Deep learning has made significant advances in artificial intelligence, particularly in natural language processing and computer vision. However, even the most advanced systems often fail in ways that humans would not, highlighting a critical gap between artificial and human intelligence. This discrepancy has reignited debates about whether neural networks possess the essential components of human cognition. The challenge lies in developing systems that exhibit more human-like behavior, particularly with regard to robustness and generalization. Unlike humans, who can adapt to environmental changes and generalize across diverse visual environments, ai models often need help with changing data distributions between training and test sets. This lack of robustness in visual representations poses significant challenges for downstream applications that require strong generalization capabilities.
Researchers from Google DeepMind, Machine Learning Group, Technische Universität Berlin, BIFOLD, Berlin Institute for the Foundations of Learning and Data, Max Planck Institute for Human Development, Anthropic, Department of artificial intelligence, Korea University, Seoul, Max Planck Institute for Informatics propose a unique framework called Alignment to address the misalignment between human and machine visual representations. This approach aims to simulate large-scale human-like similarity judgment datasets to align neural network models with human perception. The methodology begins by using an affine transformation to align model representations with human semantic judgments in odd-one-out triplet tasks. This process incorporates uncertainty measures of human responses to improve model calibration. The aligned version of a state-of-the-art vision base model (VFM) then serves as a surrogate to generate human-like similarity judgments. By clustering representations into meaningful superordinate categories, the researchers sample semantically meaningful triplets and obtain odd-numbered responses from the surrogate model, resulting in a comprehensive dataset of human-like triplet judgments called AligNet.
