Humans possess innately extraordinary perceptual judgments, and when computer vision models are aligned with them, model performance can be greatly improved. Various attributes such as scene layout, subject location, camera pose, color, perspective, and semantics help us get a clear picture of the world and the objects in it. Aligning vision models with visual perception makes them sensitive to these attributes and more human-like. While it has been established that shaping vision models along the lines of human perception helps achieve specific goals in certain contexts, such as image generation, its impact on general-purpose roles has yet to be determined. The inferences drawn from the research so far are tinged with a naive incorporation of human perceptual capabilities, seriously damaging the models and distorting the representations. It is also discussed whether the model really matters or whether the results depend on the objective function and the training data. Additionally, the sensitivity and implications of labels further complicate the puzzle. All of these factors further complicate the understanding of human perceptual capabilities with respect to visual tasks.
Researchers from MIT and UC Berkeley analyze this question in depth. Their article “When does perceptual alignment benefit vision representations?” investigates how an aligned model of human vision perception performs on various downstream visual tasks. The authors fine-tuned state-of-the-art ViT models on human similarity judgments for image triplets and evaluated them on standard vision benchmarks. They introduce the idea of a second pre-training stage, which aligns the feature representations of large vision models with human judgments before applying them to subsequent tasks.
To understand this better, we first analyze the image triplets mentioned above. The authors used the renowned NIGHTS synthetic dataset with image triplets annotated with forced-choice human similarity judgments where humans chose two images with the highest similarity to the first image. They formulate a patch alignment objective function to capture spatial representations present in patch tokens and translate visual attributes of global annotations; Instead of calculating the loss only between Vision Transformer's global CLS tokens, they focused CLS and clustered ViT patch embeddings for this purpose to optimize the local patch features along with the global image label. After this, several next-generation Vision transformer models, such as DINO, CLIP, etc., were fine-tuned with the above data using Low Range Adaptation (LoRA). The authors also incorporated synthetic images into triplets with SynCLR to calculate the performance delta.
These models performed better on vision tasks than basic Vision Transformers. For the dense prediction tasks, the human-aligned models outperformed the base models in more than 75% of the cases, for both semantic segmentation and depth estimation. Moving into the realm of generative vision and LLMs, the augmented generation-retrieval tasks were tested by humanizing a vision language model. The results again favored cues recovered by human-aligned models, as they increased classification accuracy across all domains. Furthermore, on the object counting task, these modified models outperformed the baseline in more than 95% of the cases. A similar trend persists in instance recovery. These models failed in the classification tasks due to their high level of semantic understanding.
The authors also addressed whether the training data played a more important role than the training method. For this purpose, more data sets with image triplets were considered. The results were surprising: the NIGHTS data set had the most significant impact and the rest were barely affected. The perceptual signals captured in NIGHTS play a crucial role in this with features such as style, pose, color and object count. Others failed due to an inability to capture the required mid-level perceptual features.
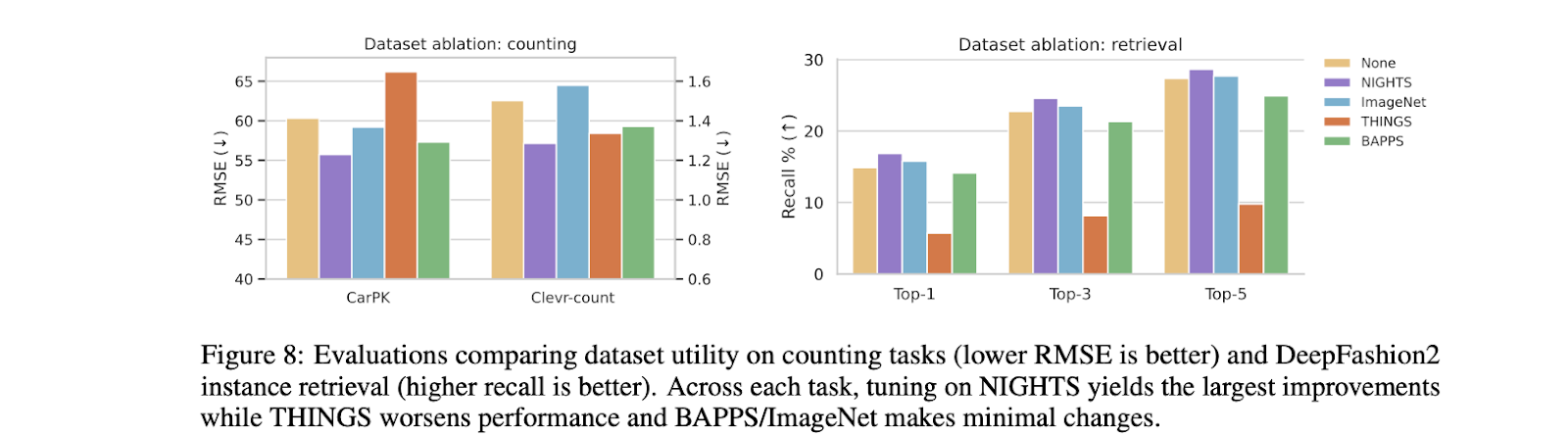
Overall, the human-aligned vision models performed well in most cases. However, these models are prone to overfitting and bias propagation. Therefore, by ensuring the quality and diversity of human annotations, visual intelligence could go one step further.
look at the Paper, GitHuband Project. All credit for this research goes to the researchers of this project. Also, don't forget to follow us on twitter.com/Marktechpost”>twitter and join our Telegram channel and LinkedIn Grabove. If you like our work, you will love our information sheet.. Don't forget to join our SubReddit over 50,000ml.
(Next live webinar: October 29, 2024) Best platform to deliver optimized models: Predibase inference engine (promoted)
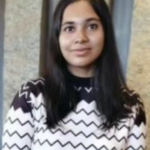
Adeeba Alam Ansari is currently pursuing her dual degree from the Indian Institute of technology (IIT) Kharagpur, where she earned a bachelor's degree in Industrial Engineering and a master's degree in Financial Engineering. With a keen interest in machine learning and artificial intelligence, she is an avid reader and curious person. Adeeba firmly believes in the power of technology to empower society and promote well-being through innovative solutions driven by empathy and a deep understanding of real-world challenges.
<script async src="//platform.twitter.com/widgets.js” charset=”utf-8″>