Liquid ai has launched its ai/liquid-foundation-models”>First series of liquid base models (LFM)ushering in a new generation of generative ai models. These models are positioned as a new benchmark for performance and efficiency at multiple scales, i.e. 1B, 3B and 40B parameter settings. This series aims to set a new standard for generative ai models by achieving cutting-edge performance across multiple benchmarks while maintaining a smaller memory footprint and more efficient inference capabilities.
The first series of LFM comprises three main models:
- LFM-1B: A billion-parameter model that delivers cutting-edge performance for its size class. It has achieved the highest scores in several benchmarks in its class, outperforming many transformer-based models despite not being built on the widely used GPT architecture.
- LFM-3B: A 3 billion parameter model ideal for mobile and edge applications. It not only outperforms its direct competitors in terms of efficiency and speed, but also positions itself as a worthy contender against models in higher parameter ranges, such as the 7B and 13B models of previous generations.
- LFM-40B: A 40 billion parameter combination of experts (MoE) model designed for more complex tasks. This model balances its performance and output quality with even larger models due to its advanced architecture, which allows selective activation of model segments depending on the task, thus optimizing computational efficiency.
Architectural innovations and design principles
LFMs are built from first principles and focus on designing powerful ai systems that offer robust control over their capabilities. According to Liquid ai, these models are built using computational units deeply rooted in dynamical systems, signal processing, and numerical linear algebra theories. This unique combination allows LFMs to leverage theoretical advances in these fields to create general-purpose ai models capable of handling sequential data types, such as video, audio, text, and time series.
The design of LFMs emphasizes two main aspects: characterization and footprint. Characterization consists of converting input data into a structured set of features or vectors used to adaptively modulate the calculation within the model. For example, audio and time series data generally require less characterization by operators due to lower information density compared to language and multimodal data.
The LFM stack is being optimized for deployment on several hardware platforms, including NVIDIA, AMD, Qualcomm, Cerebras, and Apple. This optimization enables performance improvements in different deployment environments, from edge devices to large-scale cloud infrastructures.
Comparison and performance benchmarks
Initial testing of LFMs shows impressive results compared to similar models. Model 1B, for example, outperformed several transformer-based models in terms of multimodal learning and understanding (MMLU) scores and other benchmark metrics. Similarly, the performance of model 3B has been compared to models in categories 7B and 13B, making it well suited for resource-limited environments.
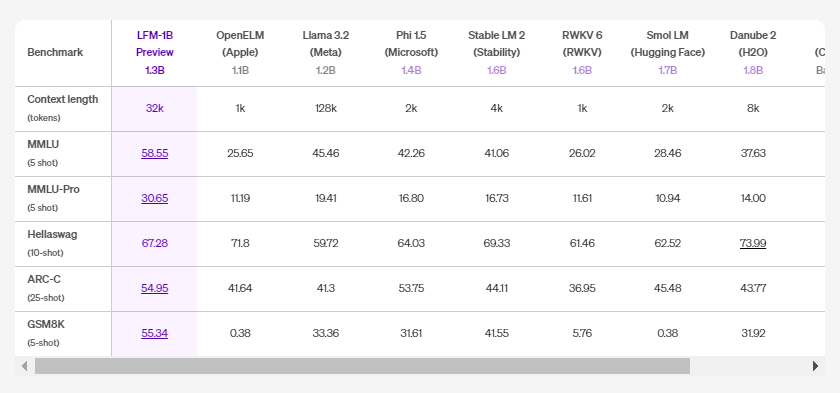
The 40B MoE model, on the other hand, offers a new balance between model size and output quality. This model's architecture leverages a unique combination of experts to enable higher performance and deployment on cost-effective hardware. It achieves performance comparable to larger models due to its efficient utilization of the MoE architecture.