The results demonstrate significant improvements in aligning machine representations with human judgments at multiple levels of abstraction. For global coarse-grained semantics, soft alignment substantially improved model performance, with accuracies increasing from 36.09–57.38% to 65.70–68.56%, surpassing the human-to-human reliability score of 61.92%. For local fine-grained semantics, alignment improved moderately, with accuracies increasing from 46.04–57.72% to 58.93–62.92%. For class boundary triplets, AligNet fine-tuning achieved remarkable alignment, with accuracies reaching 93.09–94.24%, surpassing the human noise ceiling of 89.21%. Alignment effectiveness varied across abstraction levels, with different models showing strengths in different areas. In particular, AligNet's fine-tuning generalized well to other human similarity judgment datasets, demonstrating substantial alignment improvements on several object similarity tasks, including Likert-scale and multi-array pairwise similarity ratings.
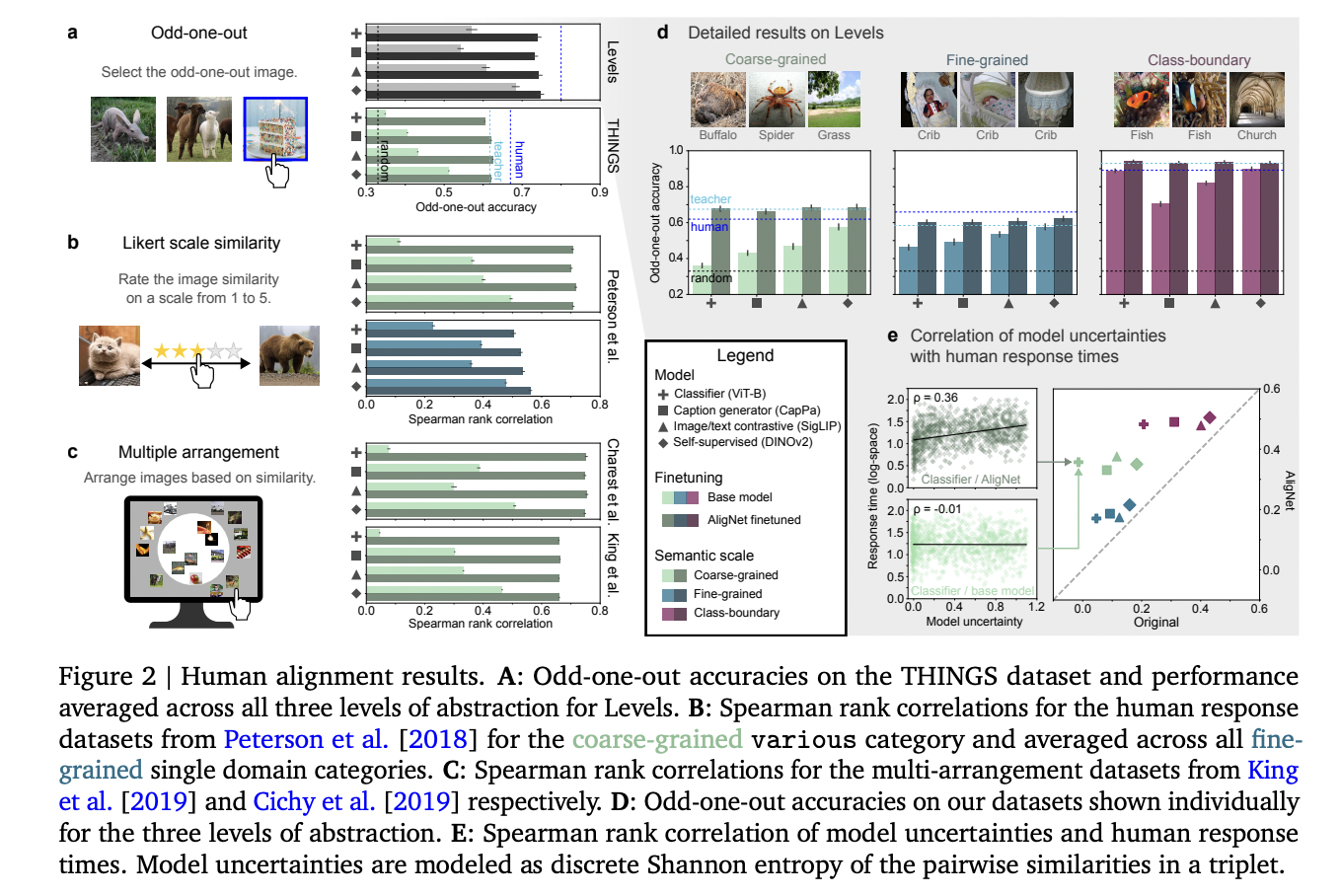
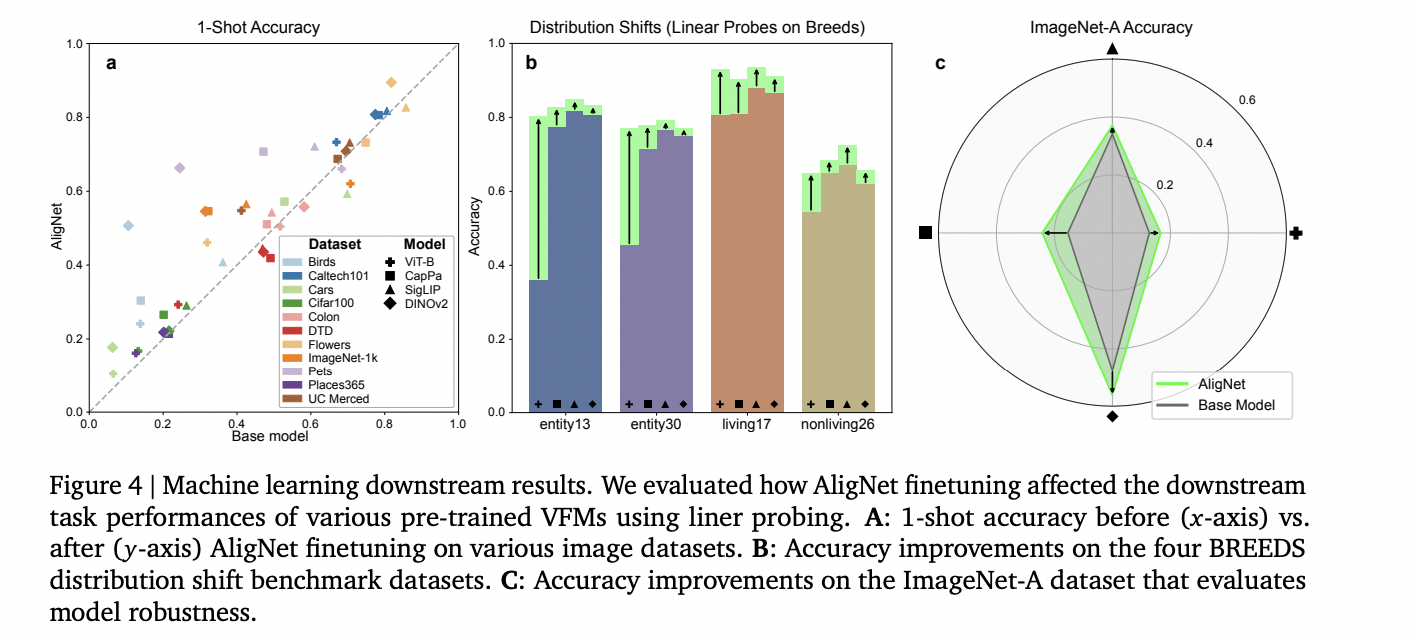
The AligNet methodology comprises several key steps to align machine representations with human visual perception. It initially uses the THINGS odd-triplet dataset to learn an affine transformation on a global human object similarity space. This transformation is then applied to representations from a teacher model, creating a similarity matrix for pairs of objects. The process incorporates measures of uncertainty about human responses using an approximate Bayesian inference method, replacing hard alignment with soft alignment.
The objective function of learning the uncertainty distillation transformation is to combine soft alignment with regularization to preserve local similarity structure. The transformed representations are then clustered into superordinate categories using k-means clustering. These clusters guide the generation of triplets from distinct ImageNet images, with choices of outliers determined by the surrogate master model.
