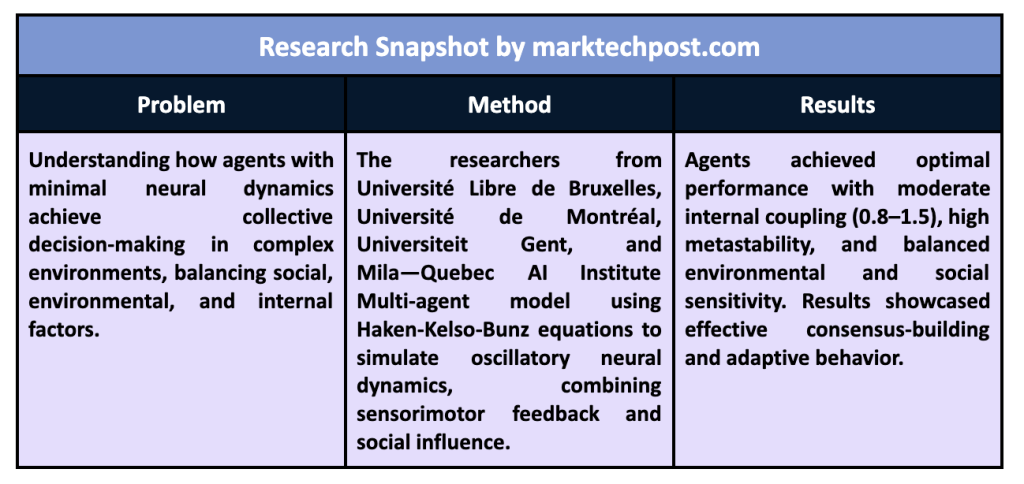
In conclusion, this research bridges neuroscience and artificial intelligence by demonstrating how biologically inspired neural dynamics can improve collective decision making in multi-agent systems. By integrating sensorimotor feedback, social interactions, and metastable neural states, the study provides a robust framework for designing intelligent agents. These findings paved the way for future applications in collaborative robotics, swarm intelligence, and adaptive systems capable of operating in complex environments.
Verify the paper. All credit for this research goes to the researchers of this project. Also, don't forget to follow us on <a target="_blank" href="https://twitter.com/Marktechpost”>twitter and join our Telegram channel and LinkedIn Grabove. If you like our work, you will love our information sheet.. Don't forget to join our SubReddit over 55,000ml.
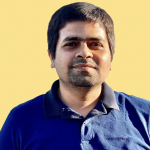
Asif Razzaq is the CEO of Marktechpost Media Inc.. As a visionary entrepreneur and engineer, Asif is committed to harnessing the potential of artificial intelligence for social good. Their most recent endeavor is the launch of an ai media platform, Marktechpost, which stands out for its in-depth coverage of machine learning and deep learning news that is technically sound and easily understandable to a wide audience. The platform has more than 2 million monthly visits, which illustrates its popularity among the public.
<script async src="//platform.twitter.com/widgets.js” charset=”utf-8″>