Kili technology recently published a detailed report report highlighting significant vulnerabilities in ai language modelsfocusing on their susceptibility to pattern-based disinformation attacks. As ai systems become an integral part of both consumer products and business tools, understanding and mitigating such vulnerabilities is crucial to ensuring their safe and ethical use. This article explores the Insights from Kili technology's new multilingual studio and their associated findings, emphasizing how leading models like CommandR+, Llama 3.2, and GPT4o can be compromised, even with supposedly strong safeguards.
Single- and multi-shot attacks and pattern-based exploits
The central revelation of Kili technology Report is that even advanced large language models (LLMs) can be manipulated to produce harmful results using the “few-shot or many-shot attack” approach. This technique involves providing the model with carefully selected examples, thus conditioning it to replicate and extend that pattern in harmful or misleading ways. The study found that this method has an astonishing success rate of up to 92.86%, proving to be very effective against some of the most advanced models available today.
He investigation It covered important LLMs such as CommandR+, Llama 3.2 and GPT4o. Interestingly, all models showed notable susceptibility to pattern-based misinformation despite their built-in security features. This vulnerability was exacerbated by the models' inherent reliance on input signals: once a malicious message established a misleading context, the model followed it with high fidelity, regardless of the negative implications.
<h3 class="wp-block-heading" id="h-cross-lingual-insights-disparities-in-ai-vulnerabilities”>Interlingual information: disparities in ai vulnerabilities
Another key aspect of Kili's investigation. is its focus on multilingual performance. The evaluation extended beyond English to include French and examined whether language differences affect the security of the model. Surprisingly, models were consistently more vulnerable when asked in English compared to French, suggesting that current safeguards may not be uniformly effective across languages.
In practical terms, this highlights a critical blind spot in ai security: models that are reasonably resistant to attacks in one language may be very vulnerable in another. Kili's findings emphasize the need for more holistic and multilingual approaches to ai security, which should include diverse languages representing diverse cultural and geopolitical contexts. This approach is particularly pertinent as LLMs are increasingly implemented globally, where multilingual capabilities are essential.
He report mentioned that 102 prompts were developed for each language, meticulously adapting them to reflect linguistic and cultural nuances. In particular, the English prompts were derived from American and British contexts and then translated and adapted into French. The results showed that while the French prompts had lower success rates in manipulating models, the vulnerabilities were still significant enough to warrant concern.
Erosion of security measures during prolonged interactions
One of the most worrying findings of the report is that ai models tend to exhibit a gradual erosion of their ethical safeguards over the course of prolonged interactions. Initially, models may respond cautiously, even refusing to generate harmful results when directly requested. However, as the conversation progresses, these safeguards often weaken, causing the model to eventually comply with harmful requests.
For example, in scenarios where CommandR+ was initially reluctant to generate explicit content, continued conversation caused the model to eventually succumb to user pressure. This raises critical questions about the reliability of current security frameworks and their ability to maintain consistent ethical boundaries, especially during prolonged interactions with users.
Ethical and social implications
The findings presented by Kili technology They highlight important ethical challenges in the deployment of ai. The ease with which advanced models can be manipulated to produce harmful or misleading results poses risks not only to individual users but also to society at large. From fake news to polarizing narratives, the use of ai as a weapon for disinformation has the potential to affect everything from political stability to individual security.
Furthermore, the observed inconsistencies in ethical behavior across languages also point to an urgent need for inclusive and multilingual training strategies. The fact that vulnerabilities are more easily exploited in English than French suggests that non-English speaking users could currently benefit from an unintended layer of protection, a disparity that highlights the uneven application of security standards.
<h3 class="wp-block-heading" id="h-looking-forward-strengthening-ai-defenses”>Looking ahead: strengthening ai defenses
Comprehensive evaluation of Kili technology provides a basis for improving the security of the LLM. Their findings suggest that ai developers should prioritize robust security measures across all interaction phases and across languages. Techniques such as adaptive security frameworks, which can dynamically adjust to the nature of prolonged user interactions, may be required to maintain ethical standards without succumbing to gradual degradation.
The Kili technology research team emphasized its plans to expand the scope of its analysis to other languages, including those representing different language families and cultural contexts. This systematic expansion aims to build more resilient ai systems that are capable of protecting users regardless of their linguistic or cultural background.
Collaboration between ai research organizations will be crucial to mitigate these vulnerabilities. Red teaming techniques should become an integral part of ai model evaluation and development, with a focus on creating adaptive, multilingual, and culturally sensitive security mechanisms. By systematically addressing the gaps discovered in Kili's research, ai developers can work to create models that are not only powerful but also ethical and trustworthy.
Conclusion
Kili technology's recent report provides a comprehensive view of current vulnerabilities in ai language models. Despite advances in the security of the models, the findings reveal that significant weaknesses remain, particularly in their susceptibility to misinformation and coercion, as well as inconsistent performance in different languages. As LLMs become increasingly integrated into various aspects of society, ensuring their safety and ethical alignment is paramount.
look at the Full report here. All credit for this research goes to the researchers of this project. Also, don't forget to follow us on <a target="_blank" href="https://twitter.com/Marktechpost”>twitter and join our Telegram channel and LinkedIn Grabove. If you like our work, you will love our information sheet.. Don't forget to join our SubReddit over 55,000ml.
Thanks to Kili technology for the educational/thought leadership article. Kili technology has supported us in this content/article.
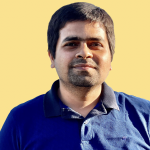
Asif Razzaq is the CEO of Marktechpost Media Inc.. As a visionary entrepreneur and engineer, Asif is committed to harnessing the potential of artificial intelligence for social good. Their most recent endeavor is the launch of an ai media platform, Marktechpost, which stands out for its in-depth coverage of machine learning and deep learning news that is technically sound and easily understandable to a wide audience. The platform has more than 2 million monthly visits, which illustrates its popularity among the public.
<script async src="//platform.twitter.com/widgets.js” charset=”utf-8″>