Finally, a robust objective function based on Kullback-Leibler divergence facilitates the distillation of the teacher’s pairwise similarity structure into a network of students. This objective of AligNet is combined with regularization to preserve the pre-trained representation space, resulting in a fine-tuned student model that better aligns with human visual representations at multiple levels of abstraction.
This study addresses a critical shortcoming in baseline models of vision: their inability to adequately represent the multi-level conceptual structure of human semantic knowledge. By developing the AligNet framework, which aligns deep learning models with human similarity judgments, the research demonstrates significant improvements in model performance on several cognitive and machine learning tasks. The findings contribute to the ongoing debate about the ability of neural networks to capture human-like intelligence, particularly in relational understanding and hierarchical knowledge organization. Ultimately, this work illustrates how representational alignment can improve model generalization and robustness, bridging the gap between machine and human visual perception.
Take a look at the PaperAll credit for this research goes to the researchers of this project. Also, don't forget to follow us on twitter.com/Marktechpost”>twitter and join our Telegram Channel and LinkedIn GrAbove!. If you like our work, you will love our fact sheet..
Don't forget to join our SubReddit of over 50,000 ml
FREE ai WEBINAR: 'SAM 2 for Video: How to Optimize Your Data' (Wednesday, September 25, 4:00 am – 4:45 am EST)
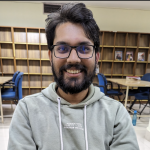
Asjad is a consultant intern at Marktechpost. He is pursuing Bachelors in Mechanical Engineering from Indian Institute of technology, Kharagpur. Asjad is a Machine Learning and Deep Learning enthusiast who is always researching the applications of Machine Learning in the healthcare domain.
<script async src="//platform.twitter.com/widgets.js” charset=”utf-8″>