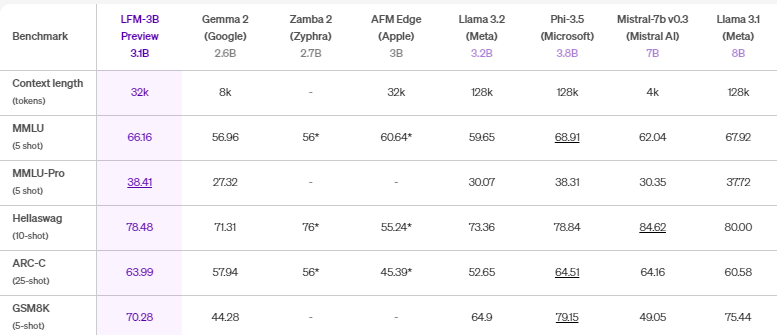
Key strengths and use cases
Liquid ai has highlighted several areas in which LFMs demonstrate significant strengths, including general and expert knowledge, mathematics and logical reasoning, and efficient long-context tasks. The models also offer strong multilingual capabilities and support Spanish, French, German, Chinese, Arabic, Japanese and Korean languages. However, LFMs are less effective in zero-shot code tasks and precise numerical calculations. This gap is expected to be addressed in future iterations of the models.
LFMs have also been optimized to handle longer context lengths more effectively than traditional transformer models. For example, the models can process up to 32,000 tokens in context, making them particularly effective for document analysis and summary tasks, more meaningful interactions with context-aware chatbots, and improved retrieval augmented generation (RAG) performance.
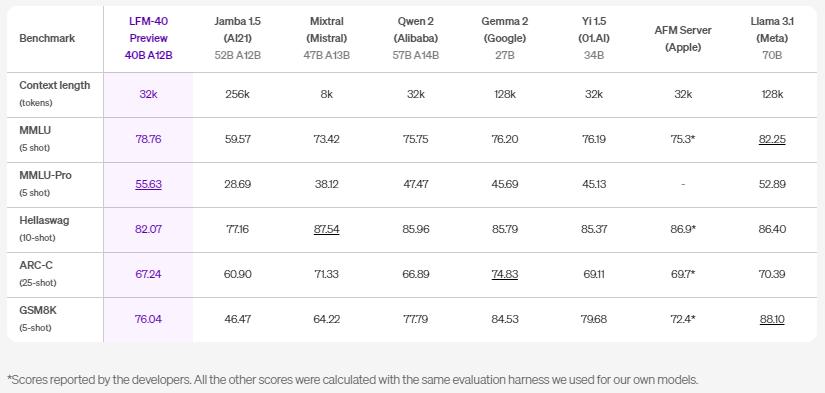
Implementation and future directions
Liquid ai LFMs are currently available for testing and deployment on several platforms, including Liquid Playground, Lambda (Chat UI and API), Perplexity Labs, and soon on Cerebras Inference. Liquid ai's roadmap suggests that it will continue to optimize and launch new capabilities in the coming months, expanding the reach and applicability of LFMs to various industries, such as financial services, biotechnology, and consumer electronics.
In terms of deployment strategy, LFMs are designed to be adaptable across multiple modalities and hardware requirements. This adaptability is achieved through adaptive linear operators that are structured to respond dynamically based on inputs. This flexibility is critical for deploying these models in environments ranging from high-end cloud servers to more resource-constrained edge devices.
Conclusion
Liquid ai's first series of Liquid Foundation Models (LFMs) represent a promising step forward in the development of generative ai models. LFMs aim to redefine what is possible in the design and implementation of ai models by achieving superior performance and efficiency. While these models are not open source and are only available as part of a controlled release, their unique architecture and innovative approach position them as major contenders in the ai landscape.
look at the ai/liquid-foundation-models” target=”_blank” rel=”noreferrer noopener”>Details. All credit for this research goes to the researchers of this project. Also, don't forget to follow us on twitter.com/Marktechpost”>twitter and join our Telegram channel and LinkedIn Grabove. If you like our work, you will love our information sheet..
Don't forget to join our SubReddit over 50,000ml
Want to get in front of over 1 million ai readers? ai-newsletter-alignment-lab-ai-releases-buzz-dataset-snowflake-introduces-arctic-embed-openai-released-gpt-4o-and-many-more” target=”_blank” rel=”noreferrer noopener”>Work with us here
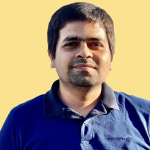
Asif Razzaq is the CEO of Marktechpost Media Inc.. As a visionary entrepreneur and engineer, Asif is committed to harnessing the potential of artificial intelligence for social good. Their most recent endeavor is the launch of an ai media platform, Marktechpost, which stands out for its in-depth coverage of machine learning and deep learning news that is technically sound and easily understandable to a wide audience. The platform has more than 2 million monthly visits, which illustrates its popularity among the public.
<script async src="//platform.twitter.com/widgets.js” charset=”utf-8